Create photorealistic images of your products in any environment without expensive photo shoots! (Get started for free)
How AI Image Generation Captures Nari Ward's 'Iron Heaven' Texture and Material Aesthetics in Product Photography
How AI Image Generation Captures Nari Ward's 'Iron Heaven' Texture and Material Aesthetics in Product Photography - Material Recognition AI Studies Iron Textures From 1970s Found Art
Artificial intelligence dedicated to recognizing materials is now examining the distinctive textures of iron used in 1970s found art. This exploration reveals that understanding artistic materials goes beyond simply recognizing colors and shapes. While AI can pinpoint areas in an image with the same material with considerable accuracy, the process highlights a crucial aspect: relying on local details like color and texture isn't enough for comprehensive material identification. This research shows how AI is changing the way we understand art, providing a deeper look into the varied and subtle visual aspects of different materials, especially when considering product photography. As AI becomes more sophisticated, it's altering our perception and categorization of artistic textures, forcing us to reconsider ideas about authenticity and representation in a world increasingly dominated by digital images. The use of AI in art doesn't just improve record-keeping; it challenges how we interact with art in its historical context.
Examining iron textures from 1970s found art has provided a unique lens for understanding how AI can analyze and reproduce material aesthetics, especially relevant in generating product images for e-commerce. Early attempts at material recognition, even with basic features like color and shape, were insufficient, pushing AI researchers towards more sophisticated approaches. We've seen that AI can, with a relatively high level of accuracy, identify areas within an image that share a common material. This capability is becoming increasingly important in areas like product photography, where the correct visual representation of materials is key to consumer appeal.
The development of convolutional neural networks has been a game-changer in texture analysis, going beyond older methods like "bag of words" to achieve much more detailed understandings. However, even with advancements in deep learning, reliably recognizing materials from just a color image remains a challenge. AI models excel at identifying objects, but tailoring them for materials requires specialized adaptation. The way we represent materials within images is now a central focus, and through that, impressive results are being achieved.
The implications for the arts are also significant. AI's role has changed from a rigid, primarily engineering-driven approach to a more interactive and nuanced understanding of historical artworks and the materials they used. The prospect of a vast digital library of artworks, filled with ultra-high resolution images, is on the horizon. This, in turn, will support a future where these images can be computationally analyzed to study the intricacies of art materials and techniques, bringing fresh insights into artistic practices. As AI develops, we also see more refined computer vision methods which can give us deeper knowledge of textures and other fine art elements. While the potential of AI in art is exciting, we should be careful about evaluating its outputs. Even with impressive results, sometimes there's a lack of material authenticity in the generated images. Further algorithm refinement is needed to achieve truly accurate representations of materials in commercial settings.
How AI Image Generation Captures Nari Ward's 'Iron Heaven' Texture and Material Aesthetics in Product Photography - AI Product Photography Builds On Ward's Visual Language and Metal Contrast
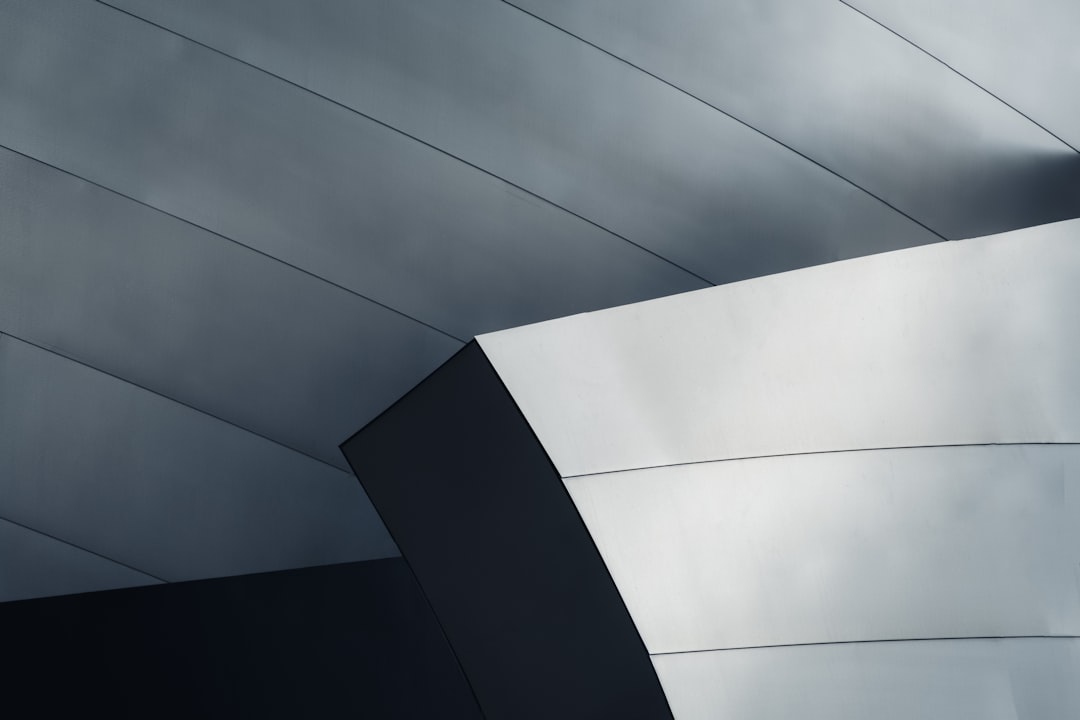
AI in product photography is increasingly drawing inspiration from artistic styles, particularly those focused on material contrast like Nari Ward's work. This involves using AI to replicate the unique visual language of artists, especially the way they explore textures like metal surfaces. AI-powered tools can now analyze and generate product images that reflect the depth and richness of these textures, capturing the essence of an artist's vision in an e-commerce context. This creates a more captivating product presentation, helping brands establish a stronger, more unified visual identity across their listings. While this technology shows promise in replicating material aesthetics, the challenge lies in ensuring that the generated images maintain a high degree of authenticity. The quest for visually compelling product imagery using AI, while exciting, also raises questions about the relationship between the digital and the real, particularly in a commercial setting.
Building on the insights gained from analyzing Ward's work, AI in product photography now aims to capture the essence of material contrasts, particularly in metal. It's becoming evident that simply replicating textures like rusted iron or polished metal isn't enough. The goal is to convey the subtleties of materiality, much like how Ward uses found objects to evoke a sense of place and time.
AI-powered product image generators are leveraging complex algorithms that can analyze vast datasets of images, helping them learn and reproduce intricate textures and finishes. The more sophisticated these algorithms become, the closer they come to capturing a particular aesthetic. While it's impressive that they can recognize areas of shared material within a photograph, they face challenges in consistently representing the visual nuances that make a material unique. We are still early in this development, and many AI models are still best used for simpler tasks.
There's a growing interest in how we represent materials in digital images. This is where the real power of AI seems to lie, as these representations can influence how we perceive and categorize products. Imagine a digital library of various materials, each with a specific 'signature' that AI can interpret. This could potentially provide a much more precise understanding of materials, both for creative design and for accurate representation in e-commerce.
However, a note of caution is warranted. While AI can create remarkably realistic images, we must remain vigilant about evaluating their authenticity. Is a digitally generated image of a rusty object truly capturing the material's essence, or is it merely a superficial imitation? We see this in how AI can get color and contrast correct but might struggle with the detailed textures of aged materials. This presents an interesting problem for the future of AI product photography as we try to develop more accurate representations of materials, and we see a clear need for improved AI models to achieve truly accurate renderings of nuanced material properties.
How AI Image Generation Captures Nari Ward's 'Iron Heaven' Texture and Material Aesthetics in Product Photography - Neural Networks Map Surface Composition Through Advanced Texture Analysis
AI's capacity to understand and replicate material textures is improving rapidly through neural networks. These networks, particularly convolutional neural networks, are adept at recognizing complex textural patterns, a skill crucial for creating realistic product imagery. This ability to analyze and interpret texture details is fundamental in bridging the gap between the artistic vision and the needs of e-commerce. We see that AI can generate product images that reflect the subtle nuances of materials, which enhances the visual appeal and helps brands present a unified aesthetic across their product listings. As these networks advance, they are capable of refining the standards for how we present products visually, but this does create a tension. While we see exciting progress in the ability of AI to "see" materials in images, there's an important need to consider how well generated images authentically portray real-world materials. The ability to accurately represent textures and materials remains a challenge for AI, and if we're not careful, the pursuit of digital perfection may overshadow the importance of genuine material representation.
Neural networks are increasingly used to understand surface textures, which is vital for product photography where small details influence how shoppers perceive an item. These networks use layered structures to recognize textures at varying levels, from broad patterns to the finer details. This multi-level approach gives AI the ability to pick up on nuances that would otherwise be missed.
Convolutional neural networks (CNNs) have made it possible for AI to be extremely sensitive to textures. They can capture both the large-scale and the microscopic features of a surface. In product photos, this allows the AI to capture aspects like subtle imperfections, which can communicate the authenticity of a material. However, accurately capturing the subtleties that make materials unique requires large and diverse datasets for AI to learn from. The AI models must be trained on a wide range of high-quality images with different lighting, materials, and even small imperfections. This provides them with a wider spectrum of examples to learn from, leading to more accurate results when generating or analyzing product images.
We've seen recently that AI models, particularly generative adversarial networks (GANs), can produce incredibly realistic images. They're capable of creating images with textures that mimic real-world materials. This ability to generate hyper-realistic product images holds great potential for e-commerce. However, the visual appeal must be balanced with authenticity.
AI researchers are utilizing methods like t-SNE (t-distributed Stochastic Neighbor Embedding) to help visualize the intricate data produced by neural networks. This helps researchers better understand the patterns within textures, ultimately aiding in creating better image representations.
We can even use AI to capture the “moments” of textures – in essence, quantifiable properties of a texture. This has implications for marketers as they could pinpoint and describe those moments in a way that better communicates a product's features, particularly related to the feel of a product.
Another interesting point is that the quality of light can change how we perceive a texture. AI systems are learning how to adjust for differences in lighting in the source images, so we can create a more consistent presentation across different product shots.
Some AI architectures have a kind of "memory" of learned textures. They can retain this memory over time. This potentially helps maintain a consistent visual identity for a brand. However, this consistency shouldn't come at the expense of authenticity.
A key problem in this area is determining if a texture is real or AI-generated. We need rigorous testing, comparing generated images with actual photos of materials. This ensures that we can evaluate the accuracy of the AI's representations.
Ultimately, creating authentic-looking product images requires the AI to focus on the finer details, those minute imperfections and irregularities that often tell us the most about a material. These small characteristics are crucial for communicating authenticity and that’s where a lot of AI research is currently focused.
How AI Image Generation Captures Nari Ward's 'Iron Heaven' Texture and Material Aesthetics in Product Photography - Generative AI Adapts Industrial Materials Into Ecommerce Product Staging
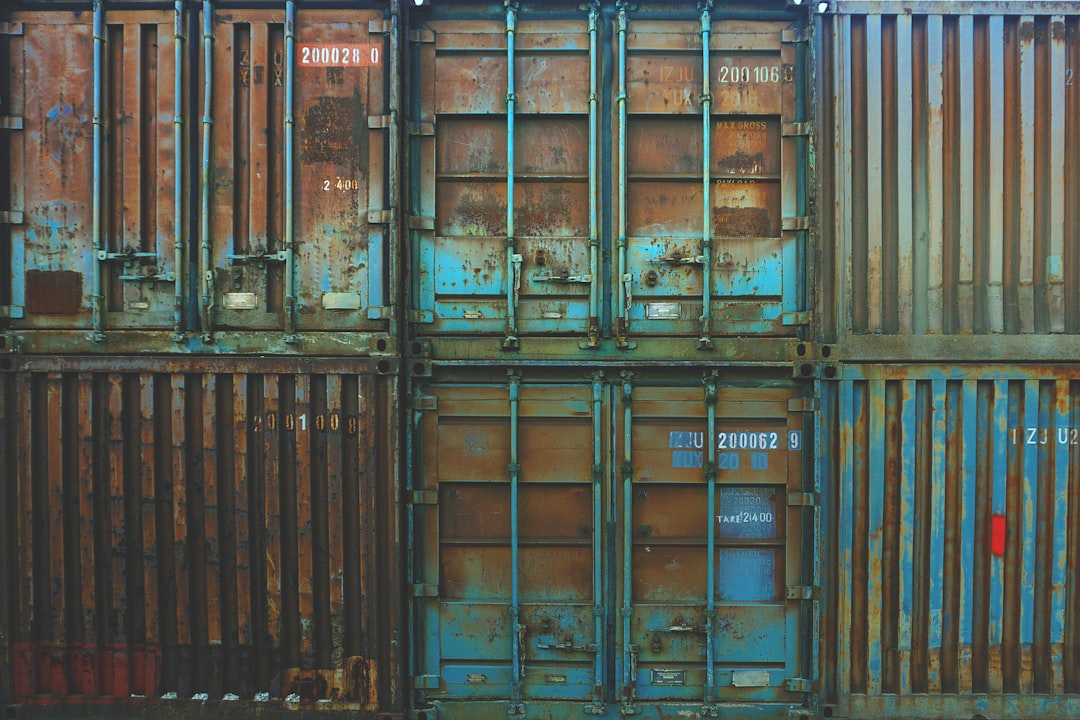
Generative AI is increasingly used to create more engaging product images for e-commerce by cleverly adapting industrial materials into visually appealing digital scenes. This technology lets us produce dynamic images that showcase the subtle textures and finishes of various products, connecting visual appeal with practical sales goals. Using advanced algorithms, generative AI can capture the complexities of materials, not only making them more visually engaging but also influencing how shoppers understand the product's authenticity. As companies strive for stronger brand identities, it becomes crucial that the generated images don't lose sight of the actual material's properties in pursuit of purely visual appeal. The ongoing effort to improve AI methods is key to striking a balance between drawing inspiration from artistic methods and meeting the practical demands of e-commerce product photography. There's a constant tension between creating something eye-catching and something genuinely representative.
AI's ability to recognize textures is having a notable impact on how we buy things online. Studies suggest that people prefer to buy products with well-represented textures, as it gives them a stronger sense of trust in the item's quality and authenticity. This has a tangible impact on e-commerce businesses.
AI systems are becoming more sophisticated in how they analyze textures. They can now cross-check a product image against a massive library of materials to confirm the texture's accuracy against its real-world counterpart. This is helpful for shoppers who want to be more certain of what they're getting.
Generative adversarial networks (GANs) are getting really good at making photorealistic images by essentially pitting two neural networks against each other. This competition allows them to produce very detailed textures that can imitate real-world materials in ways that make products look even more appealing to online shoppers.
There's a growing trend in AI-powered product staging to capture subtle imperfections like small scratches or color variations. These seemingly minor details help shoppers feel confident that a product isn't just some perfect, unreal object. This can help reduce returns.
Training AI models to generate great product images requires feeding them huge datasets filled with photos under different lighting conditions and showing different kinds of materials. These diverse datasets help the AI learn the complex interplay between light and texture, ultimately improving the quality of images it generates.
AI is adopting multi-layered techniques to study textures at many different scales. It can pick up both large-scale patterns and tiny details, which is critical for products where the feel or appearance of the material matters to consumers, like leather or fabrics.
AI systems are getting better at adapting to inconsistencies in the lighting used during product shoots, ensuring that products are presented with a uniform look. This consistency contributes to building a strong, recognizable visual identity across an e-commerce brand's online platform.
The idea of having a digital library of materials, each with a kind of 'unique fingerprint' that AI can read, is quite exciting. With such a database, AI could generate images that better represent the materials needed for a product, likely leading to increased user interest and satisfaction.
A big ongoing issue is the ability to tell the difference between authentic product images and those generated by AI. Researchers are working on creating rigorous methods to check the authenticity of textures and ensure shoppers see only realistic representations.
Interestingly, AI is beginning to capture the 'essence' of a texture, by identifying quantifiable qualities. This could be huge for marketers as they try to use language that more precisely conveys the essential features of a product, specifically when it comes to tactile qualities.
How AI Image Generation Captures Nari Ward's 'Iron Heaven' Texture and Material Aesthetics in Product Photography - Machine Learning Translates Physical Artwork Properties To Digital Images
Machine learning is increasingly adept at translating the physical characteristics of artwork into digital images, especially within the realm of ecommerce product photography. AI systems, utilizing methods like generative adversarial networks (GANs) and convolutional neural networks (CNNs), can now decipher and replicate the intricacies of materials, resulting in more detailed and accurate image generation. This capability is showcased in efforts to reproduce the unique textures present in works like Nari Ward's 'Iron Heaven,' enhancing the overall authenticity of product presentations online. Despite this progress, it's crucial to question whether AI-generated images truly encapsulate the complex nature of real-world materials or merely offer a surface-level imitation. The challenge of maintaining a balance between visual appeal and material authenticity is a central concern as the use of AI in e-commerce product staging expands.
AI's ability to understand and recreate the look and feel of materials is rapidly evolving, especially within e-commerce product photography. Researchers are finding ways to measure texture qualities, moving from just seeing color and shape to actually quantifying how a material feels or might wear. This ability to describe materials numerically could change how we market products, providing more precise details about their textures.
AI is becoming increasingly aware of lighting conditions when evaluating product images. Algorithms are learning to account for variations in light to create more consistent product images, which is crucial for online shopping where a product might be seen in many different contexts. Consistent imagery builds brand identities and helps shoppers feel confident about what they're ordering.
Building off massive libraries of images of different materials, AI is able to assign a sort of 'signature' to each material based on its texture and finish. This could mean AI will eventually be much better at assuring shoppers that what they see online reflects the actual material they’ll receive. It's all about matching digital representations with shoppers' expectations.
Studies show consumers place more trust in products with detailed textures, suggesting a strong link between the quality of product photos and sales. There's a powerful link between how well materials are rendered and how much confidence customers have in a product. This reinforces the need for ever more realistic image generation.
Researchers are working on ways to detect if a texture has been created by AI or if it’s a photo of a real object. This is important for maintaining trust in online retail and could become an important standard to ensure that shoppers are always presented with truthful and reliable product imagery.
AI is now capable of analyzing textures in real-time, changing the way generated product images adapt to how consumers interact with them. Imagine a product image changing based on how you hover over or zoom in on the product, providing immediate visual feedback to shopper interests. This ability to react to viewer preferences in real-time enhances the shopping experience.
It’s been found that adding minor imperfections to product images, such as tiny scratches or color variations, builds consumer trust. AI image generators are being trained to incorporate these nuances, enhancing their ability to capture materials authentically. This adds a level of real-world detail to the rendered image, creating a more compelling presentation.
Generative adversarial networks (GANs), are proving to be very adept at generating hyper-realistic textures. The way GANs are designed, having two competing networks, leads to constant improvement and generation of images that are incredibly difficult to distinguish from the real thing. This has implications for brands that want to showcase highly detailed textures in their product presentations.
AI is developing a multi-layered ability to see textures – identifying large-scale patterns and very small details within a surface. This detailed, multifaceted understanding is particularly critical for items where the material’s feel is important, such as fabrics or leather.
The idea of creating an extensive digital catalog of materials, complete with their unique 'fingerprints' is gaining popularity. This digital archive could provide a powerful resource for AI, enabling it to select and recreate materials with greater accuracy, further enhancing the authenticity and realism of generated product images.
How AI Image Generation Captures Nari Ward's 'Iron Heaven' Texture and Material Aesthetics in Product Photography - Digital Light Modeling Replicates Gallery Installation Photography
Within the realm of e-commerce product photography, digital light modeling is a developing technique that promises to replicate the complex aesthetics of art gallery installations. This approach uses sophisticated AI algorithms to imitate lighting and texture effects, mimicking the intricacies found in art-rich settings. The ability to recreate these intricate lighting patterns greatly improves the quality of e-commerce images, helping brands showcase their products in a way that fosters a deeper connection with shoppers. However, it's crucial to ensure that these AI-generated visuals don't simply offer a superficial impression, but accurately portray the material attributes that consumers anticipate in a physical product. The growing ambiguity between the digital and real in online shopping brings into sharper focus the need for a thorough assessment of AI outputs to ensure that online shopping experiences are built on a foundation of trust.
AI's growing ability to understand and replicate material textures is significantly impacting product photography, particularly within e-commerce. Convolutional neural networks are becoming increasingly adept at analyzing textures at multiple levels, from broad patterns to microscopic details. This multi-scale approach is crucial for generating realistic product images that capture the nuances of different materials. Simultaneously, Generative Adversarial Networks (GANs) have proven remarkably successful at producing photorealistic textures. By pitting two neural networks against each other in a competitive learning process, GANs can create images that mimic the appearance of real-world materials with a high degree of fidelity.
Interestingly, research suggests that detailed and accurate texture representation plays a significant role in consumer trust. Shoppers tend to be more confident in making purchases when they feel they have a reliable visual representation of a product's material. This is a powerful insight for e-commerce, as accurately conveying material qualities can directly impact sales. To improve visual consistency across platforms, AI systems are developing sophisticated ways to adapt to various lighting conditions in product images. This capability is essential for maintaining a strong brand identity. The future of this technology might involve creating a 'digital library' of materials, each with a unique 'fingerprint' that AI can interpret. This could allow for a higher degree of accuracy in matching digital product representations to their real-world counterparts.
In another intriguing development, studies have shown that adding subtle imperfections to AI-generated images can actually increase consumer trust. The presence of minor flaws like scratches or slight color variations can make products appear more genuine and less like artificial renderings. This can have a positive impact on reducing returns, a major concern for online businesses. Furthermore, AI is moving beyond visual recognition to quantify texture qualities. This allows for more nuanced descriptions of materials, which could change marketing approaches by providing shoppers with detailed and precise information about tactile properties. This aligns with the desire for consumers to know exactly what they are purchasing, promoting greater transparency and satisfaction.
There is also growing interest in developing AI systems that can analyze textures in real-time. This allows for dynamic product representations that adapt to user interactions, creating a more personalized and interactive online shopping experience. In tandem, some AI architectures are refining their ability to understand textures at multiple levels, capturing both the broad patterns and the finest details of a surface. This ability to discern texture nuances is particularly important for materials like fabrics or metals, where the tactile experience is central to a shopper's decision-making. As we rely more on AI for generating product images, there's a parallel need for researchers to develop methods for detecting whether a texture is AI-generated or photographed. This is essential for maintaining consumer trust in online marketplaces, ensuring that products are presented with honest and accurate visual representations. These efforts to verify authenticity are becoming increasingly important as the sophistication of AI image generation tools progresses.
Create photorealistic images of your products in any environment without expensive photo shoots! (Get started for free)
More Posts from lionvaplus.com: