Create photorealistic images of your products in any environment without expensive photo shoots! (Get started for free)
Blockchain-Powered GAN Technology Transforms AI Product Image Generation A Deep Dive into Playform's Genesis Projects
Blockchain-Powered GAN Technology Transforms AI Product Image Generation A Deep Dive into Playform's Genesis Projects - Neural Network Competition Drives GAN Image Quality for Ecommerce Products
The heart of GAN's success in generating ecommerce product images lies in the rivalry between its two neural networks. The Generator strives to create convincing product images from scratch, while the Discriminator acts as a discerning critic, attempting to differentiate between real and AI-generated images. This ongoing "competition" is the engine behind the improvement in GAN image quality. The training process becomes a continuous cycle, pushing the Generator to refine its output, striving to fool the increasingly astute Discriminator. This competitive dynamic has been further refined by improvements in convolutional neural networks, leading to more realistic and stable generated images.
However, as the use of AI-generated imagery expands, questions about the provenance and ownership of synthetic products images arise. Blockchain technology, with its promise of secure and transparent record-keeping, is being explored to address these concerns, providing a potential framework to ensure the authenticity of AI-generated content. This combination of adversarial learning and the potential integration of blockchain signals a change in how online retailers can visually present their products. Yet, this emerging technology presents challenges, particularly in determining how to evaluate the ethics of utilizing AI-generated images and defining clear ownership rights within the e-commerce ecosystem.
The core of GANs lies in a competitive dance between two neural networks: one, the generator, crafts images from random data, and the other, the discriminator, acts as a judge, trying to tell the difference between real and fabricated images. This ongoing battle is what drives the realism we see in GAN-generated ecommerce product images, pushing them towards photorealism and sometimes fooling even sophisticated computer vision systems.
This constant feedback loop in training is what allows GANs to rapidly generate product visuals that are comparable, and in some cases, exceed the quality of traditional photography. Notably, newer GAN architectures based on convolutional neural networks have brought about significant leaps in image quality and stability, producing sharper and more refined images than their predecessors.
Evaluating the effectiveness of a GAN often involves using specialized deep neural networks trained to assess aspects like image compression and perceived quality, much like how humans might judge visual appeal. These tools help researchers to objectively measure the progress made in GAN technology and tailor them to specific product types and aesthetic preferences.
While traditionally GANs required paired datasets, newer variations like CycleGAN and DualGAN have demonstrated capabilities in image-to-image translation using unpaired datasets, allowing for greater flexibility and efficiency in the generation process. The emergence of such techniques further underscores the evolving landscape of GAN applications in e-commerce.
There's an increasing need for methods that can efficiently generate high-quality data, particularly in deep learning where large datasets are critical for optimal model performance. GANs excel in addressing this need by creating realistic images from unlabeled data. The quality of these images, however, isn't without scrutiny. There are growing concerns around the transparency and ethics of using entirely AI-generated images in ecommerce, and this area requires further exploration to establish best practices and ensure customer trust in online product displays.
Blockchain-Powered GAN Technology Transforms AI Product Image Generation A Deep Dive into Playform's Genesis Projects - Blockchain Authentication Tracks Digital Asset Origins in Product Photography
Blockchain technology offers a way to track the origins and changes made to digital product images, a crucial development in the world of ecommerce, especially with the rise of AI-generated visuals. This technology creates a permanent, unchangeable record of an image's journey, providing a transparent history of its creation and any alterations. This is increasingly important as AI-generated product images become more prevalent.
The ability to trace a product image's history builds trust with customers by assuring them that what they see is authentic and hasn't been tampered with. This is particularly relevant for combating counterfeit products and manipulating product representations online. As both new and established businesses in ecommerce explore the use of blockchain for authenticating product images, the intersection of these technologies could fundamentally alter how authenticity and integrity are ensured in online product presentations. However, the integration of blockchain also raises questions about ownership and ethical considerations, highlighting the need for careful thought and deliberation within this rapidly evolving space.
Blockchain's ability to create a permanent and verifiable record of digital assets makes it a potential tool for ensuring the authenticity of product images, particularly those generated by AI. Imagine a system where every change or modification to a product image, even those created by a GAN, is recorded on a decentralized ledger. This could allow anyone to verify the origin of an image, essentially providing a sort of "digital fingerprint" that confirms its authenticity.
For example, in a scenario involving AI-generated product photos for an online store, the blockchain could record the steps involved in generating the image. This might include details of the GAN model used, any original photos or design elements that were part of the process, and even the specific individuals or teams involved in its creation. This level of transparency helps tackle issues surrounding authenticity and potential misuse of AI-generated imagery.
Moreover, blockchain can help establish clear ownership rights for AI-generated content, which is an important aspect given the increasingly blurred lines of authorship in this field. Smart contracts – self-executing agreements embedded in the blockchain – could potentially be used to automate licensing and royalty payments to creators of original content used within the generation process. This offers a way to address concerns around copyright infringement and ensure that the creators of such content get compensated fairly.
Of course, we need to consider the limitations. Integrating blockchain with AI-powered image generation still presents many technical and practical challenges. For example, how would we handle the enormous amounts of image data that are generated? How can we prevent the blockchain from becoming too slow or cumbersome due to the sheer volume of transactions related to these image datasets? These are important questions that need to be addressed for blockchain to truly become a viable solution for authenticating AI-generated product imagery.
Despite these challenges, the potential benefits are considerable. If implemented thoughtfully, this technology could enhance trust in AI-generated ecommerce product imagery, fostering transparency and helping to prevent any misleading depictions of products. Consumers could make more informed purchase decisions, leading to a higher degree of satisfaction and reducing potential returns, while retailers could streamline their processes and build more solid relationships with their clientele. This convergence of AI and blockchain in the world of online retail is still very much in its nascent stages, but it represents a fascinating and potentially disruptive innovation.
Blockchain-Powered GAN Technology Transforms AI Product Image Generation A Deep Dive into Playform's Genesis Projects - Automated Background Removal and Scene Generation Through Machine Learning
Automated background removal and scene generation, powered by machine learning, are transforming how ecommerce product images are created and presented. These methods allow for the precise separation of products from their original backgrounds, resulting in cleaner, more focused images ideal for online marketplaces. Beyond just removing backgrounds, these algorithms can now create entirely new environments for products, offering a greater level of control over the visual presentation. The rise of deep learning, particularly the use of generative adversarial networks (GANs), has led to significant improvements in image quality and realism. This has the potential to produce visuals that are virtually indistinguishable from traditional photography, offering a new level of flexibility for product visualization.
However, this increased capability also raises concerns about the authenticity and ethical implications of using entirely AI-generated product imagery. While the technology has clear potential for enhancing e-commerce, the need to maintain customer trust in the authenticity of these images is crucial. As we move forward, careful consideration must be given to the potential for misuse and the development of clear guidelines for ethical practices in AI-generated product visuals. The future of ecommerce product photography lies in a delicate balance between innovation and responsible application.
Within the realm of e-commerce product imagery, machine learning has enabled significant advancements in automating background removal and scene generation. These techniques, often relying on complex algorithms, can now efficiently isolate a product from its original background and seamlessly place it within new, synthetic environments.
Image pre-processing steps are crucial for ensuring the success of background removal tools. These tools are designed to prepare images in a way that optimizes the accuracy and quality of the final output after the background is extracted. Deep learning approaches have become increasingly important for handling this process, allowing not only for background removal but also the dynamic addition of entirely new background images, thereby opening up a broader range of creative possibilities within product visualization.
Generative Adversarial Networks (GANs) have emerged as a powerful tool for image generation in general, and specifically for creating realistic scenes and manipulating existing product images. Recent research has particularly focused on refining frameworks that utilize neural networks to achieve high-quality background removal, effectively pushing the boundaries of what’s possible in product image editing.
There's a distinction between autonomous and non-autonomous image generation, with the former focusing on completely generating images from scratch—think of computer-generated cartoons or abstract paintings. However, the more relevant application to product imagery leans toward non-autonomous methods, where we manipulate and refine existing visuals.
When it comes to the core process of removing a product's background, images are often segmented into classes, essentially categorizing pixels as either part of the foreground (product) or background. This allows the system to differentiate and effectively isolate the product for subsequent manipulation or placement in a new setting.
Interestingly, background removal techniques have evolved to include features that automatically identify salient parts of the product. This leads to a more robust and accurate separation, eliminating human error and promoting consistency in output. This is becoming increasingly vital in the e-commerce space, where a standardized output quality for AI-generated product images is essential across different product categories and sales channels. There’s a growing need for reliable and consistent image generation in order for consumers to build trust in AI-generated images as valid representations of products.
Blockchain-Powered GAN Technology Transforms AI Product Image Generation A Deep Dive into Playform's Genesis Projects - Real Time Product Angle Variations Using Advanced GAN Architecture
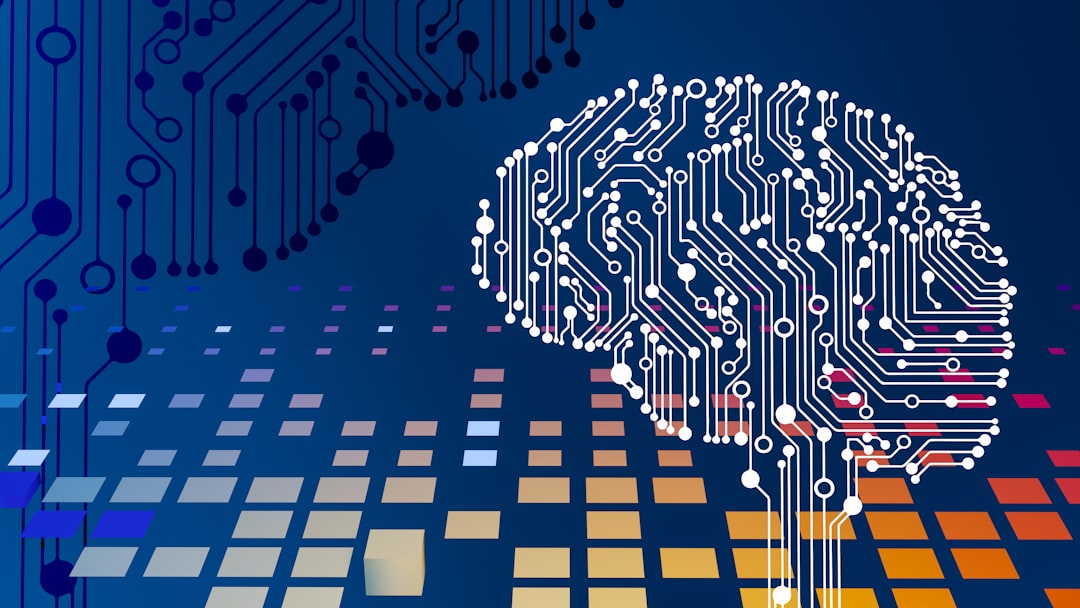
Advanced GAN architectures are now capable of producing real-time variations of product angles, a development significantly impacting the way e-commerce presents products. This allows for dynamic and diverse product presentations, offering consumers a more comprehensive view of a product before purchasing. They can see a 3D-like experience of the product from different angles through a series of images generated by the AI in real-time. However, this new ability to easily generate product views also leads to concerns. How much is too much AI-generated imagery? What is the line between helpful and misleading in how a product is presented online? The authenticity of images and the ethical considerations associated with presenting AI-generated visuals as genuine product depictions require a careful examination. Moving forward, maintaining customer trust while leveraging the benefits of these new AI capabilities will be crucial. Ecommerce platforms will need to develop strategies that balance creativity with clear disclosures about the use of AI in product image generation. If they can address the trust concerns and ethical use of AI-generated product images, it could signal a positive shift in online product marketing, particularly with the growing integration of blockchain technology.
In the realm of ecommerce, advanced GAN architectures are proving increasingly adept at generating variations of product angles in real time. This allows for quick adaptations to changing trends or promotional needs, potentially bypassing the need for multiple photo shoots. While this is efficient, it does raise questions about how the images are being created and whether they are really representative of the product in a genuine way.
The complexity of generated scenes is another area where GANs are shining. It's quite impressive how these models can create intricate backgrounds and settings that match a product's overall visual style. It's almost like they can build a tailored environment for the product to exist in, which in theory should boost a product's appeal. However, this capability could lead to some serious questions about authenticity and whether it can be sustained over time.
Interestingly, recent GAN architectures like CycleGAN are now able to generate images using unpaired datasets. This means they don't necessarily need perfectly matched pairs of images to work, which significantly broadens their application within e-commerce. We might expect to see a wider range of products having GAN-generated images in the near future. On the other hand, there could be concerns about how accurate the generated image truly is when a perfect comparison isn't readily available.
The quality of the images produced by GANs has experienced a major leap in recent years. The generated images can now attain a level of visual fidelity that surpasses many traditional product photographs. This is a significant shift, suggesting that GANs are no longer simply a novelty for producing images; they're beginning to actually outshine what was considered standard previously. But as this happens, questions arise about the future of traditional product photography, and also of how we will perceive the differences between traditional photography and AI-generated visuals in the future.
E-commerce businesses can now leverage GAN-generated images to do A/B testing, allowing them to experiment with different presentations of their products and see how customers respond. This is quite beneficial as they can tweak their marketing strategies based on real-time results from these tests. It remains to be seen whether this level of responsiveness will lead to faster innovation or potentially faster obsolescence as companies try to push the envelope on visuals.
We're also seeing specialized deep neural networks being developed to evaluate the quality of GAN-generated images beyond simply looking at the aesthetics. These tools now analyze things like sharpness and whether the image aligns with how humans typically perceive objects, helping to ensure a consistently high quality for e-commerce product catalogs. It's encouraging to see this focus on refining the quality assurance process for AI-generated imagery, but as with any new tool, it's important to evaluate whether the quality metrics are being applied ethically and fairly.
The integration of AI with traditional product photography is evolving. Convolutional neural networks can now streamline aspects of the photography process such as lighting and adjusting angles, reducing the time it takes to launch new products. This offers a huge advantage, potentially speeding up the overall development pipeline. This, however, presents potential challenges around jobs and skillsets in the future, and might further cement the divide between automated and skilled labour.
GAN-generated images have the ability to allow companies to market and sell products that might not yet exist physically. This gives companies some flexibility, enabling them to manage their inventory more strategically. The question though is whether there will be a negative impact on the buying experience, as shoppers may not feel the same way about a product they haven't been able to touch and interact with in person.
As GANs become more commonplace in e-commerce, consumer perceptions of product visuals are bound to change. Customers will likely become accustomed to higher quality imagery, forcing traditional photographers to adapt and explore how they can leverage new technology. This transition suggests the potential for a significant upheaval in the way product images are produced and what consumers expect from them. However, it's uncertain if a higher standard for visual representation equates to better products in terms of durability, usability, or safety.
Some of the more advanced GAN systems have started experimenting with the idea of combining features from different products into one image. This lets customers quickly compare options in a way that used to be much more time-consuming using traditional photography. This is a positive trend, but raises concerns around the potential for misleading or unrealistic combinations that might give a false impression of the product.
In conclusion, GANs are fundamentally changing the way ecommerce companies manage and showcase their product visuals. These advancements offer a range of new opportunities for improving user experience and streamlining the production process. However, along with these improvements come various ethical and practical challenges that need careful consideration. The ongoing development and refinement of GAN-related technology warrant close scrutiny, as it has the potential to impact a wide range of industries within the ecommerce landscape.
Blockchain-Powered GAN Technology Transforms AI Product Image Generation A Deep Dive into Playform's Genesis Projects - Zero Shot Learning Enables New Product Category Image Creation
Zero-shot learning introduces a new approach to AI product image generation, enabling models to create visuals for completely novel product categories without the need for specific training on those categories. This is achieved by essentially teaching the AI model to apply its knowledge about existing product types to generate images of unknown ones, guided by textual descriptions of the desired product. The combination of zero-shot learning with advanced techniques like Generative Adversarial Networks (GANs) makes this process even more powerful, allowing the rapid creation of realistic product images that previously would have been impossible.
While this technology promises exciting new possibilities for product visualization, it also raises questions about the authenticity and integrity of the generated images. There's a fine line between enhancing the customer experience with creative visuals and potentially misleading customers with images that may not truly represent a product. Transparency about how these images are created and ensuring customer trust in the authenticity of the images will be crucial as this technology becomes more prevalent. Therefore, while zero-shot learning possesses the potential to revolutionize product imagery, it's important to proceed with awareness of its implications and the necessity of building trust and understanding with consumers.
Zero-shot learning is a fascinating development in AI, particularly within the realm of image generation for ecommerce. It allows AI models, like GANs, to generate new product images even without being explicitly trained on that particular type of product. This is quite different from traditional AI training which requires huge amounts of data specifically related to the task. Essentially, these models can generalize knowledge they've acquired from training on a variety of images to create plausible visual representations of entirely new product categories. This holds tremendous promise for e-commerce, where brands could quickly generate diverse product visuals without the need for extensive image collections.
One notable application is in dynamic angle creation. Using advanced GANs, we can get a series of different viewpoints of a product almost instantaneously. It's like having a 3D model of a product, but only using a set of 2D images, each rendered at a different angle. This allows for far more interactive product experiences for customers. Interestingly, models like CycleGAN can do this without relying on perfectly matched pairs of images, which speeds things up considerably. However, there's the question of how much this impacts the buying experience – are these too good to be true, or will shoppers perceive them as misleading or inaccurate?
While this capacity for visual creation is impressive, the quality of the results is, naturally, crucial. It seems like the better the AI-generated image is, the more customers may respond positively, possibly leading to higher conversion rates online. There's a direct link between visual appeal and buying behavior, according to recent research. And as far as the actual process of image generation, features like background removal and scene generation can take product photos to the next level. It's now possible for AI to produce product images placed within highly stylized scenes, helping to showcase products within brand guidelines more effectively. To help assure consumers that the visuals are up to par, specialized AI systems have been built to judge the quality of the GAN-generated outputs, assessing things like clarity, color accuracy, and overall visual appeal.
The ability to rapidly adapt to changing market trends, marketing campaigns, or consumer preferences can be a real benefit for a business. By quickly tweaking images or generating entirely new sets of visuals in real-time, brands can respond quickly to market dynamics. But alongside these benefits come very real ethical considerations. The gap between AI-generated images and genuine product photographs is shrinking. This has led to concerns about misleading consumers and creating a lack of transparency around the product. How do we make sure that customers understand when they're looking at an AI-generated image and not a photograph? There's a definite risk that consumer trust can erode if they feel like they're being presented with misleading or deceptive images, no matter how high the quality of those images is.
The field of AI-powered product imagery is exciting but still under development. It's vital to strike a balance between embracing innovation and ensuring consumers feel confident about the product images they see online. While the ability to create a nearly limitless number of product visuals is captivating, the challenge remains to make sure this technology is used in a way that is beneficial and doesn't undermine the integrity of ecommerce platforms and online retail experiences.
Blockchain-Powered GAN Technology Transforms AI Product Image Generation A Deep Dive into Playform's Genesis Projects - Processing Speed Improvements Through Distributed Computing Networks
Distributed computing networks, built upon interconnected nodes with individual processing power, are accelerating the creation of AI-generated product images. This network structure allows for the rapid processing of complex tasks inherent in AI image generation, crucial for e-commerce environments needing quick results. The speed boost provided by distributed computing, coupled with blockchain's secure record-keeping, is key to producing and verifying authentic AI-generated product images in a timely manner. This speed advantage is undeniably beneficial, yet it raises ethical questions surrounding the ownership and reliability of these synthetic images, particularly as e-commerce becomes more dependent on AI-created visuals. Finding the right balance between the efficiency these networks offer and the potential ethical pitfalls they present will be crucial in determining the future of product images in online marketplaces.
Distributed computing networks offer a promising avenue for improving the speed and efficiency of AI-powered image generation, particularly for ecommerce applications. By breaking down the processing workload into smaller tasks spread across multiple interconnected nodes, these networks can tackle the computationally intensive demands of GANs. Imagine a scenario where the process of generating a product image is divided among several computers working in concert. This approach, while complex to orchestrate, can potentially unlock significant speed gains, especially when considering the ever-increasing complexity of GAN models.
One intriguing aspect is the potential to integrate quantum computing principles within these distributed networks. While still in its nascent stages, quantum computing has the theoretical capability to dramatically accelerate certain computational tasks. If successfully integrated, it might lead to a revolutionary leap in the speed of image generation and customization. Real-time adjustments to product images based on user preferences could become a reality, offering a dynamic and personalized experience for online shoppers.
Furthermore, the use of edge computing in conjunction with distributed networks can help to minimize latency in image generation. By pushing the processing power closer to the user, the time it takes for an image to be generated and displayed can be dramatically reduced. This is particularly important for e-commerce platforms that need to provide seamless and responsive user experiences.
Beyond speed, distributed networks contribute to improved system stability and resilience. Load balancing across multiple nodes ensures that the generation process is not bottlenecked by a single computer. This distribution also helps to mitigate the impact of outages, providing a more reliable platform for generating images, especially during peak shopping periods or when facing high demand.
Additionally, the decentralized nature of distributed computing networks provides opportunities for increased data privacy and security. When customer data stays local or is processed within the same regional area as the user, it reduces the risk of data breaches during image generation. The potential benefits for sensitive data, like purchase history or preferences, in this approach are significant.
The modular and scalable nature of distributed networks enables companies to flexibly adapt to changing demands. As e-commerce platforms grow, they can dynamically add or remove computing resources depending on need. This elasticity is crucial in managing fluctuations in demand without investing in significant infrastructure upgrades.
Distributed networks can also facilitate collaborative AI training environments. Multiple GAN models located across different geographical areas could potentially share and exchange information as they learn. This opens up exciting prospects for collective learning and accelerates the pace of GAN advancements, potentially resulting in product images with even more diverse styles and qualities.
However, achieving these benefits will likely require careful planning and execution. The complexities of managing distributed systems, along with the technical challenges of integrating them with GANs, should not be underestimated. Researchers and engineers will need to address issues like data synchronization, communication protocols, and robust error handling.
Despite these challenges, the prospects of leveraging distributed computing networks to enhance the speed and reliability of AI-generated images are compelling. As this area of research continues to develop, we may see a significant shift in how product images are presented and interacted with in the world of e-commerce.
Create photorealistic images of your products in any environment without expensive photo shoots! (Get started for free)
More Posts from lionvaplus.com: