Create photorealistic images of your products in any environment without expensive photo shoots! (Get started for free)
Identifying Digital Art Brushes 7 Key Techniques for Product Photo Enhancement in AI Image Generation
Identifying Digital Art Brushes 7 Key Techniques for Product Photo Enhancement in AI Image Generation - Brush Pattern Recognition Through Neural Network Style Analysis In Midjourney V6
Midjourney V6 has made strides in recognizing and manipulating artistic styles through its neural network, offering new possibilities for ecommerce product imagery. The improved neural network allows for a more nuanced understanding of user prompts, resulting in images that more accurately reflect the desired aesthetic. This means that product images can now capture a specific artistic brushstroke or painting style with greater fidelity. The ability to generate images with a higher degree of contextual awareness is also beneficial, helping the AI produce product images that feel more integrated within the intended setting or environment. Midjourney V6 also grants more direct control over image generation through features like remix mode and refined prompting tools. Ecommerce businesses can use this newfound control to fine-tune the 'look' of their product images – achieving a hyperrealistic product photo, or perhaps a more whimsical or stylized visual depending on the branding. This level of customization can be crucial for standing out in an increasingly competitive marketplace where compelling visuals are vital. While it’s not perfect, Midjourney V6 is increasingly able to meet the demand for a wide range of product photography styles, and its focus on user-driven aesthetics makes it potentially appealing to those seeking a more approachable AI art generation experience.
Midjourney V6's approach to brush pattern recognition is intriguing. It relies on intricate convolutional neural networks (CNNs) that meticulously dissect texture and stroke details within images. This allows the system to distinguish subtle differences between brushes, going beyond basic identification.
Interestingly, V6 refines the brush recognition process through a continuous feedback loop. User interactions and outcomes guide the model's learning, effectively shaping its ability to accurately predict and recommend the right brush for a given artistic style. This dynamic approach is a significant leap forward from traditional brush detection methods.
Furthermore, Midjourney utilizes transfer learning within its neural network architecture. This means it leverages previously trained models to quickly adapt to new brush styles. This speeds up the learning process, reducing the reliance on massive datasets for each new brush type.
One potential application of this capability lies in enhancing product photo generation. Imagine creating hyperrealistic depictions of artistic effects on products for e-commerce. The ability to mimic real-world brushstrokes with precision could significantly improve the visual fidelity of these images.
Beyond the obvious, this brush recognition also offers opportunities for greater customization. Users could define artistic styles that align with specific brands or product aesthetics. This level of control leads to more personalized and impactful visual content, ultimately enhancing the shopping experience.
The potential for workflow efficiency is also significant. Imagine searching through a vast library of brushes—a time-consuming process. The AI's accuracy in brush detection significantly cuts down this time, benefiting e-commerce environments where speed and turnaround time are paramount.
The system also incorporates clustering algorithms to group similar brushstrokes. This creates an organized framework for brush selection, valuable for product staging or themed marketing campaigns.
However, a caveat: the quality of the input data greatly impacts the brush recognition's effectiveness. High-quality, well-structured datasets consistently yield much better results than datasets that are inconsistent or lack clear patterns.
The reliance on community feedback is notable. Midjourney incorporates user-generated data into its learning process. This approach highlights the potential for continuous improvement, with user interactions directly shaping the neural network's performance.
Finally, e-commerce is constantly evolving as consumer preferences change. Midjourney V6's ability to recognize and generate novel brush effects provides a flexible tool for brands to adapt and stay visually compelling. It becomes a potent asset for brands wanting to stay ahead of the curve in a visually competitive market.
Identifying Digital Art Brushes 7 Key Techniques for Product Photo Enhancement in AI Image Generation - Digital Watercolor Effects Applied To Product Shadows Using Photoshop Beta 2024
Photoshop Beta 2024 introduces a new way to enhance product shadows with digital watercolor effects. This addition to the software allows users to mimic the soft, blended look of traditional watercolor paintings, creating a unique visual style for product images. The core of this technique lies in applying specialized filters and brushes designed to replicate watercolor's characteristic blur and color washes. It essentially enables designers to infuse a delicate, artistic touch into product photos, potentially increasing visual appeal and potentially giving a product a hand-crafted feel.
While this feature offers a novel approach to product image refinement, its successful implementation hinges on the user's understanding of Photoshop and their ability to master the nuances of digital watercolor. The challenge lies in blending digital tools with established design principles to achieve a desirable outcome. It remains to be seen how widely adopted these watercolor shadow effects will become, but the potential to differentiate products through a unique visual aesthetic is undeniable. Ultimately, it becomes another tool in a designer's toolbox to improve the online shopping experience.
The 2024 Photoshop Beta version offers intriguing new features for crafting digital watercolor effects, particularly when applied to product shadows. These effects seem to be driven by improved algorithms that try to simulate how light interacts with surfaces, leading to more natural-looking shadows that resemble those captured in regular photography. This update seems to focus on mimicking subtle variations in light and shade, which is key for making product images more appealing in e-commerce contexts.
One noteworthy addition is the updated layering system. It now lets artists fine-tune shadows by adjusting transparency and blending modes. This creates a multi-layered effect and might improve the illusion of depth, which is crucial for showing off products in a more lifelike way. It's interesting how the software now tries to integrate color theory into shadow creation. Using AI, Photoshop suggests colors for shadows that are meant to complement the product's colors, potentially making the product stand out more. While this sounds useful, I wonder about the actual efficacy in terms of improving sales.
Performance has also been a focal point. GPU acceleration has been integrated, leading to faster rendering times for watercolor effects, including the shadows. This might be very beneficial for designers who need to create a lot of images quickly. The algorithm used for watercolor effects has been tweaked to use "smart sampling" for better texture accuracy. This approach means the software attempts to match the textures of the shadows to the product's materials, creating a more realistic image. However, it remains to be seen how accurately this can be achieved across the range of textures commonly found in e-commerce products.
The addition of dynamic lighting capabilities allows designers to directly manipulate the light source in the image. The shadow effect will then be recalculated based on the new light, allowing for a higher degree of control over how products are presented. This is likely valuable for those looking to depict products in various scenarios or settings. Further expanding on creative control, users can now design and store custom brushes for shadow effects. This could be especially useful for businesses that want their products to have a consistent visual language. It's an intriguing way to differentiate their online product presentation.
The beta version appears to be incorporating user feedback into its development process. So, as users use the tools and provide feedback, the watercolor algorithms and shadow creation features might improve over time. This is a common strategy with AI-powered software, aiming to create an adaptable and responsive system. It's also worth noting that this version of Photoshop seems to be designed to work more smoothly with existing AI-based image generators. This interoperability is a positive sign and could streamline workflows for people who use a range of AI tools for product imaging. There is even a feature that predicts trending shadow styles based on existing e-commerce visuals. While potentially helpful, I have reservations about how this will be implemented and whether it will lead to an overreliance on predictable visual styles rather than genuine creativity. It is a double-edged sword.
Overall, the direction of the Photoshop Beta in relation to watercolor effects and product shadows seems promising. It's definitely worth watching as the technology evolves to understand the balance between automated features and the need for creative control in product imagery for e-commerce.
Identifying Digital Art Brushes 7 Key Techniques for Product Photo Enhancement in AI Image Generation - Gradient Mapping Techniques For Metal Surface Enhancement In DALL-E 3
DALL-E 3's introduction of gradient mapping techniques is a significant step forward in crafting realistic metal surfaces, especially relevant for e-commerce product photography. By skillfully manipulating color gradients, users can achieve a greater degree of visual realism in their AI-generated product images. This leads to a more captivating and detailed representation of metallic finishes, helping products stand out online. Furthermore, gradient mapping techniques offer designers enhanced control over product staging, making it easier to create visually compelling scenes that are likely to resonate with customers. While it's still a relatively new feature, the potential of gradient mapping in enhancing product visuals and shaping e-commerce aesthetic is undeniable. As AI image generation tools mature, understanding how to effectively utilize such techniques will be crucial for businesses aiming for a strong visual presence online. However, the efficacy of these methods in actually improving sales remains an open question, and there's a risk of relying too heavily on AI features instead of focusing on core design principles.
DALL-E 3's gradient mapping capabilities offer a refined way to enhance metal surfaces within product images. By meticulously analyzing how light interacts with metals at different angles, it can generate a level of realism that elevates the visual appeal of products, particularly when showcasing intricate details or finishes. This method promises a more effective way to present product features compared to traditional image manipulation techniques.
This approach can effectively create depth and dimension on metal surfaces through the use of layered gradients. By simulating various lighting conditions, it can suggest the physical form of the product, a crucial factor for online shoppers who rely heavily on visual cues when making purchase decisions. It’s like the AI is attempting to ‘understand’ how light and shadow would work on a 3D object, and this information then informs the generated image.
Gradient mapping in DALL-E 3 also allows designers to experiment with intricate color schemes on metallic objects. Imagine replicating a brushed metal finish, or the look of anodized aluminum – these are within the realm of possibilities. This flexibility can be beneficial for product differentiation, and can help tailor the aesthetic appeal to specific customer segments.
Intriguingly, gradient mapping also offers potential for subtle emotional manipulation. By adjusting color gradients, DALL-E 3 can evoke a feeling of warmth or coolness, which might influence consumer perception and purchasing decisions. While not fully explored, the idea of linking emotional responses to gradient variations is interesting and highlights the model's ability to move beyond mere realism. However, this capability comes with a degree of ethical consideration – should AI be subtly driving emotions in consumers?
One area where gradient mapping shows limitations is its dependency on the input image. For low-resolution or poorly captured images, the enhancements may be less effective or even degrade the overall image quality. This reinforces the importance of quality product photography as a fundamental part of the online shopping experience.
DALL-E 3's underlying machine learning algorithms play a significant role in driving this gradient mapping technology. By training on massive image datasets, the model gains a sophisticated understanding of how gradient shifts impact human perception of surfaces. The end result is images that are not just visually appealing but also serve as powerful tools in online product marketing.
The realistic rendering of reflections and highlights on metal is another noteworthy feature. The AI simulates how the environment might be reflected on a product’s surface, providing greater contextual awareness within the image. It’s a technique that effectively blurs the line between AI-generated and real-world product photos, which might improve the online shopping experience by reducing the sense of digital separation.
There’s a chance that gradient mapping could automate a large portion of the image enhancement workflow. If applied in a batch processing context, businesses could rapidly refine large numbers of product images, all with a consistent level of enhancement. This possibility of streamlined workflows holds substantial appeal for e-commerce environments where quick turnarounds are essential.
The versatility of this technique isn't just limited to metal. It can also be adapted to generate diverse textural effects, such as recreating rust or patina on other materials. This adaptability could be useful for product categories that aim to project a vintage or rustic feel. However, we must ensure that the techniques are applied with care and restraint.
The power of gradient mapping comes with a responsibility to avoid overusing it. Too much manipulation or a clumsy implementation can quickly degrade the image's authenticity. Designers should strike a careful balance between artistic expression and realistic representation. This careful approach will ensure that the final image is not only aesthetically pleasing but also builds consumer trust in the product being advertised.
Identifying Digital Art Brushes 7 Key Techniques for Product Photo Enhancement in AI Image Generation - Texture Overlay Methods Using Custom Brush Libraries In Stable Diffusion XL
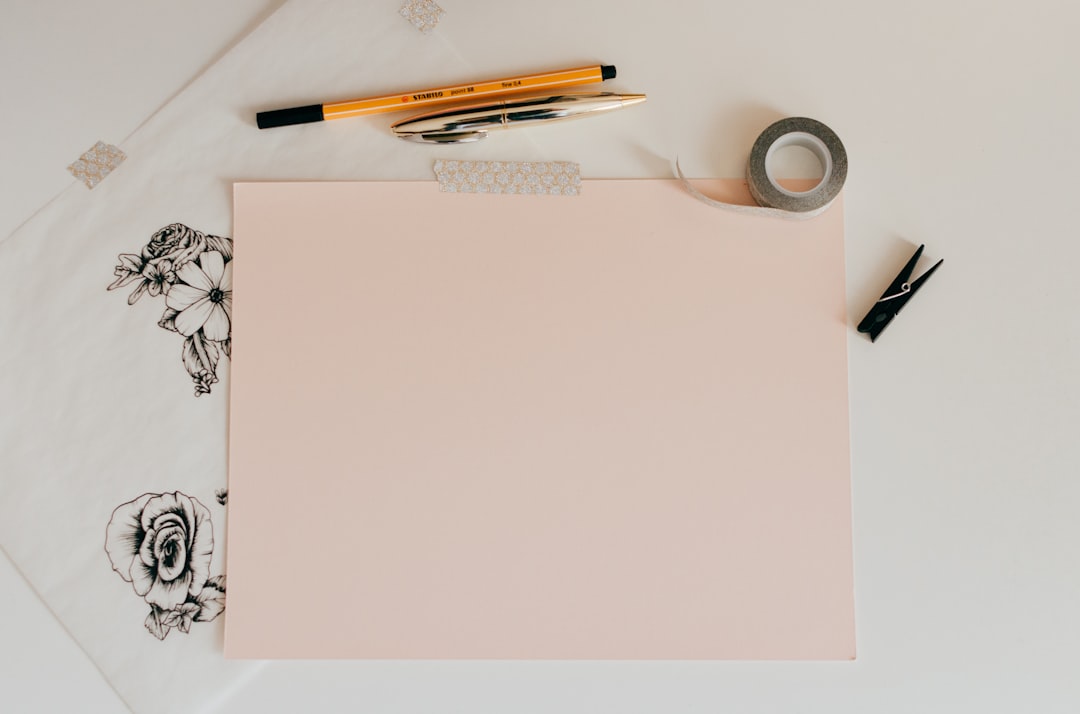
Stable Diffusion XL introduces a new level of control over texture in AI-generated images, which is particularly valuable when creating product visuals for e-commerce. The ability to incorporate custom brush libraries directly into the image generation process opens up a range of possibilities for achieving unique and realistic textures. This allows for a more nuanced approach to product staging, enabling e-commerce businesses to create visually compelling environments that better showcase their offerings.
One key benefit is the potential for generating seamless textures that tile perfectly, ensuring a cohesive look across product images and marketing materials. While the process requires familiarity with the Stable Diffusion interface and prompt engineering, it offers a level of artistic expression that wasn't previously possible with AI-driven image generation. However, relying too heavily on these new methods can sometimes lead to an over-reliance on AI-generated textures, and it's crucial to balance this with thoughtful design principles and a sense of visual restraint.
In the realm of product photography for online shopping, the ability to precisely control texture can enhance a product's appeal and communicate important details about its material or finish. By embracing these newer techniques, designers and brands can improve the overall customer experience, especially within the realm of e-commerce where shoppers often rely heavily on visuals when making purchase decisions. It will be interesting to see how the development of these techniques evolves in the future, particularly as the demand for more photorealistic AI-generated imagery grows.
Stable Diffusion XL's (SDXL) ability to utilize custom brush libraries opens up a new realm for texture overlay techniques. It's a powerful extension of previous Stable Diffusion versions, primarily focused on boosting the photorealism of AI-generated art, and it’s particularly interesting in the context of e-commerce product images. By crafting custom brushes, designers can simulate a vast range of textures – from fabric weaves to metallic finishes – making the product photos more engaging and potentially more persuasive.
One of the key advantages of using custom brush libraries is the speed and ease with which textures can be applied. This is crucial in e-commerce, where a consistent and polished visual identity is key to building brand recognition. It’s much easier to apply a particular texture across a range of products when you have a set of curated brushes, improving efficiency for companies that deal with a vast number of images. Furthermore, the ability to layer and blend different textures opens doors for complex visual effects, potentially helping products stand out. There are subtle yet powerful ways that textures can be combined to make an image more compelling.
There's also a degree of customization that’s now possible within the workflow, and it's relevant for companies looking to craft a unique brand presence. These custom brushes can be tweaked and developed further by individual users, which means the visual style of an online store can be more explicitly tied to a brand's identity. The danger is that many e-commerce sites might adopt a similar aesthetic based on what they believe will resonate with consumers.
What’s also noteworthy is the way Stable Diffusion XL incorporates community feedback. This 'interactive' component could lead to a sort of feedback loop where custom brushes get continually refined based on user preferences. Since visual trends shift quickly, adapting to new styles is beneficial for businesses who want to stay current with a constantly evolving online environment.
Beyond simple applications, the overlay methods enabled by these brushes are leveraging machine learning to interpret and reproduce fine details in textures. This can be seen in how it handles the subtle sheen of metal, or the distinct weave patterns in a fabric image. The higher quality output potentially makes a difference in how consumers perceive the value of a product.
The integration with other commonly used design software is a further plus. It can mean that Stable Diffusion XL can be seamlessly embedded into an existing design workflow involving tools like Photoshop. There is no need for a lengthy intermediate step, which improves the speed and efficacy of the entire process. Similarly, it can also effectively reproduce real-world lighting scenarios, accurately creating shadows and highlights that might enhance trust in the product being depicted.
A benefit for large-scale e-commerce businesses is the potential to utilize these tools for scaled-up production without sacrificing quality. It's possible to generate multiple variations of product images with very consistent textural application, which simplifies image generation at a high volume.
Interestingly, there's also the potential to use these tools for subtly influencing the feelings or emotions a viewer might get when interacting with a product image. We're getting close to using textures in a marketing context, not just for displaying a product, but for subtly encouraging desired actions or responses. The ethical implications of such 'emotion engineering' are something to watch closely. It’s a technology that’s still evolving and might become a powerful tool for manipulating consumer perception.
Overall, SDXL's ability to handle custom brush libraries for texture overlay represents a significant step forward in AI-generated imagery, and it's likely to become a key component in many e-commerce workflows in the coming years. The technology allows for rapid iteration, customization, and refinement, potentially delivering compelling images that encourage purchases. But as with any new technology, its application needs to be mindful of ethical considerations. It's exciting to see what creative solutions emerge from this ongoing development.
Identifying Digital Art Brushes 7 Key Techniques for Product Photo Enhancement in AI Image Generation - Light Reflection Brush Automation Through AI Object Detection
AI object detection is increasingly being used to automate tasks in digital art, specifically in areas like enhancing product imagery for e-commerce. One emerging application is the "Light Reflection Brush Automation," a technique that aims to improve the realism of product photos. This technique leverages AI to intelligently analyze images, identify product surfaces, and then automatically generate realistic light reflections. These reflections are a vital element in creating visually compelling product images, making objects appear more three-dimensional and improving their overall aesthetic appeal.
By automating this traditionally manual process, the technique has the potential to significantly streamline the creation of product visuals for online stores. This not only reduces the time required to produce high-quality images but also frees up designers to focus on other aspects of product presentation and image composition. While still a developing field, light reflection automation through AI object detection is expected to become a valuable tool for e-commerce businesses that aim to enhance their product images and make them more engaging for online shoppers. However, there is a concern that over-reliance on such automation could potentially lead to a homogenization of online product visuals and a diminished emphasis on truly creative visual design. It will be interesting to see if the future of this technology addresses these potential downsides.
The potential impact on the way products are visually presented is noteworthy. The ability to easily and quickly generate convincing light reflections on various product surfaces could greatly improve product staging. More sophisticated scenes can be generated that are optimized to highlight particular features of the product and provide more context about how a product might fit within a shopper's desired setting. There's a chance that AI could become integral in the evolving realm of online product presentations, altering how brands tell their visual stories. The degree to which this technology influences future e-commerce aesthetics remains to be seen, and it prompts questions about the role of creativity in the rapidly evolving world of online shopping.
AI object detection, a technique increasingly used in various fields, is now being explored for automating light reflection brushwork in the context of ecommerce product images. This emerging approach leverages AI's capacity to analyze images and identify key features of a product, which it then uses to intelligently apply digital brushes to simulate how light interacts with those surfaces. It's essentially like having an AI-powered lighting technician fine-tuning the illumination on every product image.
One of the core aspects of this is the quest for optical accuracy. The ambition is to create light reflections that are not simply visually appealing, but also adhere to real-world physics. By incorporating principles of optics into the AI models, we can generate reflections that change dynamically based on factors like the angle of light sources and the material of the product. This detailed level of light control is vital for conveying the true texture and visual qualities of a product.
It’s not just about a single layer of light either. AI can layer light effects like highlights, and softer, diffused lighting. This layering is crucial for achieving a sense of depth and making products appear more three-dimensional. This is especially useful for images showcasing detailed surfaces or products with complex shapes.
Integrating AI object detection means we are also approaching real-time control of the lighting and reflection effects. The possibilities are exciting. Imagine a user interacting with an e-commerce product page and, depending on their actions, the lighting dynamically changes to showcase certain aspects of the product. It can highlight textures, or demonstrate how a metallic surface might glisten under different conditions.
Furthermore, AI-driven lighting tools are becoming more contextually aware. It can analyze the surroundings in the product image, such as background colors and other objects, to factor in how light might bounce around. This feature attempts to create a more unified, natural-looking scene, which in turn, can enhance consumer engagement.
The accuracy of the AI's lighting choices also comes from leveraging vast databases. AI systems analyze how light behaves on a range of surfaces and materials to make better brush decisions. The reliance on real-world data helps bolster the authenticity of the generated image, aiming to improve trust in the product representation.
This technology also opens up new avenues for customization. Brands might develop lighting profiles that align with their specific brand aesthetic. AI can then apply these profiles to their product images, creating a distinct visual language.
AI-powered lighting solutions are not just about aesthetic enhancement, they can also be a workflow booster. With this automated process, it’s significantly faster to explore different lighting scenarios during the creation of product images. Designers can experiment with various approaches without the tedious task of manual adjustments.
One interesting facet of this new approach is that it can be adaptive. AI models can analyze user-generated content to track current lighting and reflection styles used in e-commerce and adjust their outputs accordingly. This dynamic learning process helps ensure that the visual style of product images stays relevant and aligns with the latest trends in visual marketing.
Feedback loops play a key role in improving the accuracy of the AI models. By integrating user feedback and assessing the outcomes of previous lighting choices, AI can learn to refine its techniques.
While the ability to generate visually appealing product images is a significant boon for ecommerce, it raises questions about transparency. As AI image generation becomes more sophisticated, concerns about potentially misleading representations arise. The balance between enhancing a product's visual appeal and maintaining a level of ethical transparency will be crucial as this technology develops.
Identifying Digital Art Brushes 7 Key Techniques for Product Photo Enhancement in AI Image Generation - Digital Paint Stroke Analysis For Fabric Material Rendering In Adobe Firefly
Adobe Firefly's integration of digital paint stroke analysis introduces a new way to render fabric materials, which is especially useful for creating realistic and accurate product images in ecommerce. AI-powered features within Firefly help designers create detailed and believable fabric textures, which is crucial for showcasing the qualities of clothing or textiles online. Using tools like drawing tablets and styluses makes it easier to apply traditional painting techniques in a digital environment, potentially leading to more nuanced fabric textures. Furthermore, Firefly's ability to generate images from text prompts offers greater control in customizing the look of fabric, allowing designers to align with a specific brand's aesthetic or marketing campaign. In the ever-evolving landscape of online shopping, where competition is fierce and visuals play a critical role, tools like this might become essential for capturing customer attention with product imagery that truly stands out. However, there's a risk that relying too heavily on these tools might result in a loss of original artistic expression or an over-reliance on predictable styles, which could undermine the genuine appeal of unique product designs.
Adobe Firefly's integration of generative AI across creative tools like Photoshop and Illustrator provides a powerful platform for digital artists, particularly for those working on e-commerce product imagery. One intriguing area is its capability for analyzing digital paint strokes, which is proving useful for fabric material rendering. The AI can dissect different fabric textures in a very detailed way, leading to the creation of convincingly realistic images. The system goes beyond simply mimicking fabric, it can also adapt brush strokes in real-time to fit the chosen material. For instance, the AI will apply a different brush pressure when rendering rough canvas versus smooth satin, making the image more believable.
Beyond just texture, Firefly attempts to model how light interacts with fabrics. This includes realistically rendered shadows and highlights, features that really improve the impression of 3D form. This type of light simulation helps make the product images more visually convincing in the context of e-commerce where customers need to form an impression of a product based on a flat image. Interestingly, the speed and angle of the simulated brushstrokes play a role in the final visual appeal. Designers can fine-tune these elements to subtly change how fabrics look, for instance, adding a perceived sense of luxury.
Furthermore, the efficiency of Firefly's rendering process is noteworthy. The optimization algorithms make generating detailed fabric renders quite fast, which is essential for e-commerce applications where a quick turnaround on product images is important. Designers can interact with the system, providing feedback and tweaks in real-time, improving the precision of the fabric rendering. This is important if a company needs to maintain a strong visual brand identity across a range of products.
In addition to the more basic qualities of the fabric, the AI can also generate realistic-looking wear and tear, which can be valuable for creating images of vintage clothing or high-end garments. Firefly's paint stroke analysis integrates nicely with other AI tools in the Adobe ecosystem. This means designers can combine fabric textures with other elements, streamlining the process of crafting cohesive product presentations. The algorithm also leverages AI to make suggestions for color schemes. This feature analyzes color theory principles and offers up color palettes designed to enhance visibility and potentially improve the attractiveness of the online product. Lastly, the AI has the capacity to stay updated with current fabric trends. This adaptability is helpful for brands trying to create visual content that appeals to constantly changing consumer tastes.
However, while promising, these features do raise a few questions. Can this level of AI-driven aesthetic really significantly boost sales? There's still a degree of uncertainty around that, and we need to maintain a healthy skepticism of claims in this area. Also, there's a risk that over-reliance on AI could lead to product visuals becoming too similar, ultimately diminishing the role of creativity and design principles in e-commerce marketing. Further research and analysis will be necessary to fully understand the long-term impact of these AI capabilities on the way we perceive and interact with products online.
Identifying Digital Art Brushes 7 Key Techniques for Product Photo Enhancement in AI Image Generation - Custom Brush Development For Glass And Crystal Product Enhancement
Creating specialized digital brushes tailored for enhancing glass and crystal products is becoming increasingly important for ecommerce visuals. These custom brushes give artists the ability to produce detailed textures and reflections that mirror the natural properties of these materials, resulting in more lifelike product photos. With e-commerce increasingly depending on high-quality images to boost sales, the ability to skillfully use custom brushes is crucial for designers who want to make their products stand out. Not only do these custom brushes enhance the aesthetic appeal of product photos, but they also have the possibility of influencing customer perceptions, making them a powerful tool in digital marketing. However, it's important to consider that as the use of these sophisticated tools becomes more prevalent, the risk of uniformity in product imagery emerges. This can be problematic for brands who are striving to create a unique visual identity and stand out from the crowd. The future of e-commerce image creation requires a careful balance between adopting new tools and keeping a sense of originality in visual design.
The push to create custom brushes for enhancing glass and crystal products in online stores stems from the unique way light interacts with these materials. Accurately capturing reflections, refractions, and how light disperses is vital for making product photos look realistic, and specialized brushes are needed to pull this off.
Research suggests our eyes are really good at picking up subtle changes in light and surface imperfections. This means that brushes that can mimic these details aren't just about making the image look sharper; they can also make a big difference in how engaged a shopper is with a product, possibly leading to more sales.
When we use AI to create these brushes, we can incorporate concepts from physics, like Fresnel equations, which describe how light behaves when it goes through transparent materials. This allows us to generate images of glass textures that appear more genuine.
Specifically designed brushes for glass enable e-commerce sites to simulate complex light effects, like caustics. Caustics are the patterns you get when light bends as it passes through glass, and they can make product images more visually appealing, and technically accurate at the same time.
Studies show that a large number of consumers struggle to differentiate between images made by AI and actual photos. This underlines how important it is to use advanced brush techniques to improve product images, so they can compete with more traditional photos.
For products like gemstones or cut glass, custom brush libraries can use fractal algorithms to create incredibly detailed representations of the intricate crystal structures. This can significantly enhance the perception of luxury items when they are presented online.
Simulating light and shadows with custom brushes helps to create a sense of depth and three-dimensionality in glass objects. This can boost customer confidence in what they are seeing, especially since it's known that more authentic-looking product representations in e-commerce can result in a noticeable increase in purchases.
It's interesting to note that visual studies show that the clarity and detail in an image can strongly impact a consumer's feelings. So, using custom brushes to enhance the reflective qualities of glass can potentially affect how a person feels about a product.
Recent progress in neural networks is enabling the automated design of custom brushes that are specifically tailored to the surfaces of glass and crystal items. This shifts brush development from a purely artistic process towards one guided by data analysis.
However, it's important to maintain a high level of quality control throughout the development process, as variations in the quality of the data used can lead to inconsistencies in the final image. The inherent complexity of glass textures requires careful attention to detail to ensure the highest standard in product imagery.
Create photorealistic images of your products in any environment without expensive photo shoots! (Get started for free)
More Posts from lionvaplus.com: