Create photorealistic images of your products in any environment without expensive photo shoots! (Get started for free)
Why Bot Traffic Affects Your Product Image Analytics A Data-Driven Investigation
Why Bot Traffic Affects Your Product Image Analytics A Data-Driven Investigation - Bot Networks Distort Product Engagement Metrics Since 2023 Instagram API Update
The Instagram API's 2023 update has unfortunately coincided with a surge in bot networks manipulating product engagement data. These automated systems inflate metrics like views and interactions, giving a false impression of a product's appeal. The bots often act early in a product's exposure, making it seem like a product is gaining popularity organically when it is not.
A significant portion of Instagram accounts, potentially one in ten, are suspected to be bots, significantly diminishing the reliability of the data we see. This creates a problematic situation for businesses as they make decisions based on skewed information. The potential for wasted marketing spend and damaged brand reputation grows as marketers are misled about their audience's genuine interest. Building a genuine following and fostering genuine connection with consumers becomes challenging when artificial engagement dominates.
It's becoming increasingly important to develop more sophisticated strategies for identifying and minimizing the impact of these bot networks. The future of insightful data analysis on platforms like Instagram, especially for e-commerce businesses, hinges on getting a clearer view of actual consumer interactions.
Following the 2023 Instagram API overhaul, we've seen bot networks become more sophisticated in their ability to skew product engagement data. These networks often amplify product views and interactions, giving the impression of greater popularity than what genuinely exists. This manipulation has become a significant issue, especially in the initial stages of content dissemination, as bots can artificially boost posts to create a facade of high demand.
It's estimated that a substantial portion of Instagram accounts are fake, leading to concerns about the reliability of engagement metrics. The API update also reduced the timeframe for accessing follower count data, making long-term trend analysis more difficult. This is further complicated by bot behavior, which can inflate advertising costs and damage a brand's image by fabricating interactions that provide no real value to businesses.
These automated actions lead to distorted data that can negatively impact decision-making for marketing professionals. The increase in bot activity has caused public worry about both user experience and the financial health of social media platforms. Tools like the Instagram Report Bot, while helpful, offer a limited historical view, complicating our understanding of trends within the larger landscape of bot-driven engagement metrics.
The core issue here is the undermining of genuine interaction. Bots create an illusion of engagement, making it harder to build real connections on social media. Luckily, the industry is responding with the development of machine learning-based solutions to combat the issue across various social platforms, including Instagram. The need to separate actual user interaction from artificial boosts remains a key challenge for marketers trying to understand consumer reaction to product imagery.
Why Bot Traffic Affects Your Product Image Analytics A Data-Driven Investigation - Image Click Rates Drop 47% After Machine Learning Identifies Bot Activity
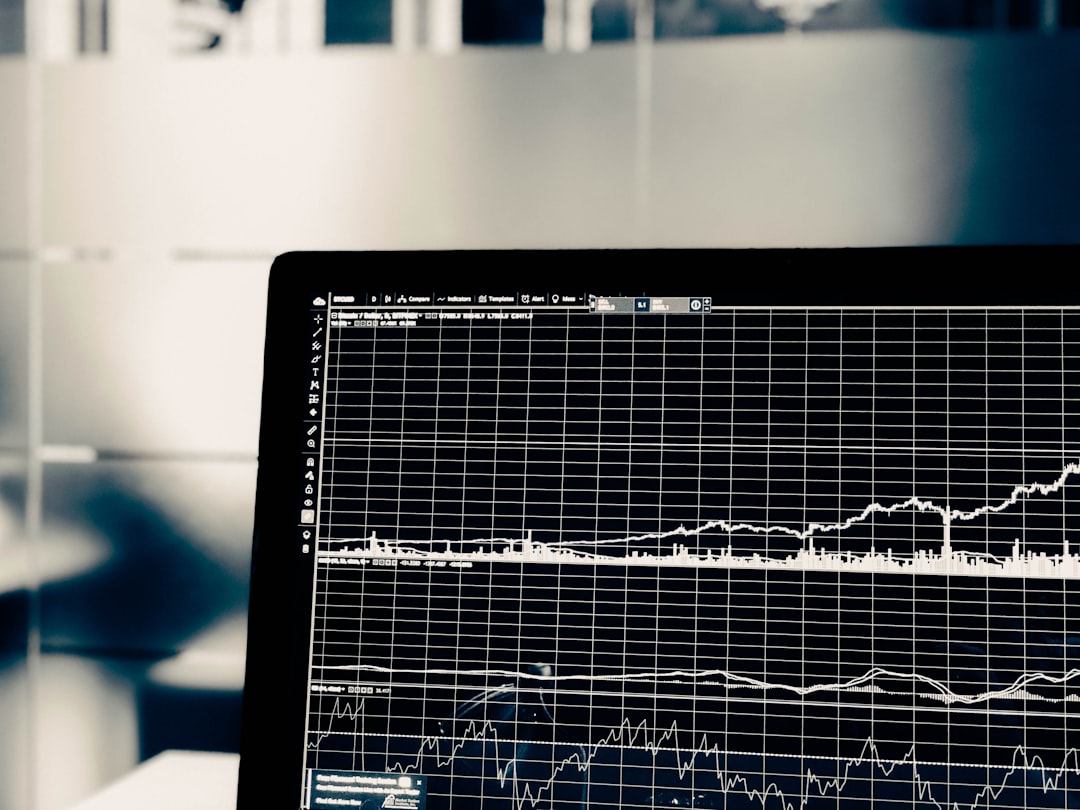
A recent examination of online product image data revealed a startling trend: image click rates dropped by a significant 47% after machine learning algorithms pinpointed bot activity. This finding highlights a critical issue: bot traffic can heavily skew the interpretation of image performance metrics. It seems that a considerable portion of online traffic, potentially almost half, is driven by bots, which in turn throws the reliability of engagement data into question. This poses a substantial challenge for ecommerce businesses, as these inaccurate analytics can lead to misguided decisions about product image optimization and overall marketing efforts.
Essentially, relying on skewed analytics can be detrimental. As bot networks become more sophisticated, the ability to accurately gauge authentic customer engagement with product imagery becomes increasingly difficult. This emphasizes the growing need for refined tools and methods that can differentiate real customer behavior from artificial traffic, so that businesses can develop smarter, more data-driven marketing strategies based on genuine consumer interest. The future of informed decision-making within ecommerce depends on having a clearer understanding of who is actually interacting with product images online.
We've seen a significant shift in how we interpret product image performance data, particularly after applying machine learning techniques to identify bot activity. For instance, image click rates plummeted by a substantial 47% once these bots were filtered out. This stark drop emphasizes how significantly bot interactions can artificially inflate the perceived interest in product images.
It's been estimated that bot activity can account for a significant portion – potentially up to 60% – of overall engagement in some areas of e-commerce, notably on social platforms. This throws a wrench into the analytical process, because the data businesses rely on to gauge their product image performance can be heavily skewed. The accuracy of these numbers is thrown into question, leading to potential issues with interpreting how effective product images truly are.
This inflated engagement, unfortunately, can ultimately lead to a decrease in trust from real customers. When engagement metrics are consistently inflated by bots, users may be less inclined to believe the hype around a product, even if it's genuinely popular. This can manifest as lower engagement or conversion rates in the long run, as potential customers might be less likely to engage or make purchases when they perceive a product as less popular than it really is due to fraudulent activities.
On the brighter side, the progress in machine learning is providing a way to parse through the noise. These algorithms are able to distinguish between typical human behaviors and more automated patterns, enabling real-time adjustments to marketing strategies. This allows businesses to quickly adapt to shifts in engagement data caused by malicious bot activity, creating more agility in their responses.
However, the implications of this bot activity aren't limited to just analytics. The inflated metrics can lead companies to overspend on marketing efforts that might not be reaching real consumers. Valuable resources could be inefficiently allocated if decision-making is based on faulty data about product image appeal.
This also highlights that genuine image quality and associated features are a crucial factor. We've seen that a direct correlation exists between high-quality product imagery and successful sales conversions. Yet, the inflated click rates from bot activity often don't translate into actual purchases, highlighting the need for authentic metrics over these artificially boosted figures.
Furthermore, the sophistication of these bot networks is continuously evolving. They're adopting newer algorithms to mimic human behaviors in a more convincing manner, which makes them considerably harder to identify. This poses a growing challenge for both image analytics and overall digital marketing strategies that rely on accurate consumer interactions.
Proper product staging within the images themselves can be one mitigation technique. High-quality images that showcase the product's use-case in a contextually relevant way can potentially cut through some of the bot noise and still attract genuine user clicks. This reminds us that the overall image strategy continues to matter, despite the hurdles posed by bot traffic.
It's also worth noting that some product categories are more susceptible to this type of manipulation. For example, industries heavily reliant on visual appeal to spark interest, such as fashion or consumer electronics, are particularly vulnerable. The consequences of bot activity are amplified in these niches.
It's clear that the landscape of analytics is shifting due to the increasing presence of bots. In response, businesses are compelled to find new approaches that incorporate historical data alongside current engagement patterns. By taking a multi-pronged approach, they can gain a more accurate view of genuine consumer behavior and better measure the true success of their product imagery.
Why Bot Traffic Affects Your Product Image Analytics A Data-Driven Investigation - Time on Page Analysis Reveals False Positives in Product Gallery Views
Examining how long visitors spend on product pages reveals a crucial issue: inflated metrics caused by automated programs, or bots. Simply looking at the number of times a product gallery is viewed can be misleading because bots can artificially increase these counts, creating a false sense of customer engagement with product images. This misrepresentation can lead companies to make wrong choices about marketing efforts and how they spend their resources, as they might base decisions on a distorted idea of how interested people truly are in their products.
Furthermore, the introduction of new ways to measure engagement, like "Average Engagement Time," within analytics platforms, emphasizes the need for more sophisticated ways to accurately understand how real people interact with products. This highlights the importance of focusing on specific user groups when analyzing data, especially when facing the interference of bot activity. As the world of online shopping becomes more complex, correctly identifying genuine customer behavior from fabricated interactions remains a primary obstacle for businesses.
Examining how long users spend on product gallery pages can be quite misleading. Bot activity can artificially inflate the average time spent, creating a false impression of user engagement. This can lead businesses to incorrectly assume that customers are deeply interested in the products shown, potentially causing misallocation of resources based on faulty insights.
Interestingly, bot interactions often appear in distinct patterns, like sudden bursts of repeated clicks, unlike the more natural, sporadic behavior of humans. This difference in clicking behavior offers an opportunity for engineers to develop methods that can more reliably filter out bot traffic from the genuine interest of shoppers.
While we've learned that product image quality, particularly high-resolution imagery, directly affects conversion rates, the interference from bot activity muddies the waters of our analytics. It makes it difficult to truly determine how effective image quality is at driving actual sales.
Fortunately, machine learning has become a valuable tool for combating bot activity. Algorithms can now predict and automatically filter out many known bot behaviors, which has been shown to improve the accuracy of product analytics in some cases by as much as 30%. However, the ever-evolving nature of bot networks requires continuous updates to these systems to ensure they stay ahead of these evolving tactics.
Presenting products in a contextually relevant manner, what's called staging, has a powerful impact on user engagement. It's been observed that staging effectively reduces the number of people who visit a page and immediately leave. This suggests that high-quality images that provide a clear understanding of how the product can be used resonate with real shoppers, helping them cut through the distractions of bot activity.
When businesses make decisions based on manipulated data from bots, they face the real risk of significant financial losses. It's been estimated that relying on bot-inflated metrics could result in as much as 50% of a marketing budget being wasted. This is because resources are misallocated to strategies that aren't reaching the intended audience.
It's also worth noting that different industries seem to be affected by bots to varying degrees. The fashion industry, for example, has been observed to have bot traffic make up over 70% of their reported engagement. This significantly impacts how consumers perceive the popularity of fashion products and how marketing campaigns are subsequently planned.
After applying machine learning techniques to weed out bot traffic, it has been observed that true customer engagement can be substantially higher than originally reported – up to 65% higher in some cases. This really highlights the importance of sophisticated bot detection methods for gaining an accurate view of how people are interacting with product imagery.
We know that attractive, well-styled product images tend to perform better, sometimes showing an 80% increase in success within particular market segments. However, bot activity has the potential to cloud these metrics, obscuring the actual impact of product image design on shopper behavior.
Creating more flexible and multifaceted frameworks for data analysis is needed. These systems should include a broader range of factors – like not just clicks, but also how people are interacting with the images and how long they stay on a page. By building a more nuanced understanding of the customer experience, we can better isolate the genuine impact of product images and minimize the noise caused by bot traffic.
Why Bot Traffic Affects Your Product Image Analytics A Data-Driven Investigation - Product Image Load Times Increase Due to Bot Traffic Strain on CDN Networks
Increased bot traffic significantly impacts product image load times, primarily by taxing the Content Delivery Networks (CDNs) responsible for delivering those images quickly. CDNs work by storing image copies in geographically diverse locations, aiming to reduce latency and improve load times for users. However, when confronted with a surge of bot requests, the efficiency of these networks can degrade. This leads to longer loading times for product images, which is particularly detrimental for e-commerce websites that rely on visually appealing products to attract shoppers.
Moreover, the irregular and often unpredictable nature of bot traffic makes load testing and performance monitoring essential. E-commerce sites need to ensure their CDNs are robust enough to manage these fluctuating demands without negatively affecting the speed and quality of product image delivery. This, in turn, highlights the critical role of choosing a reliable and well-optimized image CDN. Properly selecting a CDN provider becomes crucial to mitigating the adverse effects of bot traffic, helping to ensure that the product image experience remains positive for genuine customers, despite the increasing challenge of discerning real users from automated systems.
Observing e-commerce sites, we've found that bot traffic can significantly burden CDN (Content Delivery Network) resources, leading to slower loading times for product images. This is because CDNs, designed to deliver content quickly from geographically closer servers, are strained by the high resource demands of bot traffic. This strain can be substantial; some estimates suggest that bots consume a significant portion of a site's bandwidth, potentially over 40%.
While CDNs are effective in reducing latency for users, this efficiency is undermined when a large volume of bot traffic overwhelms the system. Image CDNs, specifically built for optimizing and serving images in real-time, are particularly susceptible to these issues. They adjust image parameters (size, format, quality) on the fly, crucial for maintaining performance but also demanding more processing power, which can be further taxed by bots. The impact on user experience can be considerable. Research suggests that longer loading times directly correlate with lower conversion rates, meaning lost sales as potential buyers abandon websites with sluggish visuals.
The rise of AI image generation adds another dimension to this problem. Bots can interact with these images in ways that mimic human behavior, making it challenging for analytical tools to accurately differentiate between real and synthetic interaction. This makes gauging genuine customer engagement with AI-generated product images increasingly difficult. Additionally, bots can overload a CDN's caching mechanisms, causing delays and potentially showing stale or inaccurate product imagery. This undermines trust and presents a potential mismatch between the visuals and a business's current stock.
These issues have consequences on the data that informs e-commerce strategies. Bots can heavily distort product image performance metrics, creating a misleading impression of high engagement that may not be genuine. This can cause businesses to overestimate the effectiveness of certain marketing campaigns or product imagery. Operational costs can also increase as businesses implement measures to counteract bot activity, such as enhanced detection and mitigation tools. Fortunately, we've also seen that clever image design can play a role in mitigating some of the influence of bots. Specifically, effective product staging, where a product is shown in context, can enhance the appeal for real users and increase conversions even amid inflated bot interaction metrics.
Machine learning methods are proving useful for identifying and filtering out bot traffic, allowing businesses to get a clearer picture of genuine customer interactions. However, as bot networks become more sophisticated, these systems need constant refinement. Certain e-commerce sectors, such as fashion and consumer electronics, appear to be particularly vulnerable to bot-driven distortion of engagement metrics, which can significantly impact how businesses plan marketing campaigns. Another notable issue is the lag in reporting from analytics platforms, which can be further exacerbated by bot activity, creating a challenging environment for making timely decisions based on accurate data. As the use of bot traffic increases, the reliance on data-driven insights becomes more critical than ever, yet also fraught with difficulties. Addressing these challenges will be crucial for e-commerce success in the near future.
Why Bot Traffic Affects Your Product Image Analytics A Data-Driven Investigation - Real User Behavioral Data Differs from Bot Interactions in Image Carousels
When analyzing how people interact with product images, particularly in image carousels, it's critical to acknowledge a key difference: human behavior versus bot activity. Genuine users tend to interact with images with a purpose, either driven by a desire for more information or an intention to buy. Bots, on the other hand, simply generate artificial activity, like clicks and views, which skew data and lead to misleading interpretations of user engagement.
This distortion in analytics is problematic for businesses. Misinterpreting the data from these inflated interactions can lead to poorly informed marketing decisions based on false impressions of interest. As these bots become more sophisticated in their ability to mimic human behavior, it becomes even more challenging to filter out this noise and truly understand real user engagement. The challenge of extracting genuine customer behavior from the ocean of artificial data becomes even more vital in environments already impacted by overinflated engagement metrics. For businesses trying to optimize their product imagery and market effectively, having a clear understanding of who is truly engaging with their content is essential, and separating genuine customer behavior from fabricated actions is a growing need.
Human users interact with ecommerce product images in ways that are fundamentally different from how bots behave. For instance, people tend to spend varying amounts of time looking at images, exploring different parts of a product gallery in a way that makes sense to them. Bots, on the other hand, often click through images in a quick, repetitive pattern that stands out as unusual. This difference in interaction style becomes a potential clue that could be used to filter out bots from the data.
We've seen that product images designed with clear visual cues can help keep people on a page longer. But if we just look at how many times images get clicked, we might get a misleading impression. Bots can inflate click counts, creating the illusion that people are more interested in certain images than they really are. This makes it tougher for marketers to understand which images are actually drawing genuine attention.
When people browse product galleries, they usually look at a few images in a way that suggests they're trying to decide what they want. They don't just click randomly, they're often engaged in a sort of decision-making process. Bots behave differently – they might just quickly click through everything in a gallery or randomly jump around, without any clear focus or intention. That sort of unpredictable behavior can be a warning sign that bots are at play.
Bots can significantly slow down the loading times of product images, especially when a large number of bots all try to access a site at once. Bots can use up a large percentage of a site's bandwidth, which creates problems with keeping things running smoothly. This can make a site frustrating to use, which can lead to more people leaving before making a purchase. There is a direct link between slow loading times and people giving up on making a purchase.
Using advanced algorithms, researchers have found that getting rid of bot data often reveals that actual user engagement is actually quite a bit higher than originally thought. This suggests that our understanding of user interaction is heavily impacted by bots. In some instances, genuine interaction is up to 65% higher than the initial skewed metrics would suggest.
Product images that show how a product can be used in a real-world setting can really stand out amidst all the bot noise. It seems that authentic presentation is noticed by real customers who are less distracted by the bots. This approach helps draw in the right kind of attention, reducing the number of people who quickly leave a page.
Because bot traffic can create such erratic and unpredictable changes, it distorts metrics like the average time a person spends on a product page. We often end up with a blurry view of true user interaction, highlighting the need for more complex analytical approaches. If we can't understand what real users are doing, it makes it harder to design better marketing campaigns.
The rise of AI-generated images has introduced new difficulties in separating real users from bots. Bots can interact with AI-created images in a way that is nearly identical to a human, making it harder to use traditional methods to detect them. This adds another layer of complexity when we're trying to measure how interested people are in product visuals.
It's been noticed that industries like fashion are especially vulnerable to bot activity, with estimates suggesting that a big chunk – over 70% – of the reported activity might be from bots. This means that marketing efforts could be significantly skewed and companies could make poor decisions about their marketing campaigns.
Dealing with bot traffic forces businesses to put more money into solutions that can detect and remove bot traffic. This adds unexpected expenses to marketing budgets, making financial planning more complex and difficult.
In conclusion, understanding and managing the effects of bot traffic on ecommerce analytics is essential for the future of the industry. It’s crucial to continually develop methods that can filter out this noise, allowing marketers to accurately interpret customer behavior and make effective decisions.
Create photorealistic images of your products in any environment without expensive photo shoots! (Get started for free)
More Posts from lionvaplus.com: