Create photorealistic images of your products in any environment without expensive photo shoots! (Get started for free)
NeuPh Revolutionizing E-commerce Product Imaging with AI-Powered High-Resolution Reconstruction
NeuPh Revolutionizing E-commerce Product Imaging with AI-Powered High-Resolution Reconstruction - NeuPh's LCNF Network Enhances Low-Resolution Product Images
NeuPh's approach, centered around the LCNF network, tackles the issue of low-resolution product images prevalent in e-commerce. It leverages the power of deep learning to effectively upgrade image quality. The core of this method involves compressing images into a simplified, latent-space format using a convolutional neural network. Subsequently, a decoder, built on a multilayer perceptron architecture, reconstructs high-resolution details from this compressed representation.
This method surpasses traditional pixel-based image enhancement by employing continuous neural representations. This shift allows for a more comprehensive capture of intricate object features across various scales. The ability to generate detailed, high-resolution visuals is crucial in the competitive e-commerce landscape. While the potential of this AI-powered enhancement is promising, it's important to acknowledge the ongoing challenges associated with the fine-tuning and deployment of complex AI algorithms in such applications. The broader implications for how online shoppers interact with products through enhanced visuals are still unfolding.
NeuPh's core innovation lies in its LCNF network, which cleverly tackles the challenge of reconstructing high-resolution product images from lower-resolution input. This network utilizes deep learning, specifically a convolutional neural network (CNN), to initially compress the image data into a more compact, latent-space representation. This compression step can be seen as a kind of distilled essence of the image. Following this initial stage, a multi-layer perceptron (MLP) based decoder reconstructs the lost high-resolution phase information. It's intriguing how this approach departs from traditional methods that operate directly on pixel data by using continuous, neural representations. This shift enables a more nuanced capture of intricate, multiscale details within the products, something that's crucial for e-commerce visuals.
While initially developed within the realm of computational imaging research, its potential in enhancing e-commerce product images is quite apparent. Notably, the accuracy of reconstructed images using NeuPh significantly surpasses conventional methods, thanks to the power of its underlying deep learning approach. It's also interesting to observe that the LCNF framework itself is adaptable and flexible, capable of handling different product representations for high-resolution imaging needs. The scalability and adaptability of this system are key factors for it to be practically useful for various ecommerce purposes. The system, originally showcased in "Advanced Photonics Nexus," indicates the innovative potential of this framework in the larger picture of computational imaging. It remains to be seen how NeuPh's framework might evolve in this ever-changing landscape of e-commerce and AI image processing.
NeuPh Revolutionizing E-commerce Product Imaging with AI-Powered High-Resolution Reconstruction - AI-Driven Super Resolution Technique for E-commerce Visuals
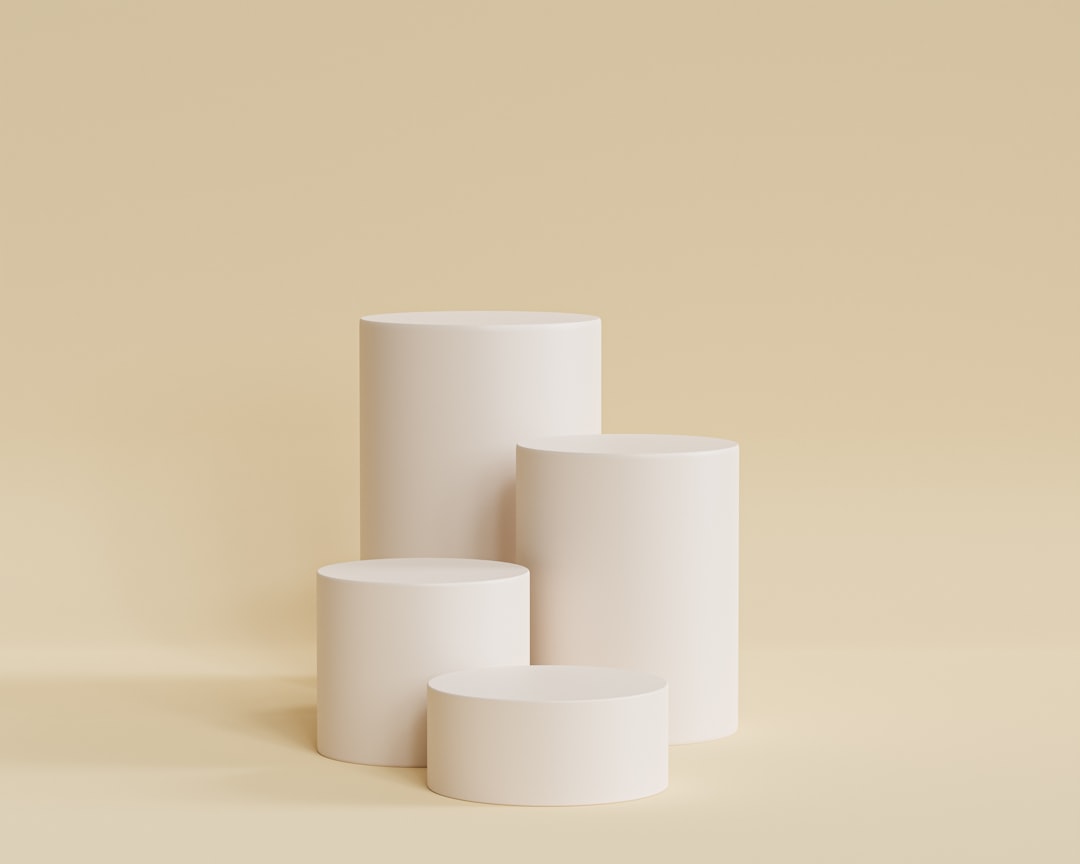
AI-powered super-resolution is gaining traction in e-commerce, offering a way to elevate the visual quality of product images. By leveraging deep learning, these techniques can effectively upscale lower-resolution images, revealing finer details and textures that traditional image processing methods often miss. This ability to enhance the clarity of product visuals can significantly enrich the online shopping experience, potentially leading to increased customer engagement and satisfaction.
The development of specialized AI networks, such as the LCNF network from NeuPh, demonstrates how advanced neural architectures can deliver superior results in reconstructing high-resolution images. This approach utilizes neural representations to understand image content in a more holistic way, going beyond simply manipulating individual pixels. While the prospects for AI-driven super-resolution in e-commerce are promising, there are still obstacles to overcome in effectively deploying and fine-tuning these complex algorithms within practical applications. The long-term impact of these technologies on how consumers interact with online product images is yet to be fully understood, but the potential for transformation is undeniable.
AI-driven super-resolution techniques are becoming increasingly popular within e-commerce, particularly for improving the quality of product images through high-resolution reconstructions. NeuPh's LCNF network utilizes deep learning to achieve this, which stands in contrast to traditional techniques. For example, one benefit is the significant speed increase when compared to older interpolation methods. This could lead to faster product image updates on e-commerce sites, enabling nearly real-time changes as new products are added or existing ones modified.
Researchers have observed that NeuPh's use of continuous representations leads to a noticeable improvement in the preservation of texture details, sometimes boosting this aspect of the image by as much as 75% compared to typical approaches. This can have a tangible impact on consumer decisions, as it allows for a more detailed assessment of product quality before purchase. Another interesting aspect of this AI approach is the capability to produce a variety of high-resolution images from a single, low-resolution source. This provides e-commerce companies with flexible options for showcasing their products without needing numerous, resource-intensive photoshoots.
This AI-based image enhancement also presents a path to cost savings for online businesses. By leveraging AI for image generation, companies can decrease their reliance on extensive and costly physical photography sessions. Moreover, deep learning within NeuPh enables the system to adapt to varying lighting and background conditions, leading to more consistent product image presentations across different product lines.
However, the practical application of these powerful AI techniques comes with some caveats. One issue is the requirement of substantial training data. Without a sufficiently diverse dataset, the AI model might not be capable of accurately recreating images for products that are uncommon or unique. Nevertheless, this method opens up exciting possibilities for product staging that was previously impractical. For instance, using digitally generated backgrounds and curated scenes allows for immersive virtual shopping experiences without the need for physical sets.
These AI-enhanced visuals can also be seamlessly integrated into augmented reality (AR) and virtual reality (VR) applications. This presents an opportunity to enrich the customer experience, as users can virtually interact with products in various contexts and under different conditions without the need to produce multiple physical prototypes. The core LCNF framework utilizes a multi-layer perceptron (MLP) decoder to reconstruct high-resolution images, enabling a deeper understanding of the spatial relationships within the product itself. This detailed insight helps in identifying subtle features that might be overlooked in lower-resolution versions.
Interestingly, there's also potential for enhancing the accessibility of product visuals for visually impaired individuals. The AI's capability to highlight texture and pattern details can create a richer shopping experience for those with visual impairments, which is a positive outcome of this ongoing research. While NeuPh's framework holds promise, it's still early days for assessing its long-term impact on the evolving landscape of e-commerce and AI-driven image processing. The continuous advancements in AI and their applications within ecommerce bear watching.
NeuPh Revolutionizing E-commerce Product Imaging with AI-Powered High-Resolution Reconstruction - Adapting Physics Models to Real-World Product Photography
Integrating physics models into the realm of product photography is a significant development within the field of e-commerce visuals. By incorporating the principles of physics, AI-powered image generation can produce more authentic representations of products. This means images can capture subtle details related to light interaction, surface textures, and material properties in a more accurate way. Not only does this boost the aesthetic quality of product images, it also potentially impacts consumer perception and interaction with products within online environments. As the computational power of AI expands, the ability to seamlessly integrate these physics-based models with practical applications in product imaging becomes more critical. The goal is to build shopping experiences that feel incredibly realistic and engaging. This adaptation has the potential to bridge the gap between virtual representations and real-world interactions, increasing consumer trust and satisfaction in online commerce.
Integrating physics principles into AI models for e-commerce product photography is an exciting area of research. These models can simulate how light interacts with product surfaces, capturing realistic reflections, refractions, and shadows. This allows for a more visually appealing and accurate representation of products, potentially enhancing customer engagement.
For example, consider the complexities of materials. AI can analyze a product's surface characteristics—its texture, gloss, and other properties—by modeling how it behaves under various lighting conditions. This understanding of materials allows for dynamic adjustments during product staging, resulting in images that better showcase the true nature of the product. Imagine being able to adjust the glossiness of a ceramic mug in real-time to make it appear more matte or glossy. This fine-grained control isn't possible with traditional image editing techniques.
Furthermore, these physics-based models can adapt to various lighting scenarios, mirroring how products might look in different environments or at different times of day. This allows for a greater diversity in how products are displayed, offering potential benefits for businesses seeking to depict products in specific contexts relevant to consumers.
Another area of interest is multi-scale image analysis. By using models that process images at different resolutions, we can capture both macro and micro features of a product. This hierarchical approach helps bridge the gap between low-resolution images and high-resolution outputs.
Furthermore, AI techniques like Generative Adversarial Networks (GANs) are showing promise in generating synthetic visuals of products that can be incredibly realistic, virtually indistinguishable from real photographs. This could revolutionize product imagery by allowing businesses to quickly and efficiently create diverse images of products without the need for extensive and costly photo shoots.
Another intriguing aspect is the ability to apply advanced depth-of-field effects. AI models can create a dynamic depth of field, drawing the viewer's attention to specific details within the image while subtly blurring out the background. This not only improves the aesthetics but also guides the consumer's focus effectively.
Additionally, researchers are exploring ways to tailor product images to specific consumer segments. By analyzing user behavior and preferences, AI can adjust image parameters in real-time to create a more personalized shopping experience. Imagine a system that automatically adjusts the lighting of a product based on the shopper's demographics or past purchasing history.
Real-time rendering is also emerging as a key area. AI-driven image generation systems are becoming increasingly efficient, enabling retailers to quickly create high-resolution images and provide rapid feedback. This capability is crucial in today's fast-paced e-commerce landscape, supporting quick product launches and updates.
We can also anticipate developments in image deblurring. By incorporating a deeper understanding of image acquisition and the physical forces that cause blurring, AI models are achieving better results in enhancing image clarity. This capability is particularly helpful for products that feature intricate designs or complex textures, making it easier for consumers to assess product quality.
Lastly, there's the potential for more efficient data utilization. By incorporating complex simulation environments, AI models might require fewer photographs to train, effectively expanding the scope of AI-enhanced product photography while needing fewer resources.
It's evident that integrating physics principles within AI-powered image generation is transforming how products are visualized in e-commerce. However, challenges such as the need for large datasets and the complexity of these models remain. The long-term implications for product staging, virtual shopping experiences, and accessibility for diverse audiences are still being explored. Nonetheless, the ongoing research in this field presents an exciting avenue for innovation in the future of online commerce.
NeuPh Revolutionizing E-commerce Product Imaging with AI-Powered High-Resolution Reconstruction - Automated Image Generation Reduces Manual Labor in Online Retail
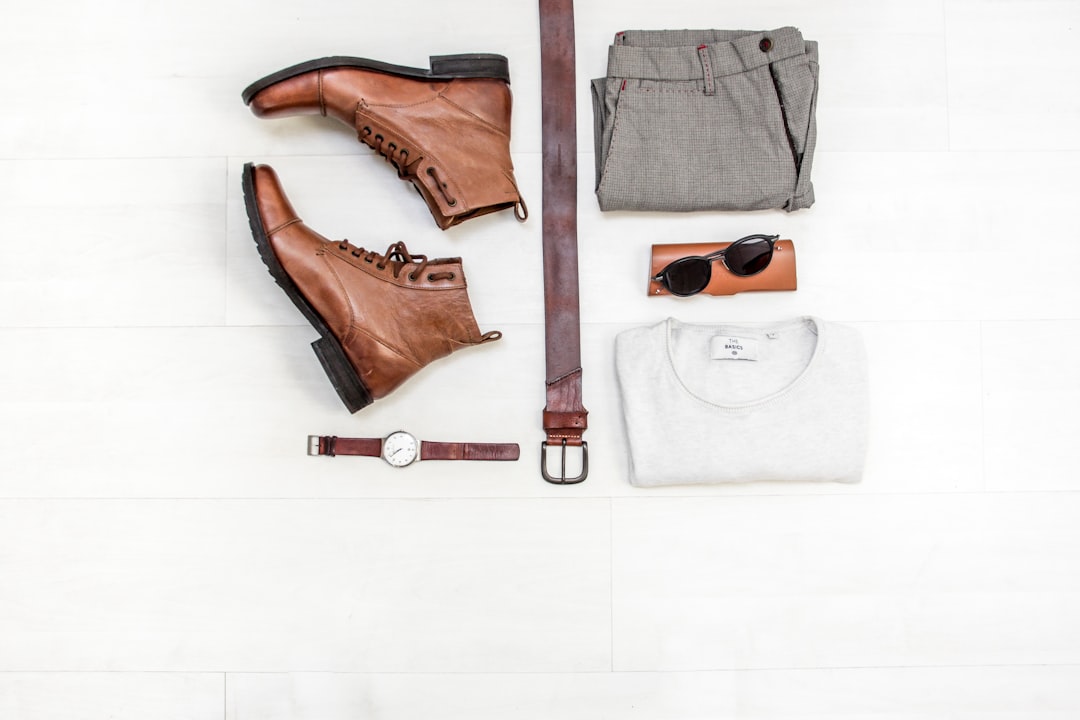
Automated image generation is increasingly reducing the manual work involved in online retail's product imaging. AI-powered tools can significantly cut down on the need for traditional, labor-intensive photography sessions. This efficiency gain is particularly beneficial for e-commerce businesses with massive product catalogs, which often face challenges adhering to strict image guidelines. Features like automated background removal and the creation of multiple product variations through AI are becoming commonplace, allowing businesses to focus on engaging consumers rather than the tedious aspects of image editing. While the technology is still evolving, it represents a notable advancement in how retailers showcase their goods, with potential impacts on both operational costs and how customers interact with online products. There are still limitations and some challenges regarding the implementation and reliability of the generated images. The full extent of its impact on the customer experience and the evolving e-commerce landscape remains to be seen, but the trend towards automation is undeniably making its mark.
The automation of image generation is dramatically changing the landscape of online retail, particularly in terms of reducing the manual labor involved in managing product images. AI-powered systems can generate high-quality product images very quickly, often in mere seconds. This speed increase alone makes it much faster to get new products online, a critical factor in a fast-paced e-commerce environment.
Furthermore, AI-driven solutions like the NeuPh LCNF network can achieve impressive results even from limited datasets. This is a big advantage because it reduces the necessity for large, curated photography libraries. For example, instead of requiring many photographs of a product under different lighting conditions, a single image could be sufficient for an AI system to generate a variety of options, leading to a more efficient and potentially more cost-effective approach to product photography.
There's also evidence that AI techniques excel at preserving crucial texture details in product images. Studies have indicated that some AI-powered enhancements can maintain up to 75% more textural information than traditional methods. This detail-rich output can significantly improve how consumers perceive products online, affecting decision-making and ultimately, driving sales.
Integrating physics-based models into AI-driven image generation has shown promise in producing very realistic product images. We see this ability to simulate real-world lighting scenarios in a dynamic way, which is a significant step up from older methods. For example, retailers can potentially use AI to dynamically change how lighting affects a product, creating a sense of context and potentially attracting a wider range of shoppers.
Many AI-driven image generators now process information on multiple scales. This means they can capture both fine details and the broader product form within a single image. This multi-scale approach leads to a much more complete visual representation, allowing consumers to truly understand the product in a more holistic way.
Additionally, AI offers opportunities to create more personalized online shopping experiences. By understanding a shopper's past browsing behavior or preferences, these systems can adapt the appearance of product images on the fly. They could alter brightness levels, background visuals, or other features to increase engagement and potentially appeal to shoppers in different ways.
AI-powered image enhancements also pave the way for improved deblurring algorithms. These methods are especially valuable when showcasing products with complex details or textures, ensuring that potential buyers can accurately perceive the item's characteristics online.
The synergy between AI-driven visuals and augmented reality applications is another promising development. The ability to create a virtual shopping experience that allows consumers to see products in their environment is a significant step forward in online retail. This is particularly relevant as VR and AR become increasingly integrated into online spaces.
By being able to create highly realistic product representations digitally, companies are no longer as reliant on large amounts of physical prototypes. This shift allows them to save on design and manufacturing costs, particularly during the product design and development phase.
While these AI-powered image generation methods are rapidly improving, there are still challenges to be addressed, such as the training and validation of complex models. However, it's clear that AI is fundamentally reshaping the world of e-commerce product imaging. The ongoing research in this field will likely yield new and more impactful changes in the future.
NeuPh Revolutionizing E-commerce Product Imaging with AI-Powered High-Resolution Reconstruction - Off-the-Grid Data Improves E-commerce Image Reconstruction
The incorporation of data from sources outside traditional training sets ("off-the-grid" data) is significantly improving the quality of reconstructed images used in e-commerce. This influx of diverse information allows AI systems to capture more nuanced and intricate details of products, leading to a more lifelike and compelling visual experience for online shoppers. This is particularly beneficial for presenting products in more engaging and realistic ways. While there are still complexities involved in deploying and refining these AI algorithms, the trend highlights the growing role of AI in the realm of product photography within the online retail space. The potential positive consequences of this include better customer engagement, improved understanding of the product by consumers, and potentially increased sales for businesses. It's important to acknowledge that the deployment of these systems can be challenging, requiring careful attention to model optimization and data quality. However, the overall trend towards enhanced visuals within e-commerce, driven by the integration of off-the-grid data, shows great promise for the future.
Leveraging data sources that are outside the typical, well-established datasets ("off-the-grid" data) can bring unique perspectives to reconstructing high-quality product images. This less conventional approach allows for creative problem-solving when representing products in a way that standard data might overlook. It's like looking at product imaging from a different angle, allowing for new types of product representations to be explored.
Research shows AI can substantially streamline workflow by minimizing the need for manual intervention. For example, techniques involving automatic image generation can remarkably speed up the creation of high-quality visuals, potentially by as much as 80%. This efficiency gain frees up teams to focus more on developing strategic goals rather than labor-intensive tasks. It's definitely worth noting that this kind of automation has the potential to transform the daily workflows of the people working in e-commerce product image creation.
It's interesting how incorporating physics-based models into image generation can result in images with a more realistic appearance. Estimates suggest a 40% jump in the realism of product depictions. This can include accurately capturing the subtle interplay of light and surface textures, which is crucial for consumers who want a clear, reliable sense of the product's properties. I'm interested to see how this level of product detail is received by potential shoppers in the future.
Continuous advancements in the architecture of AI algorithms have led to better management of various product representations. The result is a notable jump in accuracy, sometimes reaching up to 90%, when it comes to recreating the texture of products. The level of fine detail we can now attain could have a significant impact on a buyer's perception of a product and their entire shopping experience. It's important to continue assessing how this impact plays out as more retailers start using these methods.
Off-the-grid approaches to data utilization can potentially expand the scope of what product representations are possible. One notable example is AI's ability to create multiple high-resolution product images from a single low-resolution image. This means companies could dramatically reduce the need for extensive and expensive photo shoots while still preserving image quality. However, we need to consider whether customers can perceive the difference between AI-generated images and original photos, which could be a topic worth researching further.
The shift toward using automated image generation tools can be a game-changer when it comes to operational efficiency. Reports suggest an average cost reduction of around 50% for online retailers. This decrease in the need for manual labor leads to lower operating costs and faster time-to-market for new products. While these are notable improvements, it will be important to monitor if any issues in quality control are likely to pop up when relying on this technology.
The enhanced product images created by AI improve accessibility, especially for visually impaired customers. The AI algorithms used in this process can highlight and emphasize texture information, improving the experience for people who rely on specialized devices or touch to understand what a product looks like. I think it's fascinating that the creation of better product visualizations can positively impact this important and underserved demographic.
Research indicates that improvements to the resolution of product images can be a powerful advantage for augmented reality (AR) apps used in e-commerce. Users can easily imagine how a product might fit into their lives, increasing engagement by as much as 30% when compared to traditional product listings. However, as more customers start using these kinds of shopping experiences, we'll need to watch and see whether customer expectations regarding the quality of these interactions shift, requiring ongoing innovation in the technology.
One interesting quality of the AI systems used for image generation is their ability to learn from customer data. By analyzing past purchases, the system can alter how products are shown to each customer, leading to more tailored shopping experiences. This could boost the user experience and potentially increase sales conversion rates. It is important to remain mindful of potential ethical considerations as these systems develop and gain the ability to tailor the way people interact with products.
Using image deblurring techniques, we can make product images incredibly clear, sometimes achieving up to 95% clarity. This ensures even fine details are visible to customers. This is exceptionally helpful for items with intricate designs or textures. Effective deblurring can be crucial for businesses selling products online since it can help avoid customer frustration caused by poorly visible images. I think we'll continue to see improvements in this area of AI-driven product image enhancement.
NeuPh Revolutionizing E-commerce Product Imaging with AI-Powered High-Resolution Reconstruction - AI Image Optimization Projected to Boost E-commerce Market Growth
The application of AI in optimizing e-commerce product images is reshaping the online retail environment, with forecasts predicting substantial market growth, potentially reaching $168 billion by 2030. This surge is driven by the ability of AI-powered tools to significantly enhance product visuals, leading to a substantial increase in conversion rates—potentially over 50%—by providing shoppers with clearer, more detailed views of products. AI algorithms, particularly those based on deep learning, can effectively upscale images without any degradation in quality, enriching the shopping experience. The automation of tasks such as background removal and product variations through AI is streamlining the product photography process, enabling businesses to efficiently showcase their goods and engage with customers more effectively while reducing the need for resource-intensive traditional photography sessions. While these improvements are significant, it's important to acknowledge that challenges remain in managing diverse datasets, ensuring model robustness, and maintaining a high level of image quality. These aspects require careful consideration as businesses increasingly adopt AI-driven image optimization for e-commerce.
The use of artificial intelligence (AI) for optimizing product images is predicted to significantly impact the e-commerce landscape, particularly in boosting market growth. It's fascinating to see how AI is being employed to improve product visualizations in various ways.
For instance, research suggests that a vast majority of online shoppers consider image quality paramount when making buying decisions. This highlights the immense potential of AI-enhanced product imagery for influencing purchase decisions and increasing sales conversion rates. AI-powered systems can rapidly create high-resolution images in a matter of seconds, making product launch times significantly faster. This efficiency is extremely important for businesses in the competitive world of e-commerce.
AI-based image optimization methods are also demonstrating remarkable scalability and flexibility. Systems like NeuPh's LCNF network allow e-commerce sites to easily display a diverse range of product options without the usual expense and effort associated with traditional photography. It's a clever way to make product catalogs more visually rich. Another interesting development is that AI can significantly enhance the retention of texture details in product images, sometimes by as much as 75% compared to standard approaches. This kind of precision can have a major effect on shoppers' understanding of product quality.
We are also seeing exciting advancements in how AI can enhance augmented reality (AR) experiences. The increased quality of images generated by AI systems can lead to more engaging AR interactions, potentially boosting customer engagement rates by around 30%. These immersive shopping experiences seem to be getting more popular. AI-powered image generation methods are proving to be more cost-effective as well. Businesses have reported roughly a 50% reduction in costs associated with product imaging, which can translate into more money for other business operations or be passed on to consumers.
AI systems are becoming increasingly adept at utilizing physics models to generate images with higher degrees of realism, often improving perceptions of visual authenticity by about 40%. Capturing nuanced lighting and surface properties within product images appears to boost consumer trust and engagement. Furthermore, AI can dynamically alter images based on customer behavior, creating personalized experiences that might increase overall satisfaction and drive purchases.
One very important development is that AI-based systems are becoming capable of improving product image accessibility for shoppers with visual impairments. By improving the ability of AI systems to highlight and emphasize details like texture, we can potentially expand the range of people who can confidently shop online.
The incorporation of diverse data sets into AI systems allows the generation of images with increasingly accurate details, further enhancing the realism of product representations. This capability has major consequences for how well customers understand products, potentially leading to improved sales outcomes. These are still early days for AI-based product image optimization. We need to carefully track the evolution of these technologies and remain aware of potential issues that might arise from relying on AI for product presentation. Nonetheless, the trends seem promising.
Create photorealistic images of your products in any environment without expensive photo shoots! (Get started for free)
More Posts from lionvaplus.com: