Create photorealistic images of your products in any environment without expensive photo shoots! (Get started for free)
How AI Image Generation Captures the Ephemeral Nature of Product Photography A Technical Analysis of Transience in Digital Commerce
How AI Image Generation Captures the Ephemeral Nature of Product Photography A Technical Analysis of Transience in Digital Commerce - Breaking Down Adobe Firely AI Image Generator Through Real Product Staging Examples
Adobe Firefly, with its advanced AI image generation capabilities, is rapidly changing how product imagery is created, especially for online businesses. Firefly 3, the latest version, significantly improves the quality and realism of generated images by refining aspects like detail, lighting, and color—all vital for creating visually engaging product shots. The sheer number of images produced—billions—since its initial release demonstrates Firefly's influence in e-commerce, particularly in portraying the dynamic nature of digital products. While Firefly strives for ethical AI development and accessibility for all creators, we should consider the wider implications of this technology on how consumers view products and how diverse perspectives are represented. Firefly's ability to empower creators with simple text prompts not only democratizes the creative process but also shifts the traditional methods of product staging, highlighting the growing convergence of artificial intelligence and artistic expression in the evolving landscape of online shopping.
Adobe Firefly, while being a powerful generative AI tool, goes beyond simply creating images from text prompts. Its core algorithms are trained on a vast collection of product images, effectively learning the nuances of visual design, including composition, lighting, and color schemes. This understanding is crucial for generating convincingly staged product shots.
The way Firefly generates images is through sophisticated mathematical models that meticulously recreate how light behaves in real-world scenarios. The resulting imagery, with its accurate shadows and reflections, often appears indistinguishable from photos taken with a traditional camera, especially for those not well-versed in image analysis.
Firefly’s capacity to adapt to various product types is impressive. It has been trained on a massive dataset covering a wide array of products, allowing it to adjust its staging style for different categories, whether it's showcasing the latest tech gadgets or presenting high-fashion apparel.
Users can tailor the AI’s output using specific input parameters, like desired mood, textures, or background settings. This level of control allows for customized results, helping to emphasize the product's unique features in a visually compelling manner.
The impact of carefully staged environments on consumer engagement has been shown in various studies. Apparently, AI-generated images with contextually relevant backdrops can significantly improve consumer interaction. This suggests that tools like Firefly can play a vital role in designing compelling visual experiences for shoppers.
It is important to acknowledge the potential for AI to reinforce any biases present in the training datasets. To counter this, it's critical to carefully curate and diversify these datasets, thereby ensuring that product images are fair and unbiased representations across different product categories.
One of the key advantages of using AI in product imagery is the potential to streamline the entire process. Some tasks that used to require hours or days can now be accomplished in a matter of minutes. This accelerated workflow can be a substantial benefit in the demanding e-commerce sector.
Moreover, AI-generated images open doors to rapid experimentation with various staging concepts. Marketers can quickly test numerous variations without the limitations associated with conventional photo shoots. This capacity can lead to valuable insights, particularly during product launches.
The adaptable nature of Firefly's neural networks allows brands to foster a unique visual identity across their product line and marketing channels, providing a cohesive and consistent aesthetic.
When examining the impact of Firefly on e-commerce performance, there is evidence that dynamic or seasonal backgrounds within product imagery can increase conversion rates. This demonstrates how strategically implementing AI within product photography can positively affect business outcomes.
How AI Image Generation Captures the Ephemeral Nature of Product Photography A Technical Analysis of Transience in Digital Commerce - Neural Networks Interpretation of Product Lighting From Single Reference Images
AI image generation tools are increasingly leveraging neural networks to interpret and manipulate product lighting. This capability stems from advances in deep learning models that can estimate and recreate illumination effects from a single reference image, significantly improving product image quality. These models, often based on architectures like ResNet, take input from various sources, including refined shadow images and illumination buffers, to generate realistic relit product shots. The training process frequently involves synthetic datasets, providing these models with a foundation to handle complex lighting scenarios. This capacity for sophisticated lighting control highlights the potential of AI to recreate the ephemeral aspects of product photography—from subtle highlights to dramatic shadow play—all of which contribute to a product's perceived aesthetic and ultimately, influence consumer decisions. While promising, this technology must address inherent challenges in image analysis, including noise reduction and image segmentation, to deliver consistently high-quality results. The ability to generate dynamic lighting effects digitally reinforces how AI can reshape the visual presentation of products online, with significant ramifications for consumer engagement in digital commerce.
Current AI image generation tools like Firefly are increasingly leveraging neural networks to tackle the challenge of recreating realistic product lighting from limited input, often just a single reference image. These networks, frequently built on architectures like ResNet, are trained on vast synthetic datasets encompassing a range of photorealistic scenes. This approach enables them to effectively simulate complex lighting interactions, from subtle shadows to vibrant highlights, resulting in images that can convincingly mimic real-world photography.
The use of neural networks allows for a level of efficiency previously unseen in product photography. A single reference image can serve as a starting point for generating images under diverse lighting conditions, thus significantly reducing the need for numerous, time-consuming photo shoots. This flexibility stems from the network's ability to learn and apply lighting principles gleaned from its training data. Further, these networks can adapt their lighting interpretations based on contextual clues present in the reference image. For instance, if a product image suggests an indoor setting with a warm light source, the network might automatically adjust shadows and highlights to align with this inferred environment.
However, there's an interesting aspect to how these networks achieve a realistic aesthetic. They often prioritize a perceptual understanding of lighting rather than pixel-perfect accuracy. This is achieved through techniques like perceptual loss functions, which guide the network toward generating images that look visually convincing to human eyes, even if they don't precisely match the physical properties of light in a given scenario. This approach is crucial for making AI-generated product imagery appear naturally appealing to consumers.
The concept of a "latent space" is central to how neural networks manipulate lighting characteristics. This latent space essentially encodes different visual styles and features, including the subtleties of brightness and contrast. Marketers can leverage this latent space to fine-tune the output, creating tailored product images that better resonate with specific target demographics.
Furthermore, the neural networks' capacity to learn and synthesize realistic textures is remarkable. The training process exposes them to a broad range of product types and materials, leading to a nuanced understanding of how light interacts with diverse surface properties. This ability to synthesize complex textures and reflections makes the generated images appear more authentic and detailed.
While the computational demands of these networks were once prohibitive, advancements in deep learning have drastically reduced the time it takes to render a high-quality product image. This is a significant advantage for e-commerce, enabling brands to update product imagery more frequently and react faster to market trends.
However, there's a growing area of research focused on imbuing neural networks with a deeper semantic understanding of images. This would mean the network wouldn't just be capable of rendering lighting effects but would also be able to grasp the emotional and psychological impact of different lighting setups. Such an advancement would allow for a more sophisticated and targeted use of AI in product photography, allowing brands to precisely tailor images to elicit specific emotional responses from their target audience. It's an exciting frontier that promises to further revolutionize the field of product imagery.
How AI Image Generation Captures the Ephemeral Nature of Product Photography A Technical Analysis of Transience in Digital Commerce - The Technical Framework Behind Automated Background Removal in AI Commerce Photography
AI-powered background removal in e-commerce product photography leverages sophisticated machine learning techniques to streamline image editing. Deep learning models and neural networks are central to these systems, enabling the automated separation of products from their backgrounds. This automation significantly reduces the manual effort previously required for such edits, allowing businesses to manage vast quantities of product images with greater efficiency.
The ability to rapidly generate and modify backgrounds, alongside the control of lighting effects, allows for greater flexibility and responsiveness in the fast-paced world of e-commerce. By adapting the image presentation to target audiences and trends, businesses can enhance the appeal of their products and improve the overall customer experience. The evolution of these technologies is crucial for creating visually impactful product imagery, which is essential for attracting consumers in the dynamic and constantly changing realm of online shopping. There are, however, limitations to this technology and issues with bias that need careful attention in order to ensure a more equitable approach to visual representations in digital commerce. While the promise of creating highly customized, aesthetically appealing, and efficient imagery is significant, careful consideration is needed to ensure AI tools are used responsibly and equitably.
The technical underpinnings of automated background removal in AI-driven e-commerce photography are quite intricate. It relies heavily on sophisticated image segmentation algorithms that skillfully isolate product images from their backgrounds. These algorithms, often built upon deep learning architectures like convolutional neural networks (CNNs), are meticulously trained on diverse datasets. This training allows the AI systems to differentiate fine details such as edges, shadows, and surface textures with remarkable precision, essentially 'teaching' them to recognize what constitutes the product and what constitutes the background.
The field is characterized by a variety of AI models, each with its own strengths and weaknesses. Some models focus on enhancing visual clarity, while others emphasize swift processing speeds. This variety empowers e-commerce businesses to choose tools that align with their specific operational needs without compromising image quality. The best approach isn't always the fastest or most precise; rather, it depends on the context of the business.
The development of these algorithms has enabled real-time background removal in many cases. Online retailers can now dynamically adjust product displays in response to customer interactions, fostering a more engaging shopping experience. Imagine a scenario where a shopper can instantly change the background of a product to better suit their personal taste— this is a testament to the dynamic nature of AI-powered background removal.
Prior to the advent of these AI techniques, background removal was a laborious process involving considerable manual editing by skilled image professionals. This evolution towards automation not only reduces labor costs but also minimizes the inherent human error that can be present in manual editing. As a result, AI-driven solutions ensure greater consistency in product imagery across a brand's online platform.
The efficacy of AI-based background removal is often assessed using metrics such as Intersection over Union (IoU). This metric quantifies how well the AI separates the product from its surrounding environment. High IoU scores indicate a high degree of accuracy in the segmentation process, a crucial factor for maintaining the professional and visually appealing appearance of e-commerce product images.
It's also crucial to understand that the diversity of the training data significantly influences the algorithm's performance. Datasets that contain a wide variety of products in different environments enable the AI to generalize better. In other words, the more varied the training data, the more likely the AI will be able to accurately segment backgrounds for a broad range of product categories.
Some AI-powered background removal tools offer interactive features, allowing users to fine-tune the results manually. This level of user interactivity empowers e-commerce businesses to personalize their product images further, fostering greater customer confidence and enhancing overall shopping experiences. This user control can be crucial for businesses concerned about specific branding or aesthetic requirements.
The ability to automate background removal empowers brands to create a consistent visual style across their product lines. This standardization of backgrounds helps establish a recognizable brand identity, a crucial element for businesses competing in a crowded e-commerce landscape. Creating a unique aesthetic helps shoppers more easily find and recall your product when shopping online.
Several studies have shown that high-quality product images with effectively removed backgrounds can significantly boost conversion rates. Products displayed against clean, neutral backgrounds tend to capture consumer attention more efficiently, resulting in a higher probability of sales. This highlights the direct link between effective background removal and positive business outcomes in the e-commerce space.
As AI continues to evolve, there is exciting research into the use of advanced techniques like Generative Adversarial Networks (GANs) for refining background removal procedures. With GANs, the AI could potentially generate entirely new backgrounds that harmonize with the characteristics of the product, opening up a realm of possibilities for creative product presentations. The possibilities presented by AI are only just starting to be explored, promising further innovation in the future.
How AI Image Generation Captures the Ephemeral Nature of Product Photography A Technical Analysis of Transience in Digital Commerce - Understanding Computer Vision Processing for Product Detail Enhancement
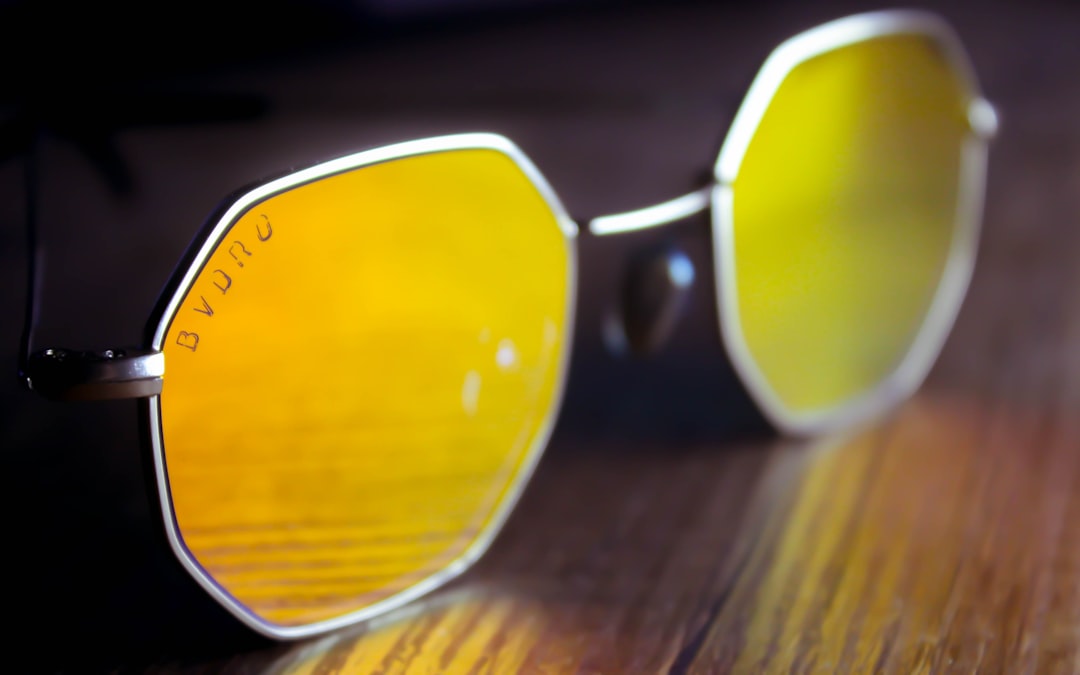
Understanding how computers "see" and interpret product details is crucial for enhancing e-commerce visuals. Computer vision relies on sophisticated image processing techniques, like analyzing pixel patterns using Local Binary Patterns, and deep learning, which can automatically extract features from large datasets of images. These capabilities allow for more accurate texture recognition and finer detail enhancements in product imagery. The ability to capture and showcase these intricate details in a visually appealing way is vital for engaging online shoppers. As AI progresses, computer vision's role in automating and improving product detail enhancement will only grow, transforming the ways brands present their goods online. This shift towards greater efficiency and visual expression in e-commerce underscores the ongoing interplay between technology and artistry in capturing the dynamic essence of product photography within the ever-changing digital landscape of commerce. While this offers a tremendous opportunity, we must be mindful of the potential for bias and inequities in how these AI systems are trained.
Within the realm of AI-powered e-commerce imagery, computer vision processing plays a crucial role in enhancing product details and creating engaging visuals. A core aspect is the use of neural networks, which are not simply striving for pixel-perfect accuracy but are designed to create images that are visually compelling to human observers. These networks often incorporate perceptual loss functions, a clever way of prioritizing how we perceive light and shadow over absolute physical accuracy, making the output appear more naturally attractive.
The ability to adapt in real-time is a striking feature of these AI systems. For instance, features like automated background removal can be dynamically adjusted based on customer interactions on an e-commerce website. This creates more engaging and tailored experiences, enabling brands to stay responsive to trends and individual consumer preferences. Behind these capabilities lies a constantly learning framework. These AI models are continuously fine-tuning their understanding through a feedback loop, improving their accuracy with every new batch of data. This continuous learning aspect reduces the reliance on constant manual adjustments and allows for significant increases in efficiency.
However, the quality of results significantly depends on the quantity and diversity of the datasets these models are trained on. A diverse and comprehensive dataset is key to reducing biases and improving the accuracy of algorithms, particularly those that focus on tasks like segmenting product images from their backgrounds. Here, a metric called Intersection over Union (IoU) provides a useful way to objectively measure how well the AI is doing this task. High IoU scores indicate that the product is successfully separated from the background, a critical factor for delivering high-quality, professional looking product images.
Pushing the boundaries of background removal are sophisticated techniques that leverage Generative Adversarial Networks (GANs). These networks hold the potential to create entirely new and unique background scenes that seamlessly complement the product itself, allowing for more creative and flexible product presentation. The shift towards automated processes is revolutionizing product image creation, where tasks that once required substantial time and effort from skilled human operators can now be handled in a fraction of the time. AI is significantly streamlining image production, providing a major advantage for businesses competing in the e-commerce landscape.
Furthermore, AI models are now capable of simulating layered lighting effects with impressive detail. This ability to capture the intricacies of real-world light interactions, including reflections and nuanced shadow play, gives AI-generated images a more authentic aesthetic. This detailed simulation is important in presenting products as attractively as possible. Moreover, the capacity for rapid A/B testing within AI-driven platforms is enabling marketers to refine their image selection through experimentation. This process allows brands to understand which visuals are most effective at driving sales and boosting customer retention.
There is also fascinating ongoing research into equipping AI models with a deeper semantic understanding of images. This line of research aims to enable AI to recognize how different visual cues and lighting can evoke distinct emotional responses. The goal is to allow brands to not only showcase products beautifully but also create images that resonate with consumers on a deeper level, tailoring visuals that tap into our emotional connection to products. This is a powerful emerging capability that could further redefine the landscape of product imagery within e-commerce.
How AI Image Generation Captures the Ephemeral Nature of Product Photography A Technical Analysis of Transience in Digital Commerce - AI Image Quality Loss Assessment Through Real E-commerce Case Studies
The use of AI for creating product images in e-commerce is rapidly expanding, offering a way to quickly generate high-quality visuals. However, a key issue arises: how do we ensure the quality of these AI-generated images remains high enough to be compelling to customers? While AI can produce impressive results, it's becoming clear that these generated images can sometimes suffer from unique distortions and a reduction in overall quality when compared to traditional product photography. These imperfections can negatively impact how customers perceive products, leading to decreased engagement and potentially even lost sales.
Because of this, there's a growing need for methods to accurately measure and evaluate the quality of AI-generated product images. Currently, evaluating AI-generated image quality often involves subjective methods like crowdsourcing, which can lead to varying results depending on the specific AI tool used and the prompt given to it. It's also becoming evident that there's a shifting understanding of what constitutes "good" image quality in the digital world, largely driven by the evolving use of AI tools. Ultimately, to succeed, brands need to ensure that their AI-generated product images are truly meeting the evolving expectations of their customers, and to do this, more objective and reliable quality assessment methods are urgently needed.
AI image generation, while offering remarkable speed and flexibility for e-commerce product photography, also introduces complexities in ensuring image quality meets the standards expected by online shoppers. One of the challenges is evaluating the output. Methods like Structural Similarity Index (SSIM) and Peak Signal-to-Noise Ratio (PSNR) provide a numerical way to gauge the quality of generated images by comparing them to real photographs. However, it's not just about numbers; it's also about how the images are perceived by human eyes. Modern AI is increasingly designed to mimic our visual system, paying attention to sharpness, color accuracy, and other visual cues that influence our judgments of quality. This approach is especially vital for products like clothes and accessories, where texture plays a significant role in attracting customers.
Interestingly, the quality of an AI-generated image can have a direct impact on how much customers trust a brand. Research suggests that higher-quality product visuals lead to a greater sense of confidence in the online retailer, which can translate into higher sales. The ability to create images tailored to specific customer groups is also a compelling aspect of AI-driven image generation. By leveraging consumer data, businesses can fine-tune images to resonate with specific demographic segments, using color variations or stylistic adjustments to create a stronger connection with their target audience. This capacity to adapt is further enhanced by the inherent learning capabilities of AI. These models continuously improve their output based on how customers interact with product images. This means that AI image generators are not static; they evolve over time to better meet our changing tastes and visual preferences.
Generative Adversarial Networks (GANs) provide another interesting angle for improving image quality. These networks can take lower-resolution or less detailed images and enhance them, often creating impressively realistic results. This can be valuable during marketing campaigns, where businesses want to quickly generate several versions of a product image to show off different aspects or features. Techniques like bicubic interpolation also play a role in optimizing image quality. This approach helps to smooth transitions and sharpen edges, resulting in sharper and more appealing product images. Even the way products are presented in a picture can be enhanced by AI. Automated tools can now analyze image composition and automatically fix issues like poorly aligned angles or incorrect lighting. This is valuable in ensuring a professional look without needing extensive manual edits.
Of course, the quality of these AI-driven image assessments depends on how the AI systems are trained in the first place. The training data must be diverse and representative of the vast range of products sold online and their accompanying visual characteristics. This step is important because if the data is skewed towards particular product types or lighting scenarios, the AI could develop biases and not perform equally well for all products. Having a well-rounded training dataset helps the AI to better understand variations in texture, light, and overall aesthetic qualities across a wide spectrum of goods. This also helps to mitigate potential bias, promoting more equitable representation of different product types within the realm of online commerce. The use of diverse datasets is becoming increasingly important as AI is adopted for a broader range of tasks related to visual representations.
How AI Image Generation Captures the Ephemeral Nature of Product Photography A Technical Analysis of Transience in Digital Commerce - Cloud Based vs Local Processing in Commercial AI Image Generation
When it comes to AI-generated images for e-commerce, whether to use cloud-based or local processing is a big decision. Cloud services have a major advantage with their processing power, which leads to quicker image generation and greater adaptability because of their scalable nature. Businesses looking for speed and top-notch image quality often find solutions like DALLE or Imagen appealing because of how quickly they can generate precisely what they need for boosting customer engagement online. On the flip side, local AI processing gives businesses more control and predictable expenses. This makes them attractive to those who are worried about their data's safety or need a system that works consistently even if the internet connection isn't perfect. As online shopping continues to evolve, choosing the right processing method is a key factor in a brand's ability to effectively present the often fleeting visual nature of products.
When it comes to using AI for generating product images in e-commerce, we have a choice between using cloud-based services or setting up our own local processing system. Each approach comes with its own set of advantages and disadvantages, which can significantly influence the effectiveness of product imagery for different e-commerce businesses.
For example, cloud services like those offered by OpenAI, with their impressive models like DALL-E 2, depend on powerful remote computers with specialized processors, like GPUs. This allows them to handle complex image generation tasks, something that can be challenging with the average computer you might find in a small business. But local systems, like Fooocus, let you have more direct control and speed because you are not limited by network speed.
One of the biggest concerns with cloud-based approaches is data privacy. When you send your product images to the cloud, it's essential to be aware of the security implications, especially if you're working with confidential information. A local system gives you better control over your data, keeping it all within your environment.
When we look at the bigger picture, cloud services often handle model updates seamlessly. They integrate new improvements as soon as they are available, which is helpful if you need to stay on the cutting edge. However, local setups can require more manual maintenance and updating of software and models, which can fall behind current capabilities.
Interestingly, local processing can offer a greater degree of flexibility for users who need highly specialized workflows. For example, it allows you to tailor the settings in a way that you might not be able to with cloud services. On the other hand, cloud-based services are highly scalable. This is particularly helpful if you have big spikes in demand during sales events where you need a lot of images quickly.
When it comes to money, the costs can be different. Cloud services are often pay-as-you-go, which can be beneficial for infrequent users. But if you are generating a large number of images regularly, investing in a local system might end up being a more cost-effective approach. Also, cloud processing relies on network connectivity, which can impact image quality in the case of unstable internet connections, whereas local processing can produce consistently good-quality images based on the consistent resources it uses.
Another consideration is how easy it is to integrate these solutions with your existing e-commerce platform. Cloud-based solutions might need some additional middleware or APIs, adding a layer of complexity that isn't present with local systems, where everything can be more tightly connected.
Finally, users who are new to cloud services might find there's a bit of a learning curve. Navigating licensing options, usage quotas, and service offerings can be challenging for those without a strong technical background, potentially creating a hurdle for small businesses.
In conclusion, the choice between cloud-based and local AI image generation ultimately depends on a variety of factors including cost, processing power requirements, speed needs, security, and the complexity of your e-commerce setup. By carefully considering these elements, businesses can leverage AI image generation effectively to create compelling visuals that help them stay competitive in the dynamic world of online shopping. And as these technologies continue to evolve, it will be exciting to see how the methods of generating and displaying product imagery are refined even further in the future.
Create photorealistic images of your products in any environment without expensive photo shoots! (Get started for free)
More Posts from lionvaplus.com: