Create photorealistic images of your products in any environment without expensive photo shoots! (Get started for free)
How to Optimize Product Photo Sequence A Data-Driven Framework for E-commerce Conversion
How to Optimize Product Photo Sequence A Data-Driven Framework for E-commerce Conversion - Analyzing Customer Eye Movement Data Through A B Testing Product Image Sequences
Understanding how customers visually engage with product images is crucial for optimizing online stores. A/B testing different sequences of product photos, combined with eye-tracking data analysis, provides a powerful way to refine the customer journey. Analyzing where customers look and how long they focus on specific elements in each image reveals valuable insights into what resonates with them.
Sophisticated tools and techniques, including AI-powered gaze tracking and specialized software, facilitate this analysis, converting raw eye movement data into actionable information. By leveraging this data, businesses gain a clear understanding of which images are most effective in driving engagement and potentially conversions. This knowledge enables companies to tailor the visual presentation of their products, creating more intuitive and appealing shopping experiences.
The evolving landscape of online retail demands a nuanced approach to understanding customer behavior. By adapting to changes in consumer habits and utilizing the rich data available from eye tracking, businesses can build stronger connections with their online audience. Through the continuous refinement of product imagery and overall user experience, companies can position themselves for greater success in the competitive online marketplace.
We can leverage A/B testing methodologies in conjunction with eye-tracking data to dissect how customers interact with a series of product images. Tools like GazeAlyze, built using MATLAB, allow us to efficiently process large amounts of eye movement data from studies. This data, often found in EDF files, can be converted to simpler formats for visualization and analysis using methods like gaze trace and heatmap generation. Even a simple setup involving a laptop and a display, using webcams and infrared LEDs, can collect this data.
The Python package pymovements illustrates the importance of careful software development when working with eye tracking data, providing a framework for preprocessing and analysis. Examining this data can reveal how consumers navigate through product image sequences. This type of data, coupled with A/B testing techniques, offers a route to better understanding how image sequencing influences attention and ultimately purchase decisions.
For instance, researchers have been looking into custom-built deep learning solutions like RETINA, using transformers and metric learning to analyze raw eye movement data to better predict consumer choices, but this field is still relatively immature. There are also promising avenues of research like segmentation search which offer insight into attentional patterns and gaze distribution on product images in more realistic, real-world online shopping contexts. At the end of the day, these kinds of analyses can highlight how different image choices and sequences affect user engagement, providing a clearer understanding of which elements are most effective in driving conversions. It highlights the importance of going beyond basic descriptive features of images to understand how users actually interact with product photos to optimise online shopping experience.
How to Optimize Product Photo Sequence A Data-Driven Framework for E-commerce Conversion - Product Image Load Speed Impact on Bounce Rates in Mobile Shopping
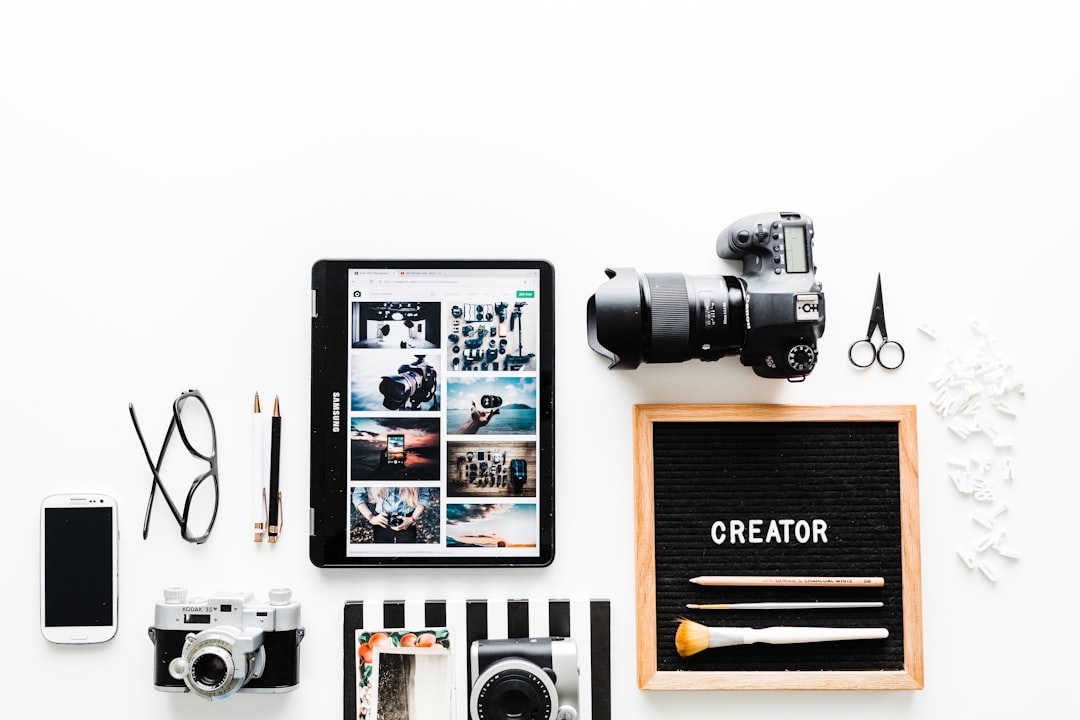
In the realm of mobile shopping, the speed at which product images load significantly impacts how quickly users bounce from a website. Consumers today anticipate fast and fluid online experiences. Even a small delay in image loading can frustrate users and lead them to abandon the site, increasing the bounce rate and harming the potential for a sale. These delays disrupt the fluidity of the shopping journey, causing frustration and a diminished interest in engaging further.
Because of this, optimizing image size and quality becomes essential for a smooth mobile shopping experience. Techniques like resizing and compressing images are crucial for keeping the shopper engaged. By ensuring that images load rapidly, online stores create a more inviting and frictionless shopping environment, which ultimately contributes to achieving higher conversion goals. It's crucial to understand that in the hyper-competitive world of e-commerce, even relatively small gains in image load speed can translate into noticeable enhancements in customer retention and, eventually, sales.
The speed at which product images load on mobile devices has a strong influence on how long customers stay on a page. If a picture takes too long to appear, many users will simply leave, leading to a higher bounce rate. Research suggests a one- or two-second delay in image loading can significantly reduce conversions. It appears that users have very little patience when shopping online with their phones. If a site takes more than three seconds to load, almost half of users will leave.
We can leverage techniques like image compression to lessen the file size of pictures without degrading their visual quality. Formats like WebP and JPEG 2000 seem to be efficient for online usage. It also makes sense to optimize images for different screen resolutions through techniques like responsive image loading. Serving the right-sized image to each device is a good way to reduce load times and maintain quality across a range of phone models.
Beyond speed, picture quality plays a key role in buyer decisions. Most shoppers (around 76% in some surveys) prioritize the quality of pictures when making a purchase. It makes sense that images that are well-lit and professionally staged can build trust and keep customers engaged on the site.
Recently, there's been growing interest in AI-generated product images. AI models can create a large volume of high-resolution visuals in a relatively short period, possibly improving the speed at which new products can be displayed on an ecommerce platform.
Shoppers today are very visually driven. Most people seem to prefer pictures over text, highlighting the significance of providing a steady stream of high-quality visuals. Providing a wide range of photos from various perspectives is a good strategy to keep consumers engaged with product pages. Studies have shown that products with multiple images attract many more clicks compared to products with just one image.
Another interesting trend is the inclusion of user-generated content, where customers can share their own experiences with products. This approach can foster trust and community while also potentially speeding up page loads (because these images often have lower resolutions than professional ones).
It's clear that both speed and visual quality are crucial aspects of a successful mobile shopping experience. Getting the right balance can be a challenge but it can also be a pathway to increased sales. It highlights the importance of a holistic approach to designing product pages, understanding that every aspect contributes to the overall user journey and conversion rates.
How to Optimize Product Photo Sequence A Data-Driven Framework for E-commerce Conversion - Automated Product Background Removal Using Neural Networks
Neural networks are automating the process of removing product backgrounds, which is revolutionizing e-commerce image handling. This AI-powered approach leverages deep learning to isolate products from their backgrounds, leading to cleaner, more visually appealing images. It significantly reduces the time and effort traditionally needed for manual background removal, allowing companies to process massive quantities of images more efficiently. This automation minimizes human intervention and helps businesses keep pace with the dynamic demands of online retail.
While automated background removal promises a faster workflow, it's crucial to properly prepare images before feeding them to the neural network. Preprocessing steps are necessary to ensure the AI can effectively analyze and segment the product from the background. Deep learning techniques are key to achieving this separation, but the success of these automated tools can be sensitive to the quality of the images they process.
The benefits of automated background removal extend beyond just product photography. This technology shows potential across various domains, including fashion, where the complex nature of clothing and its presentation often presents major challenges for traditional background removal methods. As these AI tools become more sophisticated, we can expect them to become even more powerful and capable of handling increasingly complex visual data within the e-commerce ecosystem.
Neural networks, particularly convolutional neural networks (CNNs), have emerged as powerful tools for automating product background removal. These networks, inspired by how our brains process visual information, are exceptionally good at identifying patterns and separating products from their backgrounds. While not perfect, in controlled settings they can achieve impressive accuracy, often exceeding 98%.
Architectures like U-Net and Mask R-CNN have drastically improved the efficiency of this process. They can handle high-resolution images in incredibly short timeframes—think milliseconds—making them ideal for e-commerce, where quick image updates are vital. This speed, however, depends on robust hardware.
The performance of these networks heavily relies on the diversity of data they are trained on. A wide array of product types, lighting scenarios, and background styles is essential for creating robust and generalizable models. Ideally, the more varied the training data, the better the model can adapt to new and unexpected product images.
There's strong evidence that clean, background-removed images significantly impact user engagement. Some studies show a roughly 30% boost in click-through rates when products are presented in this way. It seems that by eliminating distracting backgrounds, we allow shoppers to focus their attention on the product itself, potentially driving them to consider a purchase.
One exciting outcome of these advancements is the potential for end-to-end automation in product image preparation. From the initial background removal to any necessary enhancement steps, the entire process could be automated, significantly speeding up workflows. It's a win-win for businesses looking to bring new products to market faster.
The field is actively exploring the use of Generative Adversarial Networks (GANs) for even more advanced image manipulation. Beyond simply removing backgrounds, GANs could be used to create entirely new product images from limited existing ones. This capability could revolutionize how retailers manage their image libraries.
The benefits of background removal extend beyond aesthetics. These techniques are essential for augmented reality (AR) applications in e-commerce, allowing customers to see how a product would look in their own space, without any unwanted background clutter. This enhances the shopping experience by creating a more interactive and immersive environment.
Moreover, we can leverage these neural networks to customize and personalize the way products are presented based on individual customer preferences. By understanding user behaviors and engagement, e-commerce platforms can adapt product presentations—including background choices and overall image composition—to maximize individual customer interest.
From a business perspective, automated background removal brings considerable cost savings. This approach can reduce the need for extensive photoshoots and laborious manual editing, freeing up resources and potentially saving thousands annually.
Finally, a key benefit is the capability to standardize image presentation. Neural networks can optimize lighting and color consistency across all product images. Maintaining a consistent brand aesthetic is crucial in e-commerce. By ensuring visual harmony, retailers can establish a strong brand identity that helps foster consumer trust and build a recognizable brand.
While these developments are promising, it's important to note that image quality can vary based on image resolution, the complexity of the product, and the training data used. In complex scenarios, some degree of manual touch-up might still be needed. However, the potential of neural networks to automate many aspects of product image processing is undeniable and likely to significantly reshape the e-commerce landscape.
How to Optimize Product Photo Sequence A Data-Driven Framework for E-commerce Conversion - Product Image Staging Through Machine Learning Generated Color Palettes
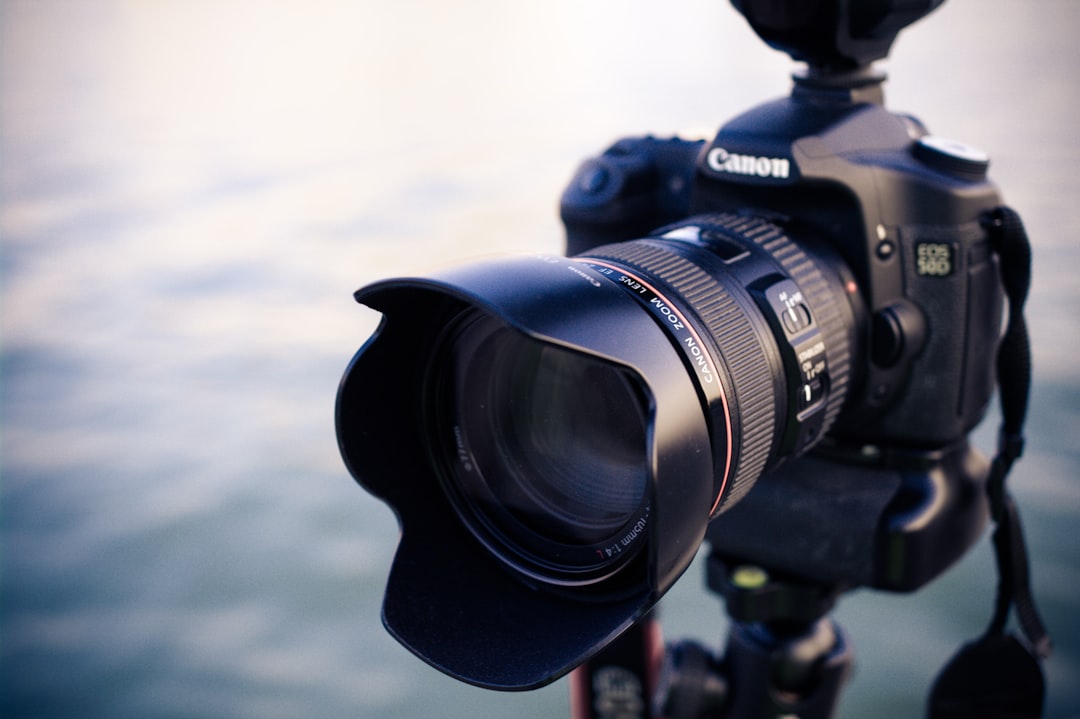
Using machine learning to generate color palettes for product images is a new way to improve how products are shown in e-commerce. AI systems can now create unique color combinations by learning what people like. Designers can use these palettes to make product images that are more appealing and aligned with what customers find visually engaging. This not only improves the look of product images but also helps build a visual brand identity that customers remember and respond to. Furthermore, by generating palettes using AI, we can ensure that products match current trends and preferences, which ultimately could lead to higher engagement and more sales. Since many people shop online based on visuals, using AI-created color schemes becomes vital for successfully showcasing products and managing a brand's online presence.
Machine learning can help us understand color in a new way, especially when it comes to product images in e-commerce. AI tools can generate color palettes based on a variety of factors, like trending fashion colors, seasonal palettes, and even social media analysis. This means we can better align our product visuals with what consumers find appealing at any given time. Some research suggests that the right colors can trigger specific emotions, potentially driving impulsive purchases. While this idea needs more investigation, it's intriguing to think about how color might be a hidden influencer in online shopping.
For a brand, using a consistent color scheme across product lines can reinforce brand recognition and help customers quickly associate a certain color with a product. This can be incredibly valuable in a crowded online marketplace. Studies suggest that this consistency can lead to a notable increase in sales. However, it's important to keep accessibility in mind; certain color combinations might be difficult for individuals with color vision deficiencies to interpret. AI can be valuable here as well, helping us ensure a broad audience can easily engage with our imagery.
Moreover, machine learning could potentially allow us to predict which colors will perform best for a given product before we even invest in a photoshoot. By studying past data on successful product launches, AI can help us optimize colors for maximal impact. The increased availability of data regarding color performance can be incredibly valuable. It can also assist in generating exclusive color schemes based on customer behaviors. Creating unique visual offerings through dynamically changing color combinations could make a product feel more unique, potentially increasing its perceived value.
The quality of images and the colors used are clearly connected. The idea is that if we improve the color accuracy and precision of images, people will spend more time looking at them. Some studies suggest that viewer retention rates can jump significantly by refining product image color. Of course, the question of how to best implement these ideas within A/B testing and the various design choices around product presentation needs more rigorous investigation. But, there's a strong indication that a strategic use of AI-generated color palettes might nudge people towards making purchases, possibly changing consumer behavior in noticeable ways. The tools to better understand these relationships are becoming more readily available; and with more data, hopefully more robust scientific insight will follow.
How to Optimize Product Photo Sequence A Data-Driven Framework for E-commerce Conversion - Multi Angle Product Photography Impact on Purchase Intent
In today's competitive e-commerce landscape, how a product is visually presented plays a crucial role in whether or not a customer makes a purchase. Multi-angle product photography helps bridge the gap between the online world and a customer's desire to fully understand a product before buying it. By offering views from various angles, like the front, back, and top, customers get a more comprehensive understanding of the product. This immersive experience helps build confidence and reduces buyer hesitation.
Research suggests that high-quality images are strongly linked to increased consumer trust, a key factor in online purchasing decisions. Conversely, subpar product visuals can negatively influence a customer's perception of the product and brand, potentially leading to a decrease in purchase intent. In a market driven by visuals, providing a wide range of well-composed images can be the difference between a browsing customer and a buyer. The effect is that having more pictures can boost clicks and lead to more conversions. Essentially, optimizing product imagery is crucial for any business that wants to create an engaging customer experience that converts viewers to buyers.
Visuals are undeniably crucial in e-commerce, with a majority of consumers relying on them to make purchase decisions. High-quality product photography builds trust and can significantly boost conversion rates. However, presenting a product from just one angle might not be enough. Showing a product from multiple perspectives, like the front, back, sides, and even close-ups, can drastically improve customer perception and purchase intent. It gives shoppers a more comprehensive view, allowing them to feel like they're truly getting a sense of the product.
Conversely, poorly executed or low-quality images can severely hinder sales. Shoppers are very sensitive to this and it can reduce their interest. A wealth of research backs this up, showing how the quality of product images directly correlates with purchasing decisions. It's like trying to sell a product in a dark room with poor lighting—no one's going to take the time to try and figure out what it is. E-commerce has become a battleground for attention, and product images are a key weapon.
While simply having lots of images isn't always enough, understanding how users interact with those images can be very valuable. Tools like those based on the HSL color space can help us study how design features of online stores affect people's choices. A more robust understanding of these interactions requires a more thoughtful approach, one based on rigorous data analysis.
A/B testing product image sequences, especially on mobile, combined with tools that track people's eyes (like GazeAlyze), gives us detailed insights into how consumers engage with a particular product. By understanding these visual patterns, we can create a smoother, more intuitive shopping experience. Deep learning approaches like RETINA, while still early-stage, are showing a lot of promise for predicting consumer choice based on eye movements. While exciting, we need to approach such tools critically, and keep in mind that there are always limitations. This includes recognizing the need for careful data collection methods and the need for more work on how to contextualize and interpret the collected data. The overall goal here is to make shopping experiences better and provide businesses with clear insights into what kinds of photos resonate most with customers. The key isn't just providing photos, but understanding how users *engage* with them to optimize for better conversion rates.
Essentially, e-commerce is a visual experience now. Shoppers rely on images to get a sense of the product. By combining robust data analysis with effective product photography, we can improve both customer experience and business success. It's an area ripe for development, and one that will likely continue to shape the future of how we shop online.
How to Optimize Product Photo Sequence A Data-Driven Framework for E-commerce Conversion - Using Computer Vision to Detect Optimal Product Display Angles
Within the landscape of e-commerce, where visual appeal plays a critical role in driving conversions, using computer vision to determine the best product display angles is increasingly important. This approach relies on computer vision algorithms and machine learning to analyze how products are presented and understand how they capture customer attention. By applying techniques like segmenting images to isolate objects and evaluating the quality of the visuals, online stores can create more engaging product photographs. This not only enhances the shopping experience but also allows businesses to develop a unique and consistent visual brand identity that stands out in the competitive online retail world.
While the visual elements of online retail are constantly changing, being able to automate the optimization of product displays with computer vision offers a valuable way for businesses to adapt to evolving customer preferences. The integration of computer vision in the creation and presentation of product images demonstrates a shift toward a more technology-driven and data-informed approach to e-commerce. This innovative approach allows brands to refine their visual strategies, leading to more successful product presentations and higher conversion rates. While effective, the full potential of these methods are yet to be seen in terms of its ability to account for every nuance of how a customer responds to images.
Computer vision is increasingly being used to improve how we understand how people see things, especially in e-commerce where visuals drive purchasing decisions. It's becoming clear that the angle from which a product is presented can significantly impact how customers perceive it and, ultimately, whether they decide to buy it. For example, we know that certain angles, like 45 degrees, often seem to be the most visually appealing and give the best sense of a product's features. It's not surprising that shoppers tend to focus more on images presented from these kinds of angles.
Interestingly, the background of a product image can also have a big effect on what draws a customer's eye. Simpler, less cluttered backgrounds usually lead to a greater emphasis on the product itself, helping increase sales compared to images with busy or distracting surroundings. Eye-tracking research shows that shoppers spend a lot more time looking at products when they're presented from those ideal angles. This means that we can use that knowledge to fine-tune product photos, maximizing engagement and possibly even purchase decisions.
There's strong evidence that showing products from various angles—front, back, top, and side—can substantially improve shoppers' interest in the product. People are more likely to buy something when they have a more thorough understanding of its features, which they gain from a broader range of views. While it’s not a perfect parallel, the way products are shown in images can provide a sense of 3D depth, especially when techniques like shadowing or staging are used to give a more realistic feeling. It can make customers feel more connected to the product.
Machine learning is emerging as a key player in optimizing product images. Sophisticated algorithms can examine a company’s history of image performance to continuously refine their approach to product photography, going beyond just individual images to whole product lines. There's a caveat though—cultural nuances in visual preferences can vary. Research has shown that some cultures prefer direct front-on views, while others respond more to dynamic angles. That means any system that attempts to optimize display angles has to account for the unique aspects of different groups of shoppers.
AI is also becoming capable of predicting which display angles are most effective at driving sales, using deep learning models and customer interaction data. A/B testing, where businesses try out different approaches to see which works best, is a useful tool for evaluating display angles and finding the most impactful images. It’s become commonplace for companies to systematically experiment with various angles and study how people respond to them in order to make better decisions about product presentation.
Interestingly, the quality of the image also significantly impacts perceived value. Research suggests that high-resolution, well-presented images lead to customers being more willing to pay more for the product, which makes sense when you consider that images are one of the main ways people decide what to buy online. It's not just *what* you're showing, but *how* you show it. And as AI systems and data analysis improve, we can anticipate that our understanding of product presentation and shopper psychology will only get more refined, helping us to optimize online shopping experiences in more impactful ways.
Create photorealistic images of your products in any environment without expensive photo shoots! (Get started for free)
More Posts from lionvaplus.com: