Create photorealistic images of your products in any environment without expensive photo shoots! (Get started for free)
The Role of Content Agility in Enhancing Product Imagery Strategy
The Role of Content Agility in Enhancing Product Imagery Strategy - Adapting Product Imagery Quickly Leveraging Agile Tactics
Applying agile thinking to how e-commerce businesses handle product visuals has become critically important for keeping pace in rapidly changing online environments. Leveraging tactics focused on quick iteration and adaptation, particularly aided by capabilities like AI image generation or sophisticated digital staging, allows for the rapid creation and modification of product imagery. This capability means brands can respond far more dynamically to evolving customer interests and market currents. Beyond simply improving aesthetics, this adaptable approach facilitates seamless experimentation with different visual styles and permits fine-tuning images for diverse platforms and specific audience segments. However, the push for speed through increasing reliance on automation carries a notable downside: the potential erosion of authentic brand identity and the diminishing role of creative human insight. Success in this dynamic area hinges on effectively balancing the adoption of new visual technologies with the preservation of a brand's unique character.
Observations stemming from the practical application of agile methodologies to the challenge of rapidly evolving product imagery for online retail suggest several notable points as of late May 2025:
1. Current iterations of AI image generation systems demonstrate the technical capability to render numerous visual representations for a single item, depicting it in diverse settings or contexts with significant speed, often completing variations within half a minute. This automation potentially bypasses many traditional manual staging and photography bottlenecks, though ensuring artistic coherence and consistency across variations remains an active area of research and implementation.
2. Empirical findings from iterative A/B testing cycles focused on product imagery variations, conducted within short sprint-like timelines, have indicated measurable impacts on user interaction metrics, sometimes showing conversion lift figures cited in the vicinity of 20-30%. This highlights the potential for data-driven optimization loops to refine visual assets quickly, provided the experimental design and tracking are robust enough to isolate the image effect.
3. Advanced deep learning techniques integrated into the image generation or processing pipelines are reportedly capable of identifying and attempting automatic remediation of minor visual discrepancies – such as transient surface particles or subtle colour shifts – aiming for a level of visual uniformity that might traditionally require painstaking manual retouching work. The reliability and artistry of such automated corrections across a wide range of product types warrant ongoing evaluation.
4. Analyses comparing product performance metrics have frequently noted that items presented with more contextually relevant or "in-use" imagery tend to correlate with higher engagement or conversion rates than those displayed solely as isolated objects against stark backgrounds. While the exact magnitude of this effect can vary widely, figures suggesting an average performance improvement of around 15% for such dynamically presented images point towards a general user preference for visuals that aid in imagining product utility.
5. The application of generative adversarial networks (GANs) is pushing the boundaries of conventional product representation, enabling the synthesis of visuals that depart from photorealism, exploring abstract or stylized aesthetics. While technically feasible and intriguing for potential brand differentiation or targeting niche audiences, understanding consumer perception and ensuring these novel styles effectively communicate product attributes without causing confusion are critical considerations.
The Role of Content Agility in Enhancing Product Imagery Strategy - Integrating AI Image Generators into the Daily Visual Workflow in 2025
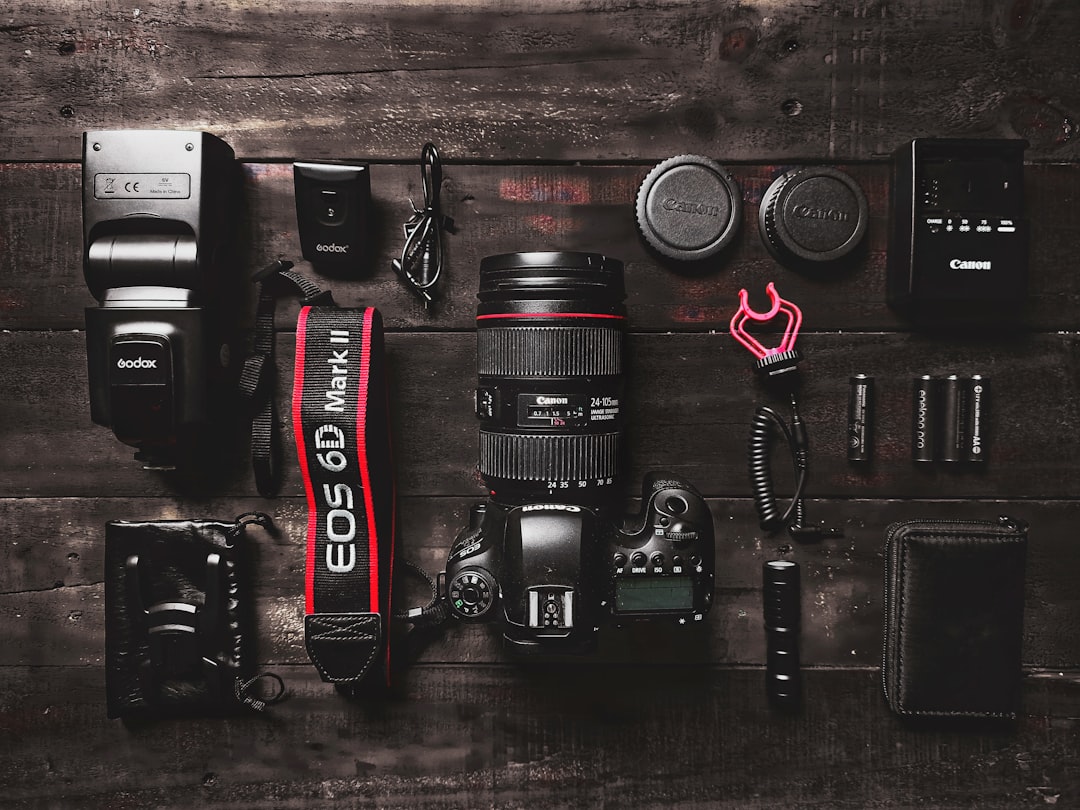
As of 2025, the integration of AI image generation tools into the daily processes of visual creation, particularly for digital platforms like e-commerce, is moving beyond experimental use cases towards being a practical component. These tools are becoming more intuitive and widely accessible, fundamentally changing who can create sophisticated visual content and how quickly it can be produced. This shift enables businesses to generate imagery with significantly reduced time and resource investment compared to traditional methods. Practically, it means workflows are adapting to allow creative teams to blend their design intent with AI-generated elements, using these systems to rapidly develop concepts, iterate on visual styles, or produce variations that previously would have been too time-consuming. However, the real challenge lies in ensuring these AI-assisted outputs genuinely serve the specific visual needs and maintain the unique quality associated with a brand, rather than defaulting to generic aesthetics, requiring a constant focus on directing the AI effectively and refining the results.
Observations stemming from the practical application of agile methodologies to the challenge of rapidly evolving product imagery for online retail suggest several notable points as of late May 2025:
1. Computational systems are being explored that correlate patterns found in accumulated textual feedback (like user reviews) with visual features of product imagery. The aim is to identify aspects that resonate positively or negatively and computationally guide future image generation or modification processes, theoretically addressing potential points of customer friction before they manifest widely, though the robustness of inferring subjective visual preferences from text remains a subject of investigation.
2. The deployment infrastructure for product visuals increasingly incorporates dynamic delivery mechanisms. Instead of a single static asset, these systems now frequently render or adapt image streams on the client-side or near-edge, tailoring resolution, compression artifacts, and occasionally detail levels in near real-time based on detected network conditions and display capabilities, aiming for perceived load speed and visual fidelity across highly variable user environments, though achieving optimal quality-bandwidth trade-offs is non-trivial.
3. Efforts to internationalize visual assets leverage AI-driven generation capabilities to programmatically adjust contextual elements within product scenes. This involves attempting to align visual cues (e.g., surrounding objects, implied settings) with perceived cultural norms or aesthetic preferences of specific geographic markets, intending to increase relevance and avoid potentially inadvertent cultural clashes or misinterpretations, though the accuracy and sensitivity of automated cultural interpretation are significant open challenges.
4. Investigations into the predictive rendering capabilities of advanced generative models indicate increasing proficiency in simulating how specific light sources and environmental illumination might interact with depicted product surfaces. This research area aims to allow for the computational generation of imagery showing items under varied simulated lighting conditions, potentially providing users with a richer preview of appearance under relevant real-world scenarios, yet accurately modeling complex material properties and subtle lighting effects remains computationally intensive and often requires significant training data.
5. A notable area of development is the integration of generative and spatial AI techniques into consumer-facing tools allowing for interactive product visualization. These systems enable users to upload personal images and computationally composite digital product models within that scene, attempting to blend the item seamlessly and adjust parameters like scale and perspective, providing a form of virtual placement; however, achieving photorealistic and correctly occluded composites consistently across diverse user-provided images presents substantial technical hurdles.
The Role of Content Agility in Enhancing Product Imagery Strategy - Digitally Staging Products for Specific Audience Segments
As 2025 unfolds, crafting distinct digital product scenes specifically for varied potential customer groups is transitioning from an advanced option to a more common practice. Enabled by the capabilities of current visual generation tools, brands are increasingly moving beyond standard item-on-white presentations. They are creating imagery that places products within simulated environments and contexts designed to resonate directly with specific consumer lifestyles, interests, or even psychological profiles. This approach aims to forge a stronger visual link, helping different audiences see themselves using or benefiting from the product in a way that feels personal and relevant. While the technology allows for considerable variation and responsiveness, effectively tailoring visuals requires a genuine understanding of the diverse perspectives and needs of each audience segment. Without careful consideration guided by human insight, there's a risk that attempts at hyper-relevance could result in visuals that miss the mark or inadvertently feel artificial, failing to build the desired connection or subtle sense of belonging. The challenge lies in using these tools to thoughtfully reflect the nuanced reality of disparate groups, not just generate variations for the sake of it.
Exploration using visual tracking methods, like aggregated gaze mapping derived from user studies or browser-based proxies, indicates distinct ways different consumer cohorts visually scan and focus on product presentations. This observation is prompting developmental work on systems that computationally adjust the visual hierarchy or prominent feature placement within generated imagery, aiming to align better with segment-specific viewing tendencies.
Initial findings from engagement metric analyses suggest that visuals crafted to evoke specific moods or subtle emotional responses, potentially by training generative models on datasets correlated with psychological descriptors or demographic sentiment, appear to correlate with greater user interaction. However, the capacity of AI to synthesize imagery that could implicitly sway perception raises significant questions regarding transparency and the potential for ethically dubious applications.
AI systems are being configured to render product scenes not just contextually but also stylistically. This involves tuning parameters related to color grading, lighting mood, or even simulated lens effects to match aesthetic preferences associated with particular audience segments, moving beyond universal "clean" looks. However, defining and accurately capturing diverse aesthetic preferences programmatically is an ongoing challenge.
Observations suggest that digitally situating products within environments or alongside accessories highly specific to niche interest groups – such as depicting a piece of audio equipment within a simulated studio setting for musicians – seems to enhance the product's perceived relevance and utility for that specific cohort, potentially influencing their valuation.
Explorations into automating the creation of short product video clips are underway, with systems experimenting with programmatically selecting visual pacing and complementing background audio styles. Tailoring elements like the music genre or tempo within these clips to align with preferences associated with different age demographics or cultural groups has, in some preliminary tests, correlated with metrics like increased viewing duration.
The Role of Content Agility in Enhancing Product Imagery Strategy - Tracking the Effectiveness of Iterative Image Updates
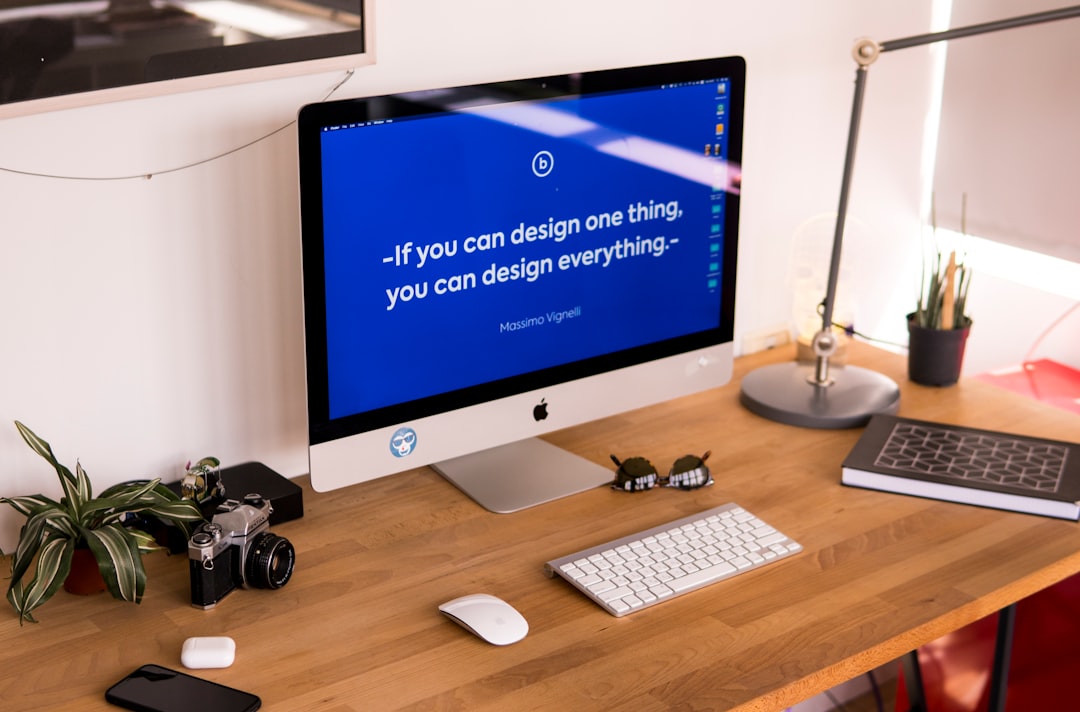
As of May 2025, keeping a close watch on whether rapid, successive changes to product visuals are actually working is crucial for businesses navigating the fast-paced online environment. With the capacity now available to generate or alter imagery quickly through automated tools and digital staging, the emphasis is shifting to understanding the real-world impact of these iterative updates. It's not enough to just create variations; the critical task is measuring which visual adjustments genuinely connect with audiences and drive desired actions, like clicking or buying. This means robust systems for analysis are becoming more central, trying to tease out the effect of a specific image change amidst countless other variables. The challenge lies in building feedback loops that are as agile as the content creation process itself, ensuring that the insights gained from tracking can genuinely inform the next wave of iterations, rather than lag behind or get lost in the speed of production. Effectively doing this requires moving beyond simple metrics and developing a clearer understanding of *why* certain visuals resonate, feeding that knowledge back into the automated and human-guided workflows.
Measuring the impact of repeatedly tweaking product images, especially within the rapid cycles enabled by generative tools or digital staging, presents interesting challenges for a researcher or engineer in 2025. It’s not just about counting clicks or measuring conversion rates anymore. Here are some observations on tracking effectiveness:
1. Quantifying the subtle improvement introduced by an iterative image update can be difficult. While metrics like "image similarity to a target concept" are used in development, determining if that technical improvement translates to a meaningful change in user perception or behavior requires sophisticated user testing beyond simple A/B splits, perhaps involving cognitive load measurement or semantic analysis of qualitative feedback tied to image versions.
2. Pinpointing which specific *aspect* of an iterative image change (e.g., slight lighting alteration, altered background object placement, color temperature shift) drives observed effectiveness metrics is still an open research area. Current tracking often shows an overall impact, but dissociating the effect of granular visual tweaks from the overall scene composition remains complex, limiting the ability to derive precise rules for future automated iterations.
3. The effectiveness of an iterative image update isn't static; it can decay over time as users become accustomed or trends shift. Tracking requires not just measuring immediate lift but also monitoring performance degradation and understanding the lifespan of an image's effectiveness before the next iteration is needed, introducing a temporal dimension to the evaluation challenge.
4. Tracking the *perceived trustworthiness* or *authenticity* of digitally generated or staged iterative images presents a particular hurdle. While metrics like dwell time or click-through can indicate initial engagement, they don't necessarily confirm that the user trusts the visual representation, requiring qualitative validation or tracking of downstream behaviors potentially linked to perception discrepancies.
5. Evaluating the effectiveness of iterative updates for highly personalized visuals tailored to specific user segments adds another layer of complexity. Standard aggregate metrics may mask segment-specific impacts, necessitating robust, potentially compute-intensive, analysis pipelines to attribute performance changes accurately to particular image iterations within targeted cohorts, making small-scale testing and tracking for numerous variations resource-intensive.
The Role of Content Agility in Enhancing Product Imagery Strategy - Managing the Pipeline for Rapid Product Image Variations
Managing the sheer volume and speed of potential visual assets now possible with advanced generation and staging technologies introduces a significant challenge for online retailers, demanding careful orchestration of the workflow, or pipeline, from concept to publication. It’s no longer just about creating variations; it's about building a structured system that can efficiently process inputs, manage the automated steps of generation and staging, and handle the outputs at scale. The efficacy of this pipeline is heavily reliant on the underlying technical infrastructure and the ability to standardize and structure content effectively, enabling seamless transitions between different processing stages and allowing for greater automation throughout the process. A crucial hurdle lies in ensuring this pipeline management system keeps pace with the generative tools themselves, preventing bottlenecks that can negate the advantages of rapid image creation. Furthermore, effectively integrating tracking and feedback mechanisms directly into this operational flow is vital, allowing performance data to quickly inform and adjust future iterations, rather than analysis being a disconnected step occurring outside the core production process.
Here are some observations regarding the technical and practical aspects of managing the systems responsible for creating product image variations at speed, as of May 2025:
1. Experimental setups are integrating near real-time environmental data – sourced potentially from aggregated location data or localized weather feeds – directly into the parameters governing image rendering pipelines. The hypothesis being tested is whether subtly adjusting the computational simulation of lighting and ambient conditions within a product scene to align with the viewer's perceived environment can impact their engagement or perception of realism. The challenge remains in establishing a statistically significant correlation between these nuanced visual adjustments and user behavior across diverse conditions.
2. A developing focus within evaluating the generative models used in product image workflows involves what's being termed "synthetic photography" benchmarks. Instead of relying on comparisons to potentially biased real-world image sets, these benchmarks use renders from meticulously defined 3D scenes with controlled light sources and material properties to test a model's ability to *synthesize* realistic interactions. The critical question arises: does achieving high fidelity on these idealized synthetic tests reliably predict performance on the complexities and nuances of actual product forms and staging requirements?
3. Beyond simple conversion rates, sophisticated analytical modules within product image testing pipelines are attempting to apply AI techniques for more granular user feedback. This involves explorations into inferring user sentiment or perceived visual hierarchy through proxy metrics, potentially analyzing eye-tracking data from opt-in panels or even patterns in mouse movements and dwell times, aiming to inform subsequent image iterations based on more subtle cues than traditional A/B outcomes alone can provide. However, the accuracy and interpretability of such inferred psychological states derived from indirect data sources remain active areas of research and debate.
4. Efforts are underway to extend automated image variation generation capabilities to specifically address accessibility needs. This includes developing algorithms to produce simplified visual representations optimized for contrast or clarity for users with low vision, or programmatically generating richer, context-aware alternative text descriptions by analyzing image content, going beyond standard object recognition to capture implied use or setting. Realizing comprehensive and accurate automated solutions for the wide spectrum of visual impairments presents considerable technical hurdles.
5. There are pilot projects integrating real-time or near real-time inventory and supply chain data directly into the control layers of generative image pipelines. The goal is to computationally ensure that product visuals displayed always accurately reflect current stock levels or available variations (like color options), automatically retiring or modifying imagery for unavailable configurations. This seeks to avoid presenting misleading options but introduces significant data pipeline and synchronization challenges, especially with legacy inventory systems.
Create photorealistic images of your products in any environment without expensive photo shoots! (Get started for free)
More Posts from lionvaplus.com: