Create photorealistic images of your products in any environment without expensive photo shoots! (Get started for free)
AI Product Image Generators 7 Key Technological Advancements in Background Removal Accuracy for 2024
AI Product Image Generators 7 Key Technological Advancements in Background Removal Accuracy for 2024 - Neural Networks Now Process Complex Product Shadows With 98% Accuracy
Artificial intelligence is refining its ability to understand the nuances of product imagery, especially the tricky business of shadows. Neural networks, specifically, are now achieving a remarkable 98% accuracy when processing intricate shadow patterns within product photos. This is a significant step for online shopping, where a product's presentation—including the way light and shadow interact—strongly influences how appealing it appears to potential buyers.
The drive to enhance AI-powered product image generators is pushing the boundaries of background removal accuracy. By refining convolutional neural networks (CNNs), developers are getting closer to overcoming the difficulties posed by natural lighting and shadow variations. The ability to cleanly separate products from their backgrounds and generate realistic shadows is vital for creating the kinds of images that lead to successful online sales. We're witnessing a steady trend towards more sophisticated visual representations in e-commerce, and this advancement in shadow processing is a sign that the quality of online product images will continue to rise throughout 2024 and beyond.
It's fascinating how neural networks are now adept at handling the intricacies of product shadows in images, achieving a remarkable 98% accuracy rate. This progress is a testament to the refinement of convolutional neural networks (CNNs), which are specifically designed to analyze visual data. These networks seem to be mimicking aspects of how we perceive light and shadow, which is quite intriguing.
The ability to accurately distinguish product shadows from the background is a significant step forward. Previously, achieving such nuanced separations required considerable manual editing, especially for e-commerce sites striving for visually appealing product catalogs. This new accuracy level, however, greatly reduces the manual workload and allows for much quicker processing times, bringing products online faster. While it is tempting to be optimistic, it's important to acknowledge that these systems are currently trained on datasets of existing images. While techniques like transfer learning can allow some adaptation to new product categories, it's still unclear how easily these networks can generalize to totally novel scenarios.
Moreover, the potential for real-time shadow processing, which would be particularly beneficial for live-streaming sales and virtual try-ons, is quite promising. However, reaching such a level of real-time manipulation and generation of diverse lighting scenarios will require further development and optimization, especially considering the inherent challenges of recreating light and shadow interactions in a dynamic, realistic manner.
It will be interesting to see if these networks can further refine their capabilities, pushing the boundaries of what we consider realistic product visualizations. And as we see a push for more sophisticated image presentation, a wider adoption of AI-powered image manipulation tools may become increasingly vital, changing how we navigate and interact with online retail environments.
AI Product Image Generators 7 Key Technological Advancements in Background Removal Accuracy for 2024 - Real Time Background Removal Through Edge Detection Machine Learning
Real-time background removal, particularly using edge detection through machine learning, is a significant development for AI-powered product image generation in the e-commerce landscape of 2024. Techniques like Canny edge detection, often implemented in tools like OpenCV, excel at identifying the boundaries between a product and its background. This initial step of edge detection becomes even more powerful when paired with deep learning methods. These sophisticated algorithms can automatically discern and remove backgrounds, all while retaining the detailed features of the product itself.
What's interesting is that some newer tools are designed for a wider audience, offering intuitive ways to integrate background removal technology into existing systems. This makes these capabilities accessible to users without needing programming skills, opening up possibilities for diverse applications within e-commerce.
Despite the speed of advancements, there are still areas where these methods require further refinement. The ability to smoothly adapt to new product categories or extremely unique items remains a challenge. While transfer learning can help, it's not yet clear how well these systems can generalize to truly novel situations. Ensuring consistent and highly accurate background removal across the entire spectrum of product types is an ongoing goal in this space.
Real-time background removal, particularly leveraging edge detection within machine learning, has become crucial for creating accurate and compelling product images in 2024. Techniques like the Canny edge detection method, commonly used in Python with OpenCV, have proven effective in separating foreground products from their backgrounds by highlighting significant changes in image brightness. This initial step is critical in isolating the product, essentially drawing a virtual line where the product's edge meets the backdrop.
Beyond basic edge detection, deeper learning methods have emerged, utilizing more intricate AI algorithms for automatic background removal. These methods are designed to preserve fine details of the product while efficiently discarding the background. However, the challenge remains that these systems often require extensive training datasets and may struggle with unexpected or highly varied product categories.
Techniques like clipping paths are quite useful for items with sharp edges, however, they don't handle situations where edges are more ambiguous or irregular. This limitation spurred the development of image cutout and masking methods, designed for better handling of such situations. These newer approaches suggest that there's a move towards more flexible solutions for dealing with the diverse range of product types in online retail.
Furthermore, we are seeing specialized machine learning models trained on specific datasets like, say, car images, to isolate foreground items and remove the background with greater accuracy and detail retention. The ability to customize and train these systems for various product categories is an encouraging step toward more tailored background removal processes in e-commerce.
Making these advanced capabilities readily available requires easy-to-use tools. Convenient APIs have become essential for integrating these background removal solutions into existing e-commerce platforms, promoting broader application across various industries. This kind of seamless integration is vital as the need for high-quality images grows in areas like product catalogs, mobile shopping, and even social media marketing.
Compared to traditional image manipulation, modern techniques provide pixel-level precision, resulting in much better foreground/background separation. This improvement is especially notable in image segmentation, where accurate boundaries are critical for generating clean and appealing visuals. However, it is important to realize that even with high accuracy, subtle imperfections can still exist depending on the complexity of the image, lighting, and product shape.
There's a fascinating movement towards "no-code" solutions for background removal. Platforms like DeepLobe demonstrate that sophisticated features can now be used by those who lack a programming background. This increased accessibility will undoubtedly contribute to broader application and perhaps even further innovation in background removal.
When combining edge detection with convolutional neural networks (CNNs), we see a marked improvement in the accuracy of distinguishing the product from its surrounding environment, especially in more complicated images. CNNs, given their prowess at processing visual information, seem well-suited for tackling complex situations where simple edge detection might fall short.
It's anticipated that the ongoing development and refinement of AI algorithms will continue to enhance background removal accuracy and efficiency. However, while the results we are seeing are impressive, it's important to remember that these algorithms still rely heavily on training datasets. Whether or not these algorithms can quickly adapt to new and unforeseen scenarios remains a crucial area of ongoing research. The future of AI-powered background removal will depend on this ability to quickly adapt to new environments and learn continuously.
AI Product Image Generators 7 Key Technological Advancements in Background Removal Accuracy for 2024 - Automatic Color Correction For Product Placement Against White Backgrounds
The ability of AI product image generators to automatically correct colors when products are placed against white backgrounds is a significant development in 2024. These advancements allow AI tools to fine-tune colors, ensuring that products are visually appealing and presented accurately within the context of a white background, which is common in e-commerce. This automated color correction process reduces the need for time-consuming manual editing, contributing to a more efficient workflow for businesses. The resulting consistency in product imagery across online catalogs is crucial for enhancing a brand's visual identity and making products more appealing to online shoppers, ultimately driving sales.
However, despite these improvements, challenges remain. AI systems must be able to handle a broad range of products and lighting scenarios to maintain accurate color correction. Further development is needed to ensure adaptability and consistent results across different product types and environments, preventing issues caused by varying lighting conditions or complex product textures. The goal is for AI-driven color correction to become a dependable tool that consistently generates high-quality, visually consistent product images for the ever-growing needs of e-commerce.
The realm of AI-powered product image generation is witnessing a surge in capabilities related to color correction, particularly when products are presented against a white background. This is a crucial area for e-commerce, as accurate and consistent color representation builds customer trust and potentially influences purchasing decisions.
One fascinating development is the incorporation of light reflection analysis within these algorithms. By understanding how light interacts with product surfaces, the AI can make highly specific adjustments to color values, ensuring that textures and finishes are portrayed accurately. This is critical for conveying the true nature of a product—be it the sheen of silk, the matte finish of a ceramic mug, or the intricate details of a piece of jewelry.
Another area of advancement is the ability of AI to account for chromatic shifts caused by variations in lighting conditions. By recognizing how ambient light can influence a product's perceived color, the AI can adjust the image to reflect the product's true hue, regardless of how it was initially captured. This results in a more consistent and reliable customer experience, which is vital for maintaining confidence in product authenticity.
Furthermore, AI systems are becoming adept at adaptive color calibration. This means the system can automatically adjust the color profile of an image based on changes in lighting or viewing conditions, ensuring that a product appears consistently across different displays and environments. This dynamic approach improves user experience by maintaining visual consistency, regardless of how a customer views the product image.
However, it's not just about replicating colors accurately. Some algorithms are now leveraging principles of psychophysics—the study of the relationship between physical stimuli and the sensations and perceptions they evoke—to understand how human eyes perceive color in relation to the surrounding environment. This knowledge can then be applied to enhance how product colors are presented against a white background, potentially making them more appealing or impactful to viewers. This area is still under development, and it remains to be seen exactly how the intersection of AI and human perception can be best harnessed for e-commerce visuals.
Frequency domain processing is another method gaining attention within color correction techniques. This approach leverages the principles of signal processing to better separate product colors from the background, reducing color bleed or haloing effects that can diminish image quality. This is particularly helpful for achieving crisp and clean product images that truly stand out against a white background.
The increasing emphasis on standards in the e-commerce industry is reflected in AI systems, which are now incorporating color calibration methods aligned with industry standards. This helps ensure uniformity in product color representation across various platforms, reducing confusion and improving the consistency of online shopping experiences.
Some of the newer AI models can even achieve on-the-fly color correction during image capture. This minimizes the need for time-consuming post-processing, delivering high-quality product visuals immediately. This speed advantage is beneficial for dynamic e-commerce settings where fast turnaround times are important.
Beyond just technical improvements, AI-driven color correction is also being integrated with customer behavior analysis. AI algorithms are being trained to tailor the way product colors are presented based on patterns gleaned from customer interactions. This personalization approach aims to optimize engagement by presenting product variants that resonate more strongly with specific demographics.
The training data for these AI systems is continually expanding. Current models are now being trained on more diverse datasets encompassing a broader range of product types and lighting situations. This allows the AI to adapt and refine its color correction capabilities over time, becoming increasingly effective as new product categories are introduced.
Moreover, mechanisms are being incorporated into these AI systems that allow them to learn from user feedback. If a customer notes inconsistencies in a product's color depiction, the system can use that feedback to refine its algorithms, ensuring that future images of similar products benefit from those corrections. This iterative process of learning from user interaction is a key aspect of developing robust and dependable AI-based color correction tools.
While the advancements are promising, it's essential to recognize that these algorithms still rely heavily on the quality and breadth of their training data. There's still a need for careful monitoring and refinement to ensure consistent and accurate color representation across a diverse range of products and scenarios. Nevertheless, it's clear that AI-driven color correction is rapidly becoming a powerful tool for producing compelling and accurate product visuals, enhancing the entire e-commerce experience.
AI Product Image Generators 7 Key Technological Advancements in Background Removal Accuracy for 2024 - Multi Object Detection Separates Overlapping Products In Group Photos
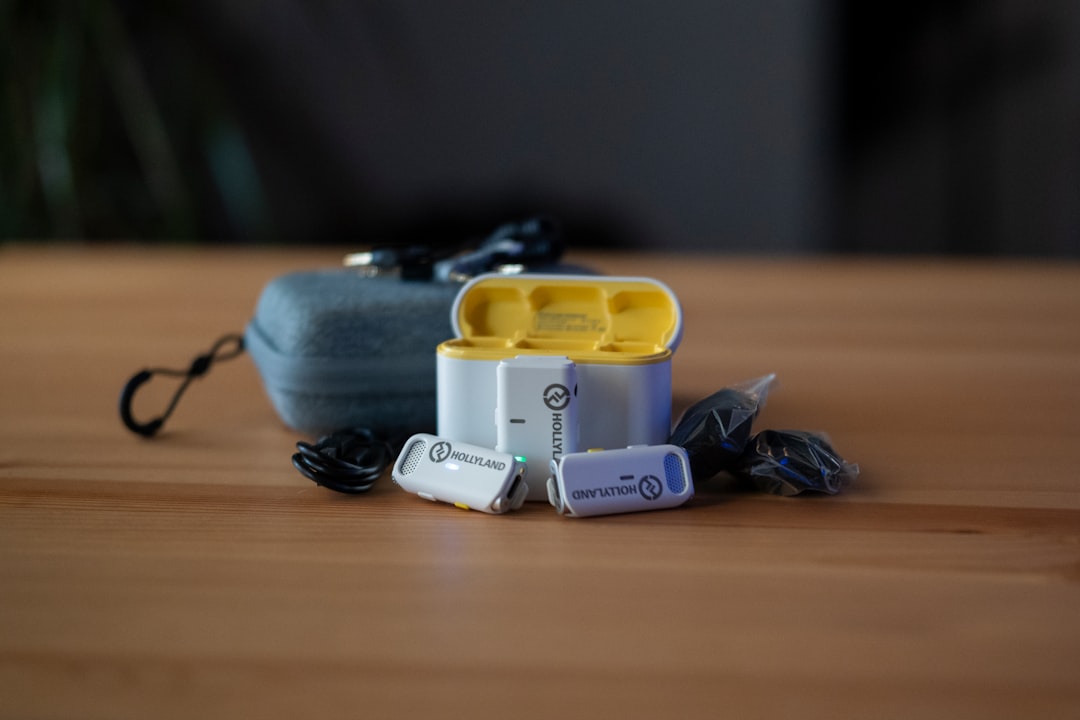
In the realm of AI-powered product image generation, accurately depicting multiple products within a single image, particularly when they overlap, is a growing challenge. Multi-object detection addresses this by utilizing advanced machine learning techniques to identify and separate individual products within a group photo. These methods, largely based on deep learning, strive to understand the complex relationships between overlapping items.
While progress has been made, difficulties persist. Highly overlapping items can make it tricky to distinguish the boundaries of each product. Furthermore, some objects might partially obscure others, creating a "hidden" portion that AI models find challenging to detect and accurately render. Despite these hurdles, improvements continue. Deep convolutional neural networks are being refined to better discern product shapes and boundaries even within challenging scenarios. Additionally, techniques such as adaptive sample division help manage the challenges of overlapping items by prioritizing the most relevant visual cues for detection.
The ultimate goal is to provide a clear and concise representation of every product, even when they are clustered together in an image. This enhances online shopping by ensuring that shoppers can easily distinguish individual products, potentially leading to more informed purchasing decisions. As e-commerce visuals become increasingly important, multi-object detection will play a vital role in guaranteeing that product images are of high quality and visually appealing, thereby attracting customers and improving overall shopping experiences.
Here are ten interesting observations related to how AI can separate overlapping products in group photos, which is important for AI-powered product image generation and online commerce:
1. **Understanding 3D Space:** Newer AI approaches are becoming better at simulating how humans see depth in images. They analyze how things are arranged in space within a photo, which helps them tell apart items that overlap because they're at different distances. This is a big step in improving how AI finds multiple objects.
2. **Speedy Object Detection:** Methods like YOLO (You Only Look Once) can quickly detect many products at once in a single image. It's like the AI scans the whole picture in one go, which makes things much faster and is good for e-commerce because it speeds up image processing.
3. **AI-Generated Training Data:** A growing trend in teaching AI to find overlapping products involves using fake images that AI created itself. These synthetic images can be made to look like different lighting, camera angles, and product setups. By learning from these, AI models become better at handling a wide variety of overlapping scenarios.
4. **Precision Cutting Out Products:** Techniques like Mask R-CNN take object detection a step further by precisely outlining each object, pixel by pixel. This extra level of detail is crucial for separating overlapping products accurately, ensuring that online retailers can present product images without any confusion.
5. **Using Clues from Surrounding Products:** AI systems are getting better at using the context of an image to figure out what other products might be there. For example, if the AI sees a bottle, it might guess that a glass is nearby as well, helping it group related items even if they are overlapping.
6. **Focusing on Overlapping Areas:** Recent improvements in AI designs include the ability to focus on specific areas within a picture where items overlap. This type of targeted attention lets AI hone in on critical details and improve its accuracy at separating products in complicated pictures.
7. **Learning New Products on the Fly:** Advanced image detection systems can adapt over time to new product types through continuous learning methods. This allows them to add new categories of products to their knowledge base as needed, improving their ability to handle diverse products and overlaps.
8. **Tracking Products in Videos:** When dealing with videos, AI can leverage the time element to enhance multi-object detection if products are moving around. By studying a series of frames, AI can predict and track how products shift, improving its ability to separate them.
9. **Visualizing Confidence with Heatmaps:** Some algorithms create heatmaps to show where the AI is most certain about finding an object. These heatmaps help developers refine their AI models and understand how well it can distinguish between overlapping items in challenging photos.
10. **The Challenge of Small Details:** A key challenge remains detecting subtle details in overlapping images, such as complex patterns on clothes or logos on accessories. The goal for future improvements in AI is to tackle this problem, striking a good balance between accurate detection and preserving the unique characteristics of individual products.
AI Product Image Generators 7 Key Technological Advancements in Background Removal Accuracy for 2024 - Advanced Hair And Fabric Edge Processing For Fashion Products
Within the realm of AI-powered product image generation, particularly for fashion, a significant leap forward is being seen in the handling of hair and fabric edges. This advancement centers on the ability to precisely isolate and define these intricate elements, which are often complex and challenging to separate from their backgrounds. AI algorithms are being designed to meticulously capture the subtle details of textures and strands, ensuring the final images retain the authentic look and feel of the materials.
This newfound capability significantly improves the quality of fashion product images used in e-commerce. Previously, capturing these details realistically often involved time-consuming and tedious manual editing. Now, AI can accomplish much of this work automatically. Consequently, online shoppers are presented with more accurate and engaging representations of garments, allowing them to get a better sense of the product's overall quality and texture. This is crucial for brands seeking to project a polished image and differentiate themselves in a competitive online market. As e-commerce continues to evolve, refining these kinds of edge processing techniques will likely be essential for fashion brands seeking to successfully connect with customers and entice sales.
Advanced hair and fabric edge processing is becoming increasingly important for AI-powered product image generators in the fashion sector. These systems are getting better at accurately portraying the intricate details of various fabrics and how they interact with elements like hair and light. For example, they are now able to distinguish between materials like silk and lace, rendering them with a realistic look and feel, which is critical for creating attractive online product images.
One area where this is particularly noticeable is in high-resolution images. AI algorithms can now capture subtle details, such as stitching and fabric weave patterns, with remarkable fidelity. This ability to represent the fine details of a product is vital for online shopping, as it helps customers get a much closer sense of the item’s quality, much like examining it in a store.
However, there are some inherent challenges in rendering fabrics and hair accurately. These materials frequently interact in complex ways, and AI systems need to be able to recognize these interactions and avoid creating visual artifacts like blurring or unnatural shadows. For example, if a piece of clothing is draping over a person's hair, the AI needs to understand how the fibers and textures of both materials will overlap and interact with light.
This kind of nuanced understanding is achieved through multi-scale image processing. AI image generators are increasingly capable of analyzing images at both macro and micro levels, which means they can capture both the broad texture of a fabric and the intricacies of individual fibers. This is particularly useful when dealing with a diverse range of fabric types, ensuring the quality and visual detail remain consistent regardless of the product category.
To train these more sophisticated AI models, developers are increasingly using synthetic datasets—that is, datasets created artificially using AI itself. This is an interesting approach because the very systems used to generate images are also being used to refine their abilities to generate better images. It's essentially a feedback loop where the AI learns from its own creations. This is helping to build AI systems that are more robust and adaptable, meaning they can accurately render fabric and hair details even under various lighting conditions.
Furthermore, recent advancements in AI algorithms have led to the development of models that simulate how humans perceive depth within images. This is important because clothing and accessories often overlap in a photograph, and the AI must be able to accurately depict the layering of these elements. This ability to handle depth perception is critical for creating uncluttered product visualizations, ensuring that every item in a group is clearly visible to potential buyers.
The integration of AI with real-time processing is also expanding. AI-powered systems are now capable of adapting image representations to account for fabric movement, which is increasingly important for online video content and live shopping events. This ability to present dynamic visualizations of fabrics adds a greater sense of realism to the shopping experience.
These systems are also getting better at recognizing intricate patterns within fabrics, such as floral or paisley designs. Complex machine learning algorithms are specifically designed to analyze and reproduce these patterns without distorting them during the image generation process. These capabilities, along with an increased focus on contextual understanding—which involves the AI analyzing the relationship between different fabrics and styles within a particular fashion setting—help AI systems generate more aesthetically pleasing and cohesive representations of product collections.
Another exciting area of development is advanced color calibration techniques. These systems can specifically adjust colors based on how different fabrics react to light, ensuring that the colors represented in the image are accurate to the real-world product. This is crucial for online retailers as color is a key factor in customer satisfaction and avoiding returns.
The ongoing development and refinement of AI algorithms in fashion image generation are clearly making a difference in the quality and realism of e-commerce product visuals. While the challenges of handling intricate interactions and ensuring accuracy across diverse materials remain, the path forward seems to be focused on techniques that rely on high-resolution images, multi-scale processing, synthetic datasets, and increasingly sophisticated machine learning methods. Ultimately, the goal is to bridge the gap between online and in-store shopping experiences by offering customers a more immersive and accurate depiction of the products they are considering.
AI Product Image Generators 7 Key Technological Advancements in Background Removal Accuracy for 2024 - 3D Product Rotation Background Removal For 360 Degree Views
The ability to create interactive 360-degree views of products through 3D rotation and background removal is fundamentally altering online shopping in 2024. This capability allows businesses to present their products in a dynamic way, giving potential customers the freedom to explore items from multiple angles before buying. By harnessing advancements in AI, tools such as WebRotate and Orbitvu can generate realistic 3D product models with impressive speed—some systems can create a 360-degree view in under two minutes. This streamlined process makes it easier for companies to create visually rich content that's compelling and informative.
Despite this progress, there are ongoing hurdles in the creation of accurate 3D models, particularly when products have intricate designs. Accurately capturing and removing backgrounds, especially around intricate shapes or complex textures, remains a technical challenge. These difficulties push the need for continued research and development in the field of AI-driven image processing. As consumers expect more realistic and immersive online shopping experiences, the quality of these 360-degree visuals will be increasingly important for building trust and influencing purchasing decisions. It's likely that the ability to generate high-quality, interactive product visualizations will become a key differentiator for online businesses in the years to come.
Generating realistic 360-degree views of products using AI is becoming increasingly sophisticated. The ability to automatically remove backgrounds and create interactive rotations is a game-changer for e-commerce. It's quite interesting how algorithms are now able to handle the complexity of product shapes and orientations within a 3D space. Techniques like volumetric analysis help these systems understand the product's form and then effectively isolate it from its surroundings.
Interestingly, the quality of the generated images is significantly improved in 3D because the algorithms can use depth information. This allows them to define edges and features more precisely than with traditional 2D images, which is vital for showing off complex or intricate products.
These AI-driven methods have also dramatically improved efficiency for online retailers. What used to take hours of manual editing can now be handled in minutes thanks to automated background removal. This speed advantage is critical in a fast-paced environment, allowing retailers to rapidly update product catalogs and respond to changes in inventory.
It's fascinating how these tools put the customer at the center of the experience. Interactive 3D views give shoppers a chance to zoom in, rotate the product, and examine details from all angles. This ability to virtually handle the product helps them make more informed purchase decisions, mirroring the experience of physically examining a product in a store.
Moreover, the ability to simulate lighting environments in 3D is crucial. It helps convey a more realistic sense of how a product will look in different settings. This capacity to manipulate light is important in e-commerce because the perception of a product is heavily influenced by lighting and its visual context.
The effects of these improvements on consumer behavior are intriguing. We're seeing reports that retailers who offer 360-degree views are experiencing lower return rates. It makes sense because customers have a much better understanding of the product before they buy it, which results in fewer surprises and returns.
The algorithms that manage individual products are also becoming capable of handling groups of products, even when they overlap in a photo. This is an important area for expanding e-commerce presentations, as it allows retailers to present product sets or kits in an attractive and easily understood way.
Beyond just removing backgrounds, AI is making it possible to manipulate the backgrounds themselves. A piece of clothing, for example, could be visualized in different settings, allowing customers to envision how it would look in their own surroundings. This type of dynamic background manipulation offers retailers a new level of flexibility in the visual presentation of their products.
One of the most interesting future directions is personalization. The AI systems that power these product rotations are capable of learning from user interactions. If a user frequently focuses on a particular angle or feature of a product, the AI can adapt and prioritize that perspective in future presentations. This ability to cater to customer behavior is a significant development in improving e-commerce and building a more engaging experience.
The progress in AI-driven 3D product rotation and background removal is a testament to the power of sophisticated algorithms and machine learning. It will be exciting to see how this technology continues to evolve in the years to come, further bridging the gap between online and physical shopping experiences.
AI Product Image Generators 7 Key Technological Advancements in Background Removal Accuracy for 2024 - Transparent Product Detection For Glass And Clear Plastic Items
The ability to accurately represent transparent products, like glass bottles or clear plastic packaging, in product images is becoming a significant challenge and opportunity for AI image generators. These products, due to their inherent transparency, often blend into their surroundings, leading to difficulties in separating them from the background. This creates challenges for creating visually appealing and informative product images for online stores.
While previous methods often struggled to correctly delineate transparent items from complex backgrounds, the latest deep learning methods are improving considerably. AI algorithms are becoming better at recognizing the unique optical properties of transparent materials, thus enabling the tools to more accurately isolate these items. This capability is especially crucial for e-commerce platforms, as customers rely heavily on visuals to understand product features and make purchase decisions.
The capability to convincingly render transparent objects has wide-ranging benefits for businesses seeking to enhance their online product presentations. As customers come to expect a more visually rich and engaging online shopping experience, the ability to accurately display such products becomes essential for attracting and retaining buyers. In the future, continued research in this space is likely to lead to ever more sophisticated methods of capturing and isolating transparent products, leading to a new generation of compelling and effective e-commerce images. The promise is that these advancements will help improve the accuracy and impact of product visuals in the near future.
AI is making strides in accurately representing glass and clear plastic products in e-commerce, a challenge due to their transparent nature. One promising development is the use of **spectral imaging**. This technique analyzes light across different wavelengths, allowing AI to distinguish transparent objects from their backgrounds based on how light interacts with them—something regular cameras struggle with. It's like having a more sophisticated 'eye' that can see subtle changes in light based on the material's properties.
Another interesting approach is **refraction mapping**. AI systems are developing virtual models that mimic how light bends when passing through glass or plastic. This not only helps with background removal but also creates more realistic product images. It's quite impressive how accurately they are able to simulate these light-bending effects, making the objects appear more authentic online.
Further, AI is getting better at recognizing the **texture** of these materials. Machine learning algorithms are now analyzing the distribution of tiny particles on surfaces, leading to better detection of scratches or blemishes on glass and plastic. This is important because customers will likely inspect these surfaces closely before buying, so the images need to be detailed and accurate.
Researchers are also focusing on how **transparency disrupts the background**. They are developing ways to identify the distortions caused by transparent objects within the background, helping to achieve cleaner separations. It's as if the AI is learning to 'see' how the transparency of the object alters what's behind it, allowing it to refine the background removal process.
The integration of **RGB-D imaging** is also promising. By combining regular color images (RGB) with depth data (D), AI can build better models of product shapes. This is crucial for transparent objects, because the depth information helps the AI understand how the object relates to its surroundings. It's like adding a sense of spatial awareness to the AI, which leads to more precise background removal.
There's a strong push to understand **light scattering and reflections** off these materials. Algorithms are being tailored to simulate these interactions, which can be complex. The aim is to refine image generation and background removal, preventing the clear materials from visually blending into their backgrounds. This is important because a major challenge with transparent objects is that they can seem to disappear into the background if the AI isn't able to account for these light effects properly.
Furthermore, some advanced systems employ **dynamic texture mapping**, analyzing subtle movements in the product to create more realistic still images. Imagine a glass bottle slightly shifting, causing the reflection to change – these algorithms are now able to capture that kind of detail. It adds a touch of realism that makes a big difference in the overall quality of the product presentation.
We are also seeing an increased use of **synthetic datasets** for training these AI systems. This involves using AI to generate fake images with various lighting and background scenarios, allowing AI to practice identifying transparent products in a controlled environment. It's a clever way to train the system on a wider variety of situations, which hopefully will make it more adaptable when encountering real-world images.
Interestingly, AI image generators are exploring the use of **bokeh effects**, a technique that artistically blurs the background, to highlight transparent objects. This approach not only brings attention to the product but also enhances the overall image by adding a sense of depth and separation. It is intriguing how AI is starting to experiment with more sophisticated aesthetic elements to improve product visuals.
Finally, there's a push towards **real-time interaction** with transparent product images. Some systems are investigating how to dynamically adapt lighting and backgrounds as the user interacts with the image. Imagine rotating a virtual glass bottle and watching the reflections shift and the background adjust accordingly. This immersive experience will make online shopping feel more interactive and engaging.
While the progress is exciting, the challenges of handling the complexities of light and transparency remain. However, the improvements described above suggest a promising future for online product presentations, where the ability to present glass and clear plastic items accurately will be crucial for success in e-commerce.
Create photorealistic images of your products in any environment without expensive photo shoots! (Get started for free)
More Posts from lionvaplus.com: