Create photorealistic images of your products in any environment without expensive photo shoots! (Get started for free)
7 Free Stock Image Platforms That Support Product Photography AI Training in 2024
7 Free Stock Image Platforms That Support Product Photography AI Training in 2024 - Raw Stock by Pixelperfect Creates AI Model Compatible Images From Physical Product Photos
Pixelperfect's Raw Stock project aims to bridge the gap between traditional product photography and AI image generation. By converting real-world product images into a format suitable for training AI models, Raw Stock contributes to the expanding field of AI-driven product visualization. The importance of high-quality product photography for boosting online sales is well-established, and this new approach could play a key role. As AI models are increasingly used to generate product images, the significance of good training data becomes even more apparent. This means a focus on composition, lighting, and context during image capture or selection for AI training. The goal is to cultivate AI models that create images reflective of a brand's identity and style, providing a smoother, more consistent presentation across various platforms. The increasing availability of tools that allow for editing and customization of generated images furthers this trend. Businesses can now achieve professional-looking and branded visuals relatively easily. This democratization of high-quality product imagery can be a valuable asset, especially for smaller businesses operating in a highly visual online space.
Raw Stock, developed by Pixelperfect, takes a different approach to stock imagery by focusing on making physical product photos suitable for AI model training. Their system essentially translates real-world product images into a format that AI systems can easily understand and learn from, specifically for e-commerce purposes.
This method employs cutting-edge AI techniques like GANs to convert the physical photos into high-quality digital versions, significantly improving their usefulness in AI-powered marketing efforts. Unlike some other stock sources, Raw Stock's emphasis is on capturing the fine details of the physical product—lighting, textures, and intricate aspects— ensuring that AI training datasets reflect the product accurately. This is crucial for getting realistic results.
They've also engineered the process to be adaptable to different industries. The needs of, for example, fashion and electronics in terms of image quality can be quite different, and Raw Stock seems to be designed to address those variations. Furthermore, by using AI, they can generate product imagery in various settings or environments, potentially saving time and costs associated with traditional staging and photography.
Raw Stock claims it can even react to trends and changing consumer preferences, dynamically altering product images to remain relevant. This ability, if it actually works as claimed, could prove extremely valuable. Of course, the core requirement for any AI model is the quality of the input data. Raw Stock ensures high-resolution images with proper formatting, attempting to minimize quality loss during the conversion process.
Their platform is supposedly compatible with a wide range of AI frameworks, helping to integrate into various e-commerce systems. Additionally, their technology can perform tasks like removing backgrounds and enhancing colors. Research has suggested that consumers engage more with clean and crisp images, potentially leading to higher conversions.
Generating multiple variations of the same product through AI is also a feature they promote, possibly aiming at better capturing a customer's attention. While there's research indicating that product variations in images can increase engagement, it's still an open question whether this feature truly yields significant benefits in practice.
7 Free Stock Image Platforms That Support Product Photography AI Training in 2024 - Pexels Expands Dataset Library With 50k Ecommerce Ready Product Shots
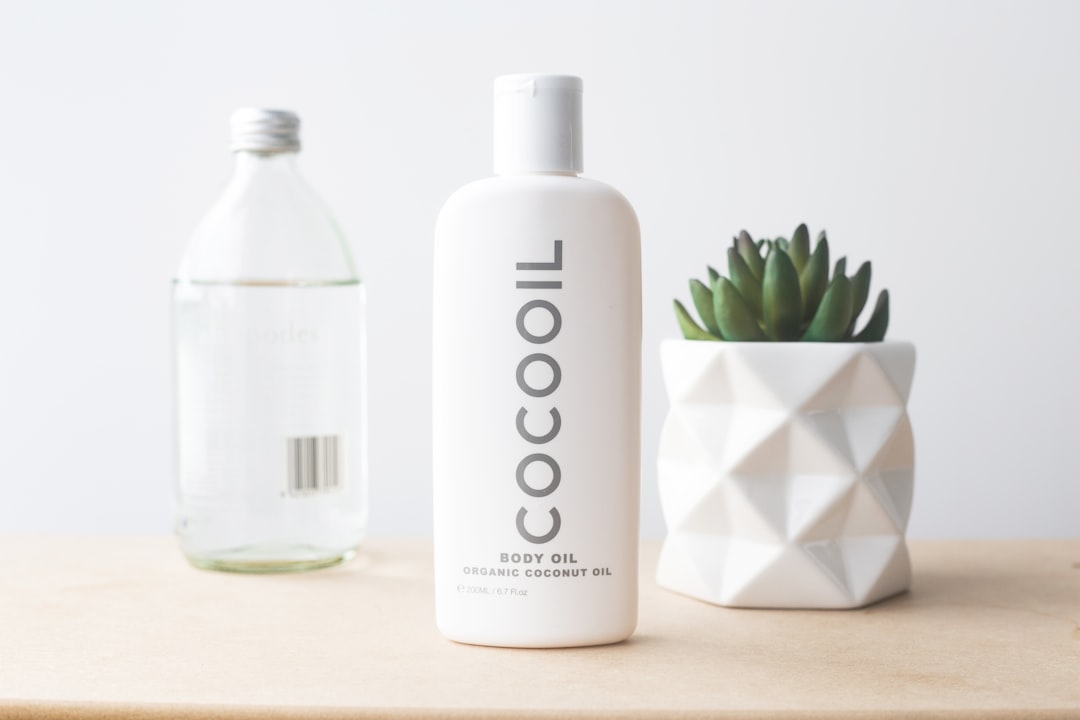
Pexels has recently boosted its collection of free-to-use images by adding 50,000 new product photos specifically designed for online stores. This move is clearly intended to help e-commerce businesses and marketers create more engaging and visually appealing product presentations. Pexels has always been known for its vast library of high-quality, royalty-free images and videos, all of which can be used without needing to give credit to the photographer. Since good product images are crucial for driving online sales, this new addition could be very helpful for businesses looking to improve their visual marketing.
While Pexels' expansion is noteworthy, it's important to realize that the competition for image resources is strong. Platforms like Burst (from Shopify) and Unsplash also provide substantial collections of high-quality photos suited to e-commerce. The overall trend toward higher-quality visual content across e-commerce is clear, and these platforms play an important role in making it easier for businesses of all sizes to find images that work for them. It remains to be seen how impactful this addition to Pexels' library will ultimately be in a field with so many other players.
Pexels' recent addition of 50,000 product images specifically designed for e-commerce is a good example of how readily available, high-quality visuals are becoming increasingly important in online retail. We know that well-chosen images can significantly boost sales conversions, potentially by a substantial margin, which makes this kind of resource interesting.
The broad range of product categories within Pexels' new dataset is useful for training AI models to generate visually appealing content that's more attuned to different customer groups. This is quite promising because it suggests the possibility of using AI to create more effective, targeted marketing campaigns and refine brand messaging.
From a research perspective, the idea of consistent image quality being linked to greater consumer trust is fascinating. A polished and visually appealing presentation doesn't just look better, it appears to boost confidence in a brand and its offerings. This reinforces the idea that high-quality product photography is about more than aesthetics—it's about building confidence in a brand.
The integration of AI into product image generation also raises intriguing points about efficiency. AI-powered image creation can drastically reduce the time it takes to bring products to market. Instead of solely relying on traditional photoshoots, companies can now experiment with generating a variety of image variations and adjust them on-demand.
One area where Pexels' dataset seems to contribute is the importance of effective product staging in images. How a product is presented visually can influence how customers perceive its value and even affect their willingness to pay more. It's an area ripe for further exploration, especially as it relates to how AI models might be trained to understand these aesthetic nuances.
Exploring variations within the same product's imagery via AI-generated versions is also interesting. Initial studies suggest that offering diverse angles or styles can increase customer engagement and allow companies to better understand consumer preferences. It's an area where there's still a lot to be investigated regarding the effectiveness of A/B testing different variations in real-world scenarios.
The potential to weave 3D product models and high-resolution images together seems like a logical extension of the trend we're seeing. It allows for interactive shopping experiences which, from the data, appear to keep people more engaged on product pages.
The idea of dynamically updating visuals based on inventory or real-time changes is worth noting. If implemented properly, this could address frustrating situations where customers see products in online stores that are not actually available.
The level of detail within product images, whether it's texture, material, or other intricate features, is also crucial. High-quality visuals that showcase these elements help customers gain a clear understanding of the products they are buying which, in turn, can lead to fewer returns.
As e-commerce continues to change, the capability of AI to dynamically adapt images to current trends and new data suggests that the future of product visuals will be more driven by insights and data analysis than ever before. This dynamic ability to adjust marketing and product presentation on the fly is likely to provide a significant advantage in a highly competitive market.
7 Free Stock Image Platforms That Support Product Photography AI Training in 2024 - Flickr Creative Commons Now Tags Studio Photography For Machine Learning
Flickr, with its extensive collection of over 500 million Creative Commons-licensed images, has become a valuable resource for AI training, especially in the area of product photography. This vast library, built on the principle of open access and sharing, is now specifically tagging studio-quality photos to be more readily available for machine learning projects. Since its 2004 integration with Creative Commons licenses, Flickr has provided a platform for photographers to readily share their work under flexible usage terms, leading to a diverse and expansive dataset beneficial for both artistic expression and AI development.
This ease of access for AI researchers is crucial for the continued refinement of algorithms used in product image generation, which plays a growing role in ecommerce. However, as AI technology becomes more sophisticated, the use of Creative Commons images for training raises important questions around legal and ethical boundaries. The relationship between AI-generated output and the creative works that fuel them is a complex and still-developing area, demanding careful consideration. Essentially, the question of how historical photographic records are being recontextualized by modern technology continues to be debated as AI's influence on various aspects of image making grows. The implications of this for both individual creators and the broader visual landscape remain to be fully explored.
Flickr, with its vast library of over 500 million Creative Commons-licensed images, offers a rich resource for training AI models, particularly in the realm of product photography. This massive collection, built by a community of photographers willing to share their work under flexible licensing terms, provides a diverse set of images that can improve the variety and accuracy of AI-generated product visualizations. The Creative Commons licensing model, integrated into Flickr since 2004, makes this possible.
The tagging system Flickr employs is a crucial asset. It allows for better organization and retrieval of images, making it easier to curate datasets for specific product photography training needs. AI models benefit from this organized metadata, which lets them quickly find relevant imagery for learning. Researchers have already leveraged similar Creative Commons image libraries to train AI algorithms, for example in facial recognition tasks. It's intriguing to consider how this massive dataset of product-related imagery could help improve the quality of outputs, potentially making AI-generated product photos more realistic.
It's fascinating how Creative Commons licenses have become a catalyst for innovative uses in AI, including e-commerce. Businesses can readily tap into a pool of free images to train their own AI image generators, circumventing the potentially high costs of procuring custom image datasets. The tagging system doesn't just improve search but also gives insights into current trends. This dynamic aspect of image data is particularly useful for AI, as it can create product imagery that reflects the latest consumer interests, allowing companies to adapt their visuals quickly.
However, this abundance of images brings a trade-off: the quality can be uneven. The sheer size of Flickr’s database necessitates a nuanced approach to data selection. Simply using every image might not be ideal, as a model trained on images of mixed quality could produce outputs with inconsistent quality. The research community is grappling with questions about how this variety affects training outcomes.
Moreover, the wide range of images showing various product staging techniques presents an opportunity. AI models exposed to these diverse presentations might develop a better understanding of how to create visually compelling product shots. This resource might be particularly valuable for smaller businesses lacking access to expensive studio setups or design expertise.
There's also the exciting prospect of extending the use of Flickr's dataset beyond 2D images. Perhaps, AI models could leverage these images to create 3D product visualizations, further enriching online shopping experiences. Interactive product viewing is already growing in popularity, and this could be a key development in the evolution of e-commerce.
Interestingly, the connection between high-quality images and consumer trust has also been observed. This suggests that AI models trained on Flickr's better-categorized and appropriately tagged images could generate outputs that reflect a brand's professionalism. For companies striving to build trust, the ability to generate images with a polished, consistent aesthetic could be very beneficial.
Creative Commons is a two-sided coin. While it democratizes visual content and is a boon for smaller businesses, it also carries a risk of oversaturating the market with similar visuals. Companies will need to carefully evaluate the usefulness of the images they choose to ensure their visuals stand out in a sea of readily available imagery.
One potentially transformative aspect is the ability to use Flickr's image data to inform real-time adjustments to product imagery. Integrating AI with Flickr's image database could pave the way for A/B testing in the visual realm. This flexibility could lead to significant marketing gains by allowing businesses to respond to customer behaviors rapidly in an ever-changing online marketplace.
The intersection of Flickr's massive image library and the burgeoning field of AI for product photography offers a rich ground for research and innovation. While challenges remain, the potential to improve the quality, diversity, and impact of product visuals is undeniable.
7 Free Stock Image Platforms That Support Product Photography AI Training in 2024 - Opencollection Shares Fashion Product Images From 200 Independent Labels
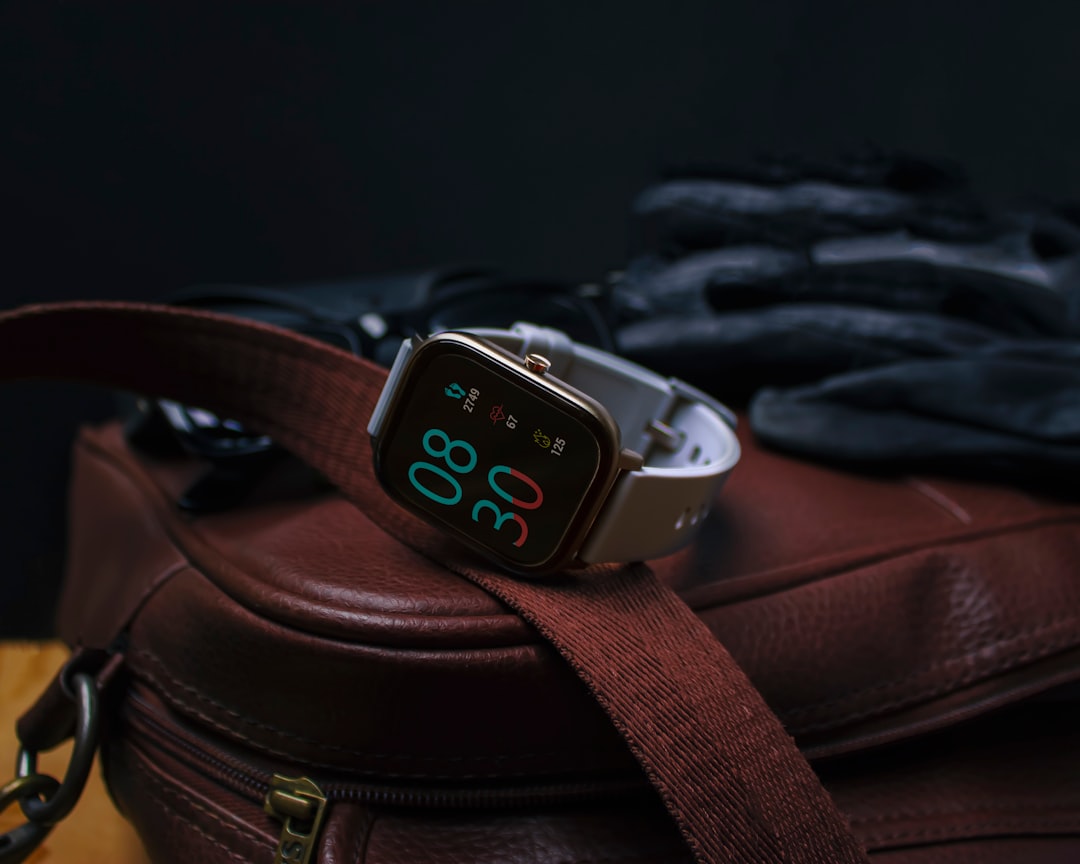
Opencollection has provided a substantial resource for the e-commerce sector by releasing a wide-ranging set of fashion product photos from 200 smaller fashion brands. This extensive collection includes 44,000 high-definition images along with details like brand names, seasons, and intended customer profiles. The rich metadata is useful for organizing and filtering images, potentially making it more useful for training AI models. The surge in AI-driven product image generation highlights the need for high-quality data to train the AI systems effectively. Opencollection's collection could be a valuable resource in this area. This kind of dataset helps not only independent designers but also shows the increasing connection between fashion and technology in the online space. It reinforces the idea that carefully selected product photos can foster consumer confidence and play a vital role in driving sales in the competitive world of online retail. It will be interesting to see if this approach can help smaller labels in the long run.
Opencollection offers a curated collection of fashion product images from around 200 independent brands, presenting a diverse range of styles and aesthetics. It's interesting how this platform focuses on smaller, less mainstream brands, as opposed to the usual big-name labels seen in standard stock photography. This could be beneficial for businesses seeking a unique visual identity. The dataset includes approximately 44,000 high-resolution images, along with details such as product categories and descriptions. This level of detail is helpful for sorting and filtering, and could make it a strong training dataset for AI models focused on product image generation.
Some of the datasets also include metadata like brand names, the season the image relates to, and the intended age group of the clothing. This makes searching and filtering much easier. You can imagine how this could be helpful if, say, you wanted to train an AI model to generate images of fall clothing aimed at teenagers.
While it's great to see datasets focused on a specific area, such as this fashion niche, it remains to be seen how well these resources will serve broader AI training needs. Datasets like this, with details like brand name, season, and target audience, raise the question of whether we'll see a trend towards more specialized datasets in the future. Could it be that the era of large, generalized datasets is fading, to be replaced by smaller, highly curated ones? It's an interesting point to consider given the rise of AI in e-commerce.
Of course, high-resolution images are crucial for e-commerce, and Opencollection emphasizes this point. It's widely accepted that higher-quality images lead to fewer returns, as customers have a better idea of what they're buying. This platform's collection could provide a valuable resource for anyone developing or refining AI image generation systems. By training AI on a diverse range of clothing styles and brands, it may be possible to produce outputs that better capture the subtleties of various fashion aesthetics.
The overall aesthetic consistency across product images is crucial for brand recognition. It makes sense that a business would want consistent visuals in their online stores, as it's been shown to boost consumer trust. With Opencollection's focus on independent labels, the platform might provide a starting point for AI to learn how to translate a brand's unique personality and visual identity into images.
Finally, there's the intriguing question of how easily Opencollection images can be integrated into existing e-commerce systems. We're seeing a push toward making these image resources readily available for a wider range of online stores and platforms. The fact that these images could potentially be used to update product displays based on seasonal trends or recent consumer interests could be a significant advantage. We'll need to see how effectively this works in practice. If the ability to create and update product images based on real-time information becomes a reality, it would represent a significant leap forward in online retail.
While it's still early to fully gauge the impact of Opencollection on AI-powered product photography, it's a platform worth keeping an eye on. Its focus on fashion from independent designers presents a unique opportunity for those working on improving the visual experience in online stores. Whether it can truly address a wider range of training requirements remains to be seen.
7 Free Stock Image Platforms That Support Product Photography AI Training in 2024 - Shopistry Launches Open Source Dataset Of 3D Generated Furniture Photos
Shopistry has released a publicly available collection of 3D-rendered furniture photos intended to help train artificial intelligence (AI) models used in product photography. This dataset, featuring a variety of 3D furniture images, is aimed at improving AI's ability to generate realistic and diverse product visuals for online stores. This move appears to be part of Shopistry's broader goal of streamlining the creation and management of online businesses, especially for smaller operations. Notably, while some image platforms are wary of using AI-generated photos, Shopistry is offering this dataset to potentially improve the realism of product images within e-commerce. While this initiative could be valuable for refining AI-generated product photos, it also introduces considerations about whether reliance on synthetic images negatively affects the perceived authenticity or brand character of products presented online.
Shopistry's release of an open-source dataset of 3D-generated furniture photos is a noteworthy development in the world of e-commerce imagery. By creating a library of rendered furniture images, they've sidestepped the need for traditional product photography, allowing for greater flexibility in showcasing products in various settings. This is interesting because it opens up possibilities for more dynamic product presentations tailored to specific customer interests, without requiring a huge number of physical photoshoots.
It's also noteworthy that the dataset is designed to adapt in real-time. They claim it can adjust image features based on changes in inventory or emerging trends, which could be incredibly useful for avoiding customer frustration when a product shown online isn't available. This idea of on-the-fly adjustments to visual content has potential for making e-commerce experiences more seamless.
Another benefit is the potential cost reduction for smaller businesses. 3D-rendered images can help smaller players to present their goods in a variety of scenarios, perhaps enabling them to compete more effectively with larger brands that may have larger budgets for more traditional photography. Whether it genuinely improves their competitive standing is certainly a question that warrants future examination.
The dataset's creators are also aiming to improve user engagement. They focus on creating visually appealing images, hoping that high-quality and well-composed product visualizations will lead to more conversions. It's a common-sense idea, given that people tend to be more receptive to professional-looking imagery, but the specifics of how that translates into improved business outcomes is still a matter of further exploration.
Shopistry's approach also includes robust metadata tagging within the dataset. This tagging can enhance the training process for AI models because it allows for easier filtering and categorization of images. This feature could be useful in tailoring product presentations to target audiences, allowing for more effective marketing campaigns.
It's widely observed that high-quality product visuals build trust with consumers. In this context, the Shopistry project's focus on realistic 3D renderings could lead to increased consumer confidence. Whether it actually achieves this in real-world scenarios remains to be seen, but it certainly seems to be a logical goal.
Furthermore, the use of high-quality 3D renderings can be seamlessly integrated with augmented reality (AR) applications. This could be a significant development in the future of online shopping, because shoppers would be able to see how furniture would look in their own homes. This has the potential to improve user experiences and may potentially lead to lower product return rates.
The capability to create variations of a single product image is also a valuable aspect of Shopistry's approach. The capacity to adapt to a wide range of potential customer preferences could make it more effective in addressing the needs of niche market segments.
By offering clear, detailed visuals, the Shopistry initiative could reduce the incidence of customers returning unwanted products. If the generated images accurately represent the product's features, customers are more likely to make informed decisions about their purchases. This is certainly beneficial to all parties.
The dataset's open-source nature encourages collaboration within the e-commerce community. This openness might facilitate the creation of even more comprehensive and specialized datasets, leading to more innovative product visualization techniques in the future.
Overall, Shopistry's dataset represents a fascinating development in the world of online product presentation. It highlights a potential shift away from traditional photography toward more flexible and adaptive digital rendering. However, further research and real-world experimentation are needed to determine how this new method truly impacts consumer behavior, purchasing decisions, and the overall efficiency of e-commerce platforms.
7 Free Stock Image Platforms That Support Product Photography AI Training in 2024 - ImageSpring Offers Multi Angle Product Photography From Small Business Users
ImageSpring focuses on helping small businesses by providing a service that captures products from multiple angles. This is important because in today's online marketplaces, the quality of product photos is crucial for increasing sales. Small businesses, in particular, need to create a good visual impression to stand out and compete with larger companies, and high-quality photos are a key element of that. This service appears to offer a detailed and imaginative way to showcase products, which can encourage buyers to trust a brand and be more likely to make a purchase. There's a wider trend toward using AI to create product images more easily, and ImageSpring's services seem to fit in with that trend by blending more traditional photo skills with modern technological approaches. Overall, it demonstrates the growing emphasis on visual aspects in online business, particularly for those just getting started in the competitive e-commerce arena.
ImageSpring focuses on providing multi-angle product photography, which is especially useful for smaller businesses that might not have extensive photo resources. It's about getting a product seen from a variety of perspectives, which research indicates can lead to improved customer understanding and, possibly, higher sales. Their approach potentially cuts down on the time and money needed for traditional product photography. A single product shoot, with multiple angles, can be labor-intensive, but ImageSpring's tech might streamline this significantly.
Interestingly, they also utilize AI to generate various background scenes, letting businesses present products in different settings without needing a bunch of individual photo shoots. It can also be a quick way to adapt product images for things like seasonal sales or changes in consumer tastes. It's been suggested that well-done product visuals from different angles also build trust in a brand. This could translate to fewer customers hesitating to buy things online, improving brand perception.
Humans tend to grasp things faster visually than through words, and multiple angles play to this. People can virtually rotate or manipulate a product in their minds, which can create a more immersive shopping experience compared to just seeing a single image. It's becoming more common to link product imagery directly to inventory databases. ImageSpring seems to be part of this trend, allowing users to quickly change or remove images if a product is no longer available, helping to avoid customer disappointment.
The quality of lighting and surface textures within these shots matter a lot for online sales. If images capture material details well, it can potentially increase customer happiness and, perhaps, lead to fewer returns. It’s a way for smaller companies to build a look and feel for their brand, tailoring the product photography to their marketing strategies without always relying on external photography firms. This might also give smaller companies a slight edge as consumer preferences shift and change. E-commerce has to adapt quickly, and features that let businesses change or enhance product imagery in a timely fashion could give them a boost.
7 Free Stock Image Platforms That Support Product Photography AI Training in 2024 - StyleLens Database Features Lifestyle Product Images From Creative Professionals
StyleLens provides a database filled with lifestyle product images contributed by creative individuals. This collection offers a diverse range of visuals that can help e-commerce businesses present their products in a more realistic and engaging way. The platform's emphasis on lifestyle photography aligns with the growing trend of showcasing products within everyday contexts, fostering a stronger connection with potential buyers. As AI becomes more integrated into generating product images, having a database of diverse, authentic photos like the one provided by StyleLens becomes increasingly important for building well-rounded and effective AI models. The ability to find high-quality images that reflect a desired brand aesthetic and target audience is key for smaller online businesses competing in a visually-driven marketplace. While there are many stock image sources, the unique nature of StyleLens’s curated collection of lifestyle images can potentially help businesses stand out. The effectiveness of this approach will depend on the quality and diversity of the photos available within the StyleLens platform and how successfully they capture the unique visual styles that different businesses require. It remains to be seen whether StyleLens’s collection can compete effectively with other free stock image platforms but the platform’s approach to lifestyle product photography has potential for improving the overall visual appeal of online stores.
StyleLens offers a collection of lifestyle product images contributed by various creative professionals. This database aims to provide a diverse range of visuals suitable for training AI models for e-commerce purposes. It's interesting how they've managed to capture a variety of aesthetics, which is crucial for AI models that need to adapt to the differing needs of various product categories and brand styles. The speed at which StyleLens can churn out images in diverse styles is also noteworthy. Traditional photography processes can be slow and require extensive planning, but AI models could leverage these kinds of image libraries to rapidly create variations of product shots in less time.
While StyleLens provides a database of lifestyle imagery, its emphasis on linking image datasets with contextual information like usage and customer demographics is potentially quite useful. It's not enough just to have a lot of pictures, the AI model needs to understand how and where those images are being used. It suggests that there's a move towards using AI to not just generate images, but also to predict how those images might influence customer behavior.
There's a clear focus on having the platform adapt to consumer behavior in real-time. It's conceivable that StyleLens could be used to tweak a product's visual presentation based on real-time customer activity. Whether it actually yields tangible results is unknown at this point. It is interesting, though, that the image creation process is not seen as static but as something that should evolve as customer behavior does.
One notable point is the versatility of this resource across industries. Electronics products, fashion items, and other goods all have different visual characteristics. A model capable of adapting its output to these differences would be a significant advantage in the e-commerce sector. It remains to be seen whether StyleLens' dataset is comprehensive enough to achieve this in practice.
The question of engagement is crucial in online shopping. Many studies show that people respond more positively to images that provide more visual information about a product. The more detailed the image and the more ways you can view a product, the better. This relates to the point that if a customer can more easily visualize a product, they might be less likely to return it.
StyleLens might be helpful in fostering brand consistency across different product lines, which can improve a brand's overall image. The question is if this can be efficiently achieved and translated into consumer trust. There's evidence suggesting a link between a strong visual brand identity and increased trust, but it's unclear how well StyleLens' database can help businesses achieve this in practice.
The fact that StyleLens can interface with different e-commerce platforms is significant. If the platform can seamlessly integrate with existing e-commerce systems, it may become easier to create and manage a large catalog of products, ensuring consistency in branding across online channels. It could streamline the updating of product images or promotional campaigns.
StyleLens' focus on high-resolution images means that fine details are retained, especially important for product imagery where visual cues can influence customer purchasing decisions. It's become more important to provide detailed information about the material of products and surface textures, helping a shopper to gain a more complete understanding before buying.
The way that StyleLens' data feeds into machine learning is significant for AI research. Datasets like this improve the quality of the output in the form of product visuals. This could, in turn, improve the accuracy of algorithms aimed at understanding consumer behavior. It's an interesting interplay between image generation and prediction.
StyleLens is actively tracking user interaction with the images it provides, giving valuable insights into customer behavior. By looking at how people engage with the images, it can refine future generation strategies. This is essential for creating effective product marketing campaigns that resonate with potential customers. This sort of iterative, data-driven approach is increasingly critical in e-commerce, where trends can change rapidly.
While StyleLens appears to have some potentially helpful features, whether it significantly improves the effectiveness of AI-driven product photography remains to be seen. Its effectiveness will ultimately depend on the extent to which it can refine AI model capabilities for creating better visuals that, in turn, drive sales.
Create photorealistic images of your products in any environment without expensive photo shoots! (Get started for free)
More Posts from lionvaplus.com: