AI-Generated Product Images Balancing Innovation and Cultural Sensitivity in E-commerce
AI-Generated Product Images Balancing Innovation and Cultural Sensitivity in E-commerce - Training data quality determines AI image generation success
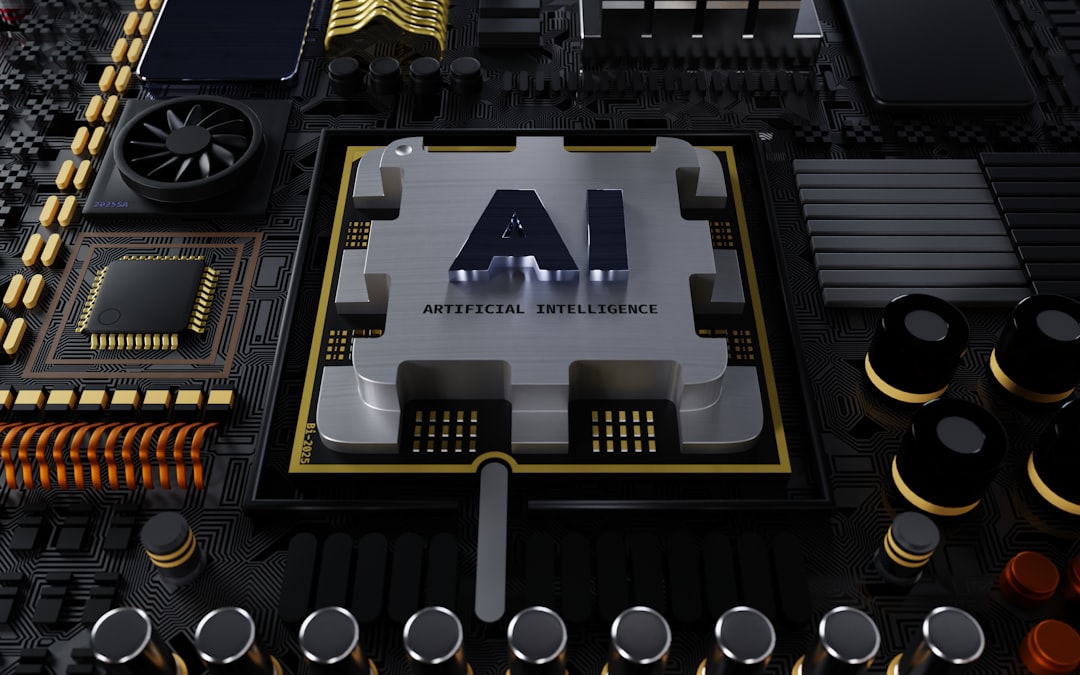
The effectiveness of AI image generation hinges on the quality of the training data. It's not just about any data; it's about data specifically tailored to the task at hand. Imagine teaching a child to draw a car. If you show them only crude sketches, their drawings will reflect that. Similarly, AI systems trained on low-quality or inconsistent data will produce images that fall short.
In e-commerce, where visual appeal is paramount, this becomes a major issue. If the AI is trained on a mixture of real and artificial images, the results can be unpredictable. The "quality collapse" that often occurs in such scenarios highlights the need for dedicated, well-structured training data to ensure a consistent and professional look.
As AI image generation becomes more prevalent, the need for robust quality assessment tools will become increasingly vital. Simply applying traditional image quality metrics won't suffice. AI-generated images introduce their own unique set of distortions, requiring dedicated tools that can accurately gauge their quality and identify potential problems.
The success of AI image generation for e-commerce product images hinges on the quality of the training data. It's not just about the volume of data but also its relevance, diversity, and accuracy. This is especially critical as AI models, like StyleGAN and diffusion models, can exhibit a drop in quality if trained primarily on other AI-generated images. The training data must capture the real world; otherwise, it can lead to inaccurate representations of product textures and materials, which could mislead consumers.
Furthermore, poorly labeled data can introduce biases in AI outputs, potentially harming a brand's reputation and alienating customer segments. This underscores the importance of consistent prompt structures and terminology throughout the training dataset to ensure consistency in AI-generated product images. High-quality training data with diverse representations can enhance the authenticity of AI-generated images, minimizing the risk of generating misleading visuals and improving the accuracy in reflecting the physical attributes of products.
While post-processing techniques can enhance the polish of AI-generated images, the foundation of quality lies in the training data. This is particularly important for e-commerce where accurate and visually appealing product images are crucial for customer engagement and satisfaction. It's fascinating how advancements in AI-powered image generation allow for real-time adjustments based on user interactions. However, these adaptive features are only as effective as the high-quality training data behind them.
There's a growing need for databases that consistently evaluate the quality of AI-generated images, especially as these images become increasingly prevalent in various sectors. The development of comprehensive databases for AI-generated image quality assessment is crucial to address quality variance among different AI generation models. This could lead to the development of new techniques like the Distribution Matching Distillation (DMD) method, which merges principles from GANs and diffusion models to produce high-quality images at a much faster pace. However, traditional deep neural networks used for image quality assessment struggle with AI-generated images compared to their performance on natural scene images. Therefore, developing quality assessment models specifically tailored for AI-generated content is critical.
AI-Generated Product Images Balancing Innovation and Cultural Sensitivity in E-commerce - Crafting effective prompts crucial for AI product image creation
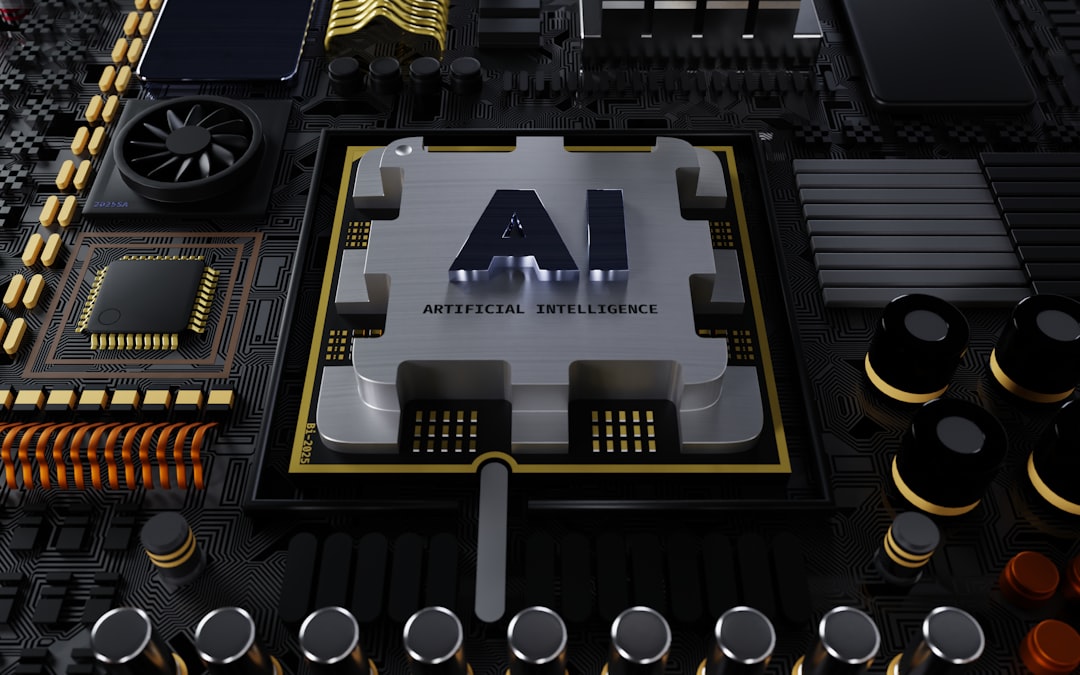
Crafting effective prompts is a crucial skill for generating appealing product images using AI. These prompts are like blueprints that guide the AI, and their quality directly impacts the final result. Balancing clarity and specificity is key, ensuring the AI accurately understands your vision. A poorly constructed prompt can lead to ambiguity and unexpected outcomes. Clear descriptions, capturing the desired aesthetic and context, are vital.
As AI tools become more popular, especially among younger generations, mastering prompt engineering becomes crucial. This skill is essential for achieving high-quality results and meeting consumer expectations. The journey of AI-generated images begins with thoughtful prompts that shape the technology to create visuals that resonate with consumers.
The quality of a prompt is absolutely crucial for creating effective product images with AI. It's not just about throwing a bunch of words at the system and hoping for the best; we need a more deliberate and strategic approach. For example, it's fascinating how even slight variations in the language used to describe a product can lead to drastically different results. A prompt that focuses on specific product features will likely result in images that highlight those features, while a prompt that uses general terms might lead to more generic, less focused images.
There's also a strong argument for incorporating specific style descriptors within prompts. If we want a product image to reflect a specific artistic style, the AI needs to be explicitly told. This suggests that AI systems aren't yet capable of fully understanding and interpreting style cues like humans do. It's like giving an AI artist a specific paintbrush but then expecting them to understand how to use it without explicit instructions. The more information we provide about the desired aesthetic, the better the chances of getting a result that truly matches our vision.
Then there's the issue of color. AI systems often struggle with accurate color representation, especially when they lack information about lighting conditions. This is because human perception of color is complex and influenced by various factors. A prompt that includes details about the lighting scenario can greatly improve the accuracy of color in the generated image, helping to avoid any surprises when a customer receives their product and finds it looks different from what they saw online.
The cultural context is also a fascinating aspect of prompt design. Including cultural references or design principles within prompts can help the AI generate images that are more appealing and relevant to specific consumer groups. This highlights the need for AI systems to become more culturally aware and sensitive, especially as they become more widely used in global markets.
But it's not just about crafting the perfect prompt – it's about an iterative process. As the research suggests, prompt refinement is key to achieving optimal results. It's about experimenting, analyzing the initial output, and making adjustments based on what we see. It's like sculpting, slowly shaping the image to match our vision. This process isn't limited to just the prompt, though. The quality and diversity of the data used to train the AI also play a major role in the final results. If the dataset is narrow, then the AI will be limited in its ability to produce creative and diverse images.
But the research also throws a wrench into the idea that "more is better" when it comes to prompts. Sometimes, shorter and simpler prompts can be more effective than overly detailed ones. This suggests that AI systems might not always be able to handle complex instructions as well as humans do.
The exciting part is that there are ongoing developments like transfer learning that offer promise for tailoring AI to specific tasks. Imagine an AI system that's specifically trained on a vast library of product images, which then can generate remarkably accurate and visually appealing product images for new products. This could greatly reduce the need for massive datasets, leading to faster and more efficient results.
It's a game of optimization. A/B testing is becoming increasingly popular in e-commerce, not just for market strategies but also for prompt refinement. By experimenting with different prompt variations and analyzing how users react to the resulting images, we can fine-tune our prompts to maximize engagement and ultimately drive sales.
And with the evolution of AI, we are now entering a realm where the lines between image creation and interaction are blurring. Some AI systems are becoming adaptive, adjusting images based on user input in real-time. This opens up fascinating possibilities for personalized product experiences where customers can interact with visuals and dynamically create customized versions of the products they are considering. It's a shift from static visuals to dynamic experiences, and it's all powered by the prompts we craft.
AI-Generated Product Images Balancing Innovation and Cultural Sensitivity in E-commerce - Interactive visuals allow customers to preview product variations
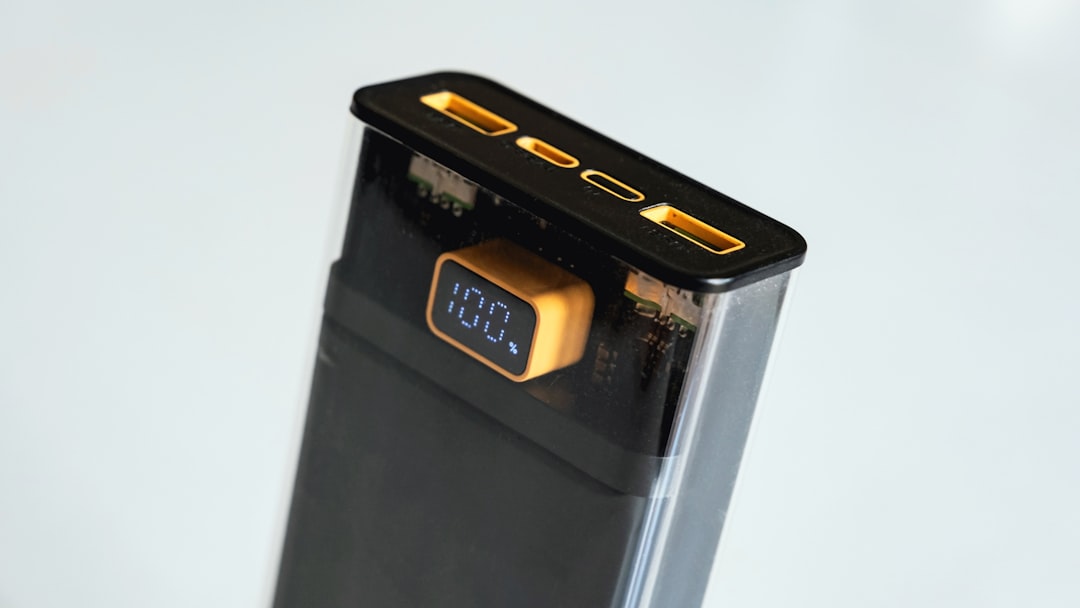
Imagine a world where online shopping is no longer a game of chance, where you can actually see how that shirt would look on you, or how a new couch would fit in your living room. This is the promise of interactive visuals. Using AI, companies can now create product images that customers can actually play with, letting them explore different variations - color, size, style - in real time. It’s a step beyond simply looking at a static image, it's a truly immersive experience.
Think about it - you no longer need to rely on your imagination, you can 'try on' clothes or see how furniture would look in your own space. This sort of personalized experience can significantly reduce the risk associated with buying something online. But there are some big challenges too. As we move forward with this technology, we need to ensure that these digital portrayals stay true to the real world and are sensitive to cultural differences. After all, we want people to trust what they see online, right?
Interactive visuals in e-commerce are fascinating in their potential to influence customer behavior. It's not just about pretty pictures; it's about providing a richer, more engaging experience that goes beyond static product images. While I'm not one for making claims about specific numbers, the research suggests some intriguing trends. Interactive visuals, by allowing customers to preview variations, seem to shorten the time it takes for them to make a decision. This could be due to a reduced cognitive load, as the visuals provide a clearer and more digestible way to understand the product's features.
However, there's a flip side to consider. Implementing such interactive features requires significant technological resources, and not every platform has the capacity or the budget. While the potential for increased engagement and reduced returns is tempting, the cost of implementation needs to be weighed against the benefits.
On the other hand, the possibility of leveraging interactive visuals to promote cross-selling is exciting. If a customer can easily visualize how different products might work together, it could lead to a higher average order value, which is a win for the retailer.
This raises further questions about the potential for integrating interactive visuals with user-generated content. Could we imagine a scenario where customers could not only preview products but also share their customized versions with others, further enhancing the product discovery process?
Overall, interactive visuals are a compelling development in e-commerce, but as with any AI-driven innovation, a critical perspective is essential. The potential benefits are exciting, but the practical considerations need careful analysis.
AI-Generated Product Images Balancing Innovation and Cultural Sensitivity in E-commerce - AI automation streamlines 75% of product image workflows
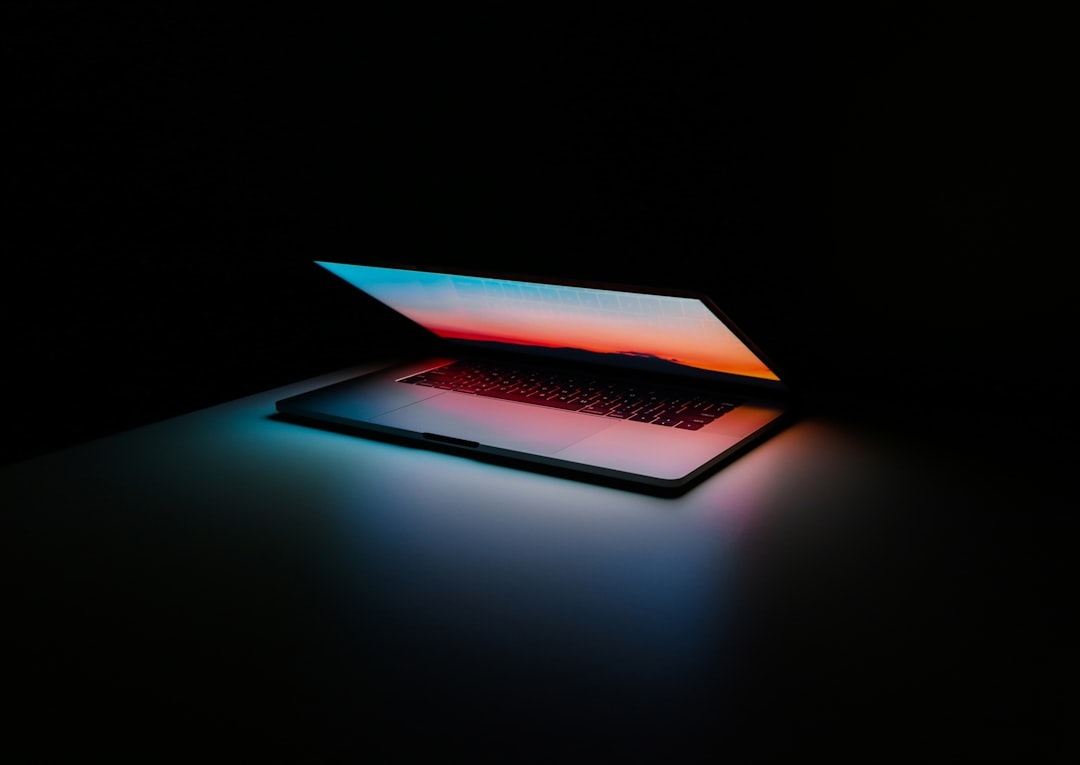
AI is changing the way e-commerce companies create product images. It's now possible to automate up to three-quarters of the image workflow, saving time and money. This makes it easier to create more eye-catching product listings, which is essential in today's competitive market. With AI-generated visuals, businesses can create unique and customized marketing content that perfectly matches their brand identity. But we have to be careful as this technology evolves. The images need to be accurate and culturally sensitive to avoid misleading customers. Businesses must prioritize quality and integrity in how they present their products to maintain consumer trust in an increasingly AI-powered world.
It's fascinating how AI is streamlining product image workflows in e-commerce. I've seen reports claiming that AI can automate up to 75% of these tasks, taking over roles traditionally handled by human designers. This shift means that image creation, from background removal to resizing and color adjustments, is becoming increasingly algorithmic. While it's undeniably efficient, it does make me wonder if there's a potential loss of that human touch that can bring unique creative vision to a product image.
But there are also exciting opportunities for AI to go beyond simply replicating what humans do. AI can now analyze a wealth of data—not just images but also customer feedback and text descriptions—to create more personalized product visuals. This opens up avenues for truly customized product experiences, where AI adapts images based on user preferences, even potentially adjusting the imagery to reflect cultural sensitivities. It's a significant step towards a more inclusive and user-friendly online shopping experience.
The research suggests that AI can even create product images with incredibly realistic textures and reflections, going beyond basic photo editing. The question remains, however, whether these realistic depictions are always accurate and beneficial. It's interesting to consider if hyperrealistic imagery could mislead consumers into having unrealistic expectations about the physical product.
One of the most intriguing aspects of AI-powered product image generation is its ability to learn and adapt based on user behavior. This adaptive learning, coupled with AI's ability to incorporate various data sources, opens up the possibility of creating visuals that truly reflect customer preferences. It's as if the AI is becoming a perceptive assistant, understanding what resonates with customers and refining images to achieve better engagement and, ultimately, higher sales. However, this raises a concern for me: who is monitoring and evaluating this AI-driven evolution? Is there a risk of AI becoming too narrow in its focus, potentially neglecting other aspects of product presentation or customer needs?
The role of AI in e-commerce is becoming increasingly complex, and it's crucial to maintain a critical perspective. We need to acknowledge both the remarkable potential of this technology and its limitations. While AI can streamline workflows and create truly compelling imagery, it's vital to ensure that the human element remains a part of the process, providing oversight and ensuring that these technological advancements are ethically and culturally responsible.
AI-Generated Product Images Balancing Innovation and Cultural Sensitivity in E-commerce - Cultural sensitivity checks essential in AI-generated content
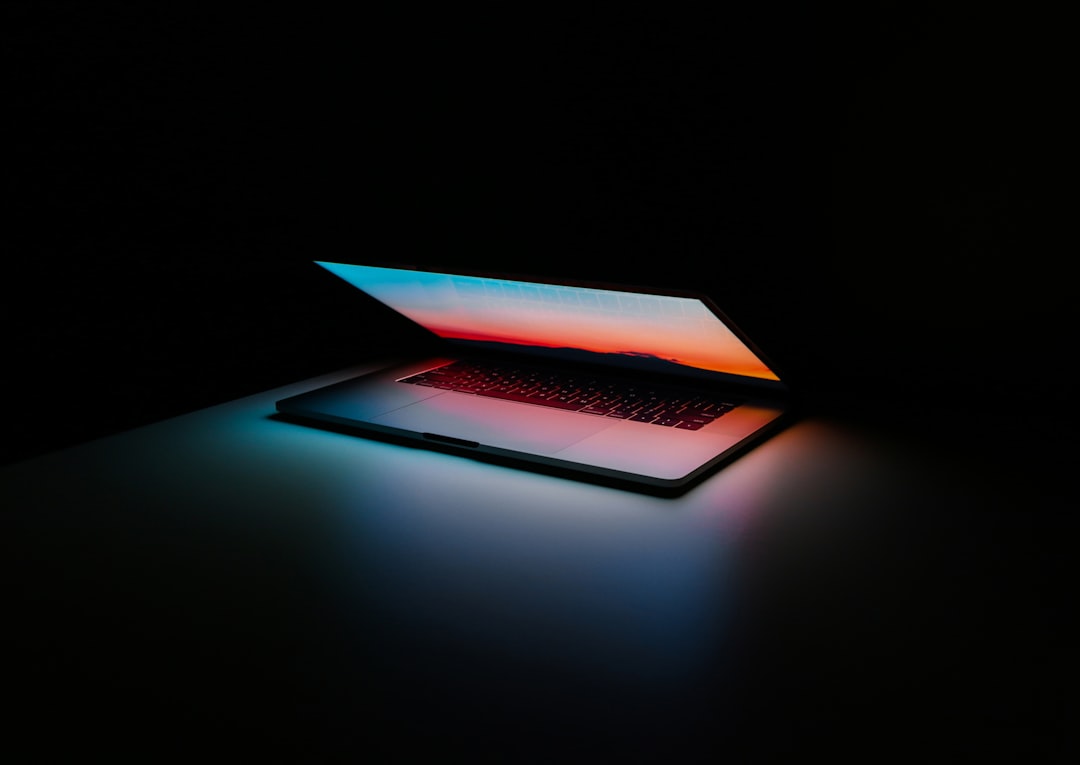
The rise of AI-generated product images in e-commerce has brought to light a critical need for cultural sensitivity checks. While AI can create impressive images, it often struggles to understand the subtle nuances of different cultures. This can lead to images that are insensitive or even offensive.
To prevent these issues and ensure inclusivity, it's crucial for companies to have a system in place where human editors or cultural consultants review AI-generated images. These experts can identify potential problems and ensure the visuals resonate well with diverse audiences. This step not only helps maintain a positive brand image but also demonstrates a commitment to ethical practices in a global marketplace.
As AI continues to advance, the importance of balancing technological innovation with cultural sensitivity will become increasingly important for the future of e-commerce.
AI-powered image generation holds immense potential for e-commerce, but it's a double-edged sword. While it can create dazzling and personalized visuals, it can also inadvertently perpetuate cultural stereotypes. This is where cultural sensitivity checks come into play. Imagine, for example, an AI generating product images for a clothing line that uses a culturally significant symbol in a way that's insensitive or even disrespectful. Such a misstep could damage a brand's reputation and alienate a large segment of its potential customers.
The issue isn't just about avoiding offense; it's about fostering meaningful connections. Research shows that customers are more likely to buy products from brands that demonstrate a genuine understanding of their culture. This translates to better brand engagement, higher conversion rates, and ultimately, increased revenue.
But here's the catch: AI models are only as good as the data they're trained on. If the training data is skewed or lacks diversity, the generated images might inadvertently perpetuate harmful stereotypes. For example, an AI might produce images that reinforce outdated and inaccurate views about specific cultures. This can lead to unintended consequences, making it even more crucial to incorporate robust cultural sensitivity checks.
The good news is that there are solutions. For instance, diverse teams of experts can be involved in training AI image generators. They can provide valuable insights into different cultural contexts and help ensure that the resulting images are culturally appropriate and respectful.
This is not just about avoiding PR disasters; it's about creating an inclusive and engaging experience for customers. It's about making sure that everyone feels seen, heard, and valued. The potential for AI to revolutionize e-commerce is enormous, but we need to be mindful of the cultural implications. The future of e-commerce might be AI-powered, but it's also deeply human, demanding that we use this technology with care and sensitivity.
More Posts from lionvaplus.com: