Create photorealistic images of your products in any environment without expensive photo shoots! (Get started for free)
AI-Powered Product Image Generation Leveraging Quantized LLMs for Efficient E-commerce Visuals
AI-Powered Product Image Generation Leveraging Quantized LLMs for Efficient E-commerce Visuals - Quantized LLMs Streamline Product Image Creation for E-commerce Platforms
Quantized LLMs are changing how e-commerce platforms create product images by making the process more efficient and less demanding on resources. By significantly reducing the memory needed to run these large language models, they open up new possibilities. For instance, automatic product descriptions can be generated from images, accelerating the process of adding new products and potentially reducing the need for manual work. Methods like QLoRA demonstrate how powerful language models can be customized for e-commerce, without requiring excessive computing power. Recent progress in image generation, with models like FLUX1, shows that we might see even higher quality images and better adherence to descriptions. In the bigger picture, quantized LLMs have the potential to boost the visual quality of online stores and improve the user experience through things like more relevant product suggestions, thus improving usability and perhaps even boosting conversions.
1. The reduced memory footprint of quantized LLMs offers a compelling advantage for smaller e-commerce businesses. They can potentially create high-quality product visuals without needing the immense resources typically associated with sophisticated image generation, leveling the playing field against larger competitors.
2. It's fascinating how quantization methods have progressed. While converting model weights to lower precision, they still retain a remarkable level of performance, suggesting we might be able to optimize these models further without sacrificing too much quality in image generation.
3. The ability to automatically adjust product staging with AI opens up exciting possibilities. We can potentially experiment with various backgrounds and scenes for the same product within a single shopping experience, offering greater flexibility for customers and perhaps appealing to broader preferences.
4. The prospect of real-time product image customization based on user interactions through integrated quantized LLMs is really intriguing. This might blur the line between traditional e-commerce and personalized shopping, allowing retailers to dynamically tailor visuals based on a shopper's unique characteristics or history.
5. The idea of using quantized LLM-driven algorithms to predict the best-performing product images is very compelling from a business perspective. If it can be accurately done by analyzing historical data, it potentially allows for highly targeted marketing efforts and could help maximize sales.
6. The speed at which quantized LLMs can generate product image variations opens doors for A/B testing on a massive scale. We can finally start to glean very nuanced insights into consumer preferences related to visual elements, potentially finding patterns that were hard to capture with traditional methods.
7. Creating images that simulate a physical retail environment by automatically adjusting lighting using quantized LLMs could be a great way to build trust with online shoppers. It adds a degree of realism that might make customers feel more confident in their purchases.
8. Quantized models offer the potential to generate product images with exceptionally realistic textures and materials, minimizing the post-processing steps that can be a major time sink in product staging. This simplification could be quite beneficial for efficiency.
9. The ability to show different variants of a product (like color, size, or style) within a single image using AI is an interesting development. It provides a more comprehensive view of available options to shoppers, streamlining the browsing experience and reducing the need for multiple, potentially costly photoshoots.
10. One of the more practical implications of using quantized LLMs in e-commerce is the ability to significantly reduce the time it takes to launch new product lines. By generating images quickly, companies can potentially cut down on weeks or even months of traditional preparation and photoshoot time, helping them react more quickly to market trends and customer demands.
AI-Powered Product Image Generation Leveraging Quantized LLMs for Efficient E-commerce Visuals - AI-Driven Automated Post-Processing Enhances Product Photo Quality
AI is injecting a new level of refinement into product photography for online stores. These systems are capable of automatically enhancing image quality by subtly adjusting factors like color balance, contrast, and brightness. The result is a more visually engaging product presentation, capable of capturing a shopper's attention more effectively. Previously labor-intensive and time-consuming post-processing steps, like precise cropping and complex lighting adjustments, can now be handled automatically, freeing up resources and potentially speeding up the process of getting new products online. The automation not only reduces the burden on teams but also fosters consistency, ensuring all images in a catalog maintain a similar visual style and quality, creating a polished overall brand experience. In essence, AI-driven post-processing acts as a powerful tool to elevate the quality of online product photography, boosting the potential for converting window shoppers into customers. While the technology may seem to simplify the process, there's still a need to make thoughtful choices about which tools are best suited for a given product and e-commerce environment. There's a risk of losing the distinct aesthetic or style of a brand if AI is not thoughtfully integrated. It's essential for businesses to experiment and fine-tune these techniques to ensure their visual language still resonates with their target audience.
AI-driven automation is increasingly being used to refine product photos after they're initially captured. These systems can now automatically enhance the sharpness and clarity of images, often outperforming traditional editing methods that rely on manual adjustments and a photographer's experience. While this is interesting, I wonder if there's a risk of images becoming overly processed and losing some of the unique characteristics of a product.
AI's ability to swiftly remove unwanted backgrounds from images is a huge time saver. It promises to significantly reduce the labor and costs involved in preparing product photos, which will likely streamline operations. However, it's important to ensure the automatic background removal doesn't compromise the image quality, and maybe we need to watch for any unusual artifacts these AI algorithms might introduce.
Image enhancement algorithms driven by AI have the potential to significantly boost the visual appeal and clarity of details in product images. This could ultimately reduce the number of returns online retailers face due to customers finding the products don't look as good in person as they did in the online photo. It's a promising avenue for improving customer satisfaction, but it'll be important to track return rates in detail to assess whether this truly reduces customer dissatisfaction.
Interestingly, AI can also analyze how customers interact with product images to determine which visual styles resonate best. By examining things like click-through rates and conversion rates, AI could help companies make better decisions about their visual marketing approaches. I'm curious to see how these techniques develop, as it could lead to incredibly tailored shopping experiences for individual consumers, but there are also privacy concerns we need to address if the system is capturing data on our choices.
Generative adversarial networks, or GANs, are increasingly being used to create variations of product images without requiring extensive photography or retouching. These AI systems can quickly generate multiple versions of an image, helping to optimize for visual appeal without having to take a ton of new photos. The effectiveness of this approach will depend on the underlying datasets used to train the models, and we have to make sure that the GAN outputs don't look artificial.
One interesting application is that AI can dynamically adjust product images in real-time based on how a shopper is interacting with the webpage. It might alter the lighting conditions, the product angle, or even incorporate different backgrounds. This sort of personalized visual experience might be very appealing to consumers. But I worry that it could push us into an era where customization goes too far, and we start to see highly engineered shopping experiences that might stifle genuine discovery of new products.
We can now achieve much more with high-dynamic-range (HDR) imaging thanks to AI post-processing tools. These tools help product photos display a wider range of colors and contrast, making them visually richer. It's definitely improving image quality, but it's worth paying attention to whether these enhancements might also artificially distort colors or make them look too vibrant for realistic representation.
By analyzing massive amounts of data on product images and shopper behavior, AI systems can now predict which visuals are likely to perform best in marketing campaigns. This could enable e-commerce companies to optimize their visual marketing efforts with incredible precision. While this is a powerful tool for companies, we must carefully consider the implications of AI driving these types of marketing decisions. What will the human element in creativity and design look like in this future?
Studies suggest that AI-enhanced product images can give the impression of higher quality, affecting how consumers perceive both the product and the brand associated with it. This could have a significant impact on how businesses establish brand image and perception. I wonder if this leads to a standardization of aesthetics that becomes overwhelming or if it opens doors to new forms of creative experimentation within visual product design.
Automated post-processing can also be used to integrate multiple usage scenarios within a single product listing. For example, we could see product images showcasing items in a range of settings, combined with images featuring accessories or how the product is used. This improved ability to visualize a product's use in context could help buyers create a deeper connection with the product and better understand its functionality. I wonder whether the increasing level of detail in online images might lead to a change in how customers perceive the need to physically touch and examine products prior to purchase.
AI-Powered Product Image Generation Leveraging Quantized LLMs for Efficient E-commerce Visuals - Large Language Models Enable Intelligent Product Image Captioning
Large language models (LLMs) are increasingly used to generate captions for product images, a process that's becoming more sophisticated thanks to advances in both computer vision and natural language processing. These models can analyze an image and then create a text description, essentially automating a task that was previously done manually. The ability to combine visual analysis with natural language generation is key, allowing for more informative and engaging product descriptions.
While this approach can certainly improve the quality of product image captions compared to generic, pre-written ones, it still faces challenges. LLMs, despite their capabilities, sometimes struggle to capture the finer details of an image and can still generate somewhat generic descriptions that don't fully convey the specifics of a product. There's a growing focus on techniques to create richer and more accurate captions, where LLMs go beyond simply describing what's in a photo and attempt to encapsulate the true nature and significance of the product.
The prospect of LLMs becoming a more integrated part of e-commerce visuals is exciting. They could help create more user-friendly online shopping experiences by ensuring that product information is presented in a way that aligns with the visuals, potentially increasing both understanding and customer engagement. However, it's crucial that the field keeps addressing the limitations in the current LLMs to ensure they don't simply create generic descriptions, instead generating detailed, engaging captions that accurately describe the product and potentially increase sales and conversions.
Large language models (LLMs) are increasingly being used to automatically generate captions for product images, bridging the gap between visual and textual information within e-commerce. This is a fascinating development in the field of computer vision and natural language processing, as it lets us explore how AI can understand and describe images in ways that resonate with shoppers. LLMs trained on vast datasets of product images and related text can learn to generate captions that are not only descriptive but also tailored to the specific context of the image, the product, and potentially even the individual customer.
The potential of LLMs in image captioning has been enhanced by recent advancements in vision-language pretraining (VLP), which uses huge datasets of image-text pairs to improve a model's ability to generate relevant captions. However, there are still challenges; some LLMs tend to create generic captions that might miss important details about the image or the product it shows. Techniques like FuseCap aim to address this limitation, pushing LLMs to generate captions that are more nuanced and insightful. Notably, models like BLIP2 demonstrate zero-shot image-to-text generation, allowing us to create captions without extensive pre-training, making the technology more accessible for various applications.
The larger trend here is toward automation within e-commerce. We see generative AI methods emerging in enterprise settings for tasks like automating the creation of image descriptions and powering image search functionalities. This is a significant shift from earlier captioning methods that were mostly manual or involved simpler neural networks. It's important to remember, though, that limitations in the training datasets can create obstacles for these models. If the datasets don't contain comprehensive descriptions, the AI might struggle to create accurate or truly engaging captions. For example, training data might lack specific details about product features or fail to capture subtle nuances of product design.
This intersection of natural language and computer vision in e-commerce is exciting. It opens the door for a richer shopping experience, potentially improving conversions by generating more informative captions that help shoppers understand a product's characteristics. Additionally, we can start envisioning multilingual product descriptions and tailored marketing content driven by the same AI systems, increasing a business's ability to reach global markets. While promising, it's worth keeping in mind the trade-offs involved. As we strive for more efficient captioning, we need to ensure that brand identity and voice remain consistent. The push for automation shouldn't lead to bland, generic descriptions that are devoid of the unique qualities that make a brand appealing. The future of automated captioning is clearly tied to the ongoing evolution of LLMs and the availability of large-scale image-text data, so we'll continue to see refinements and improvements over time.
AI-Powered Product Image Generation Leveraging Quantized LLMs for Efficient E-commerce Visuals - Generative AI Produces Dynamic Product Image Sequences for Enhanced UX
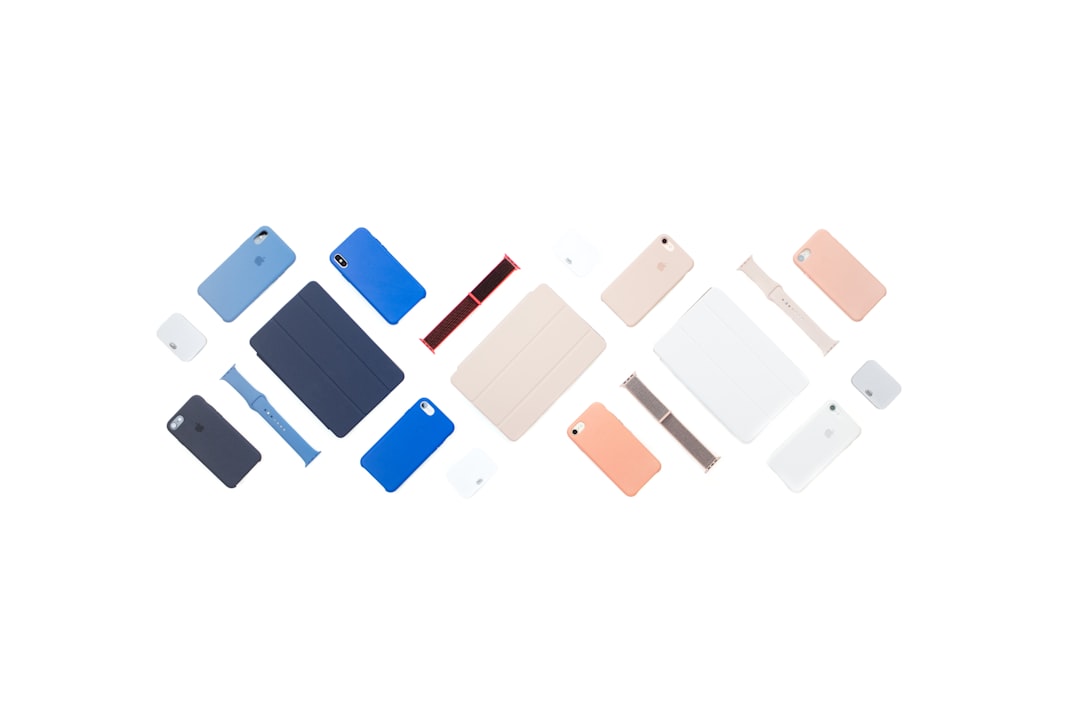
Generative AI is enabling a new level of dynamism in e-commerce product visuals through the creation of interactive image sequences. These sequences can be tailored to individual shoppers, dynamically adapting to their actions on a website. This means product displays can be customized on the fly, changing backgrounds, showcasing different angles, and creating a more immersive shopping experience that caters to individual tastes. By offering a wider range of presentation options for the same product, brands can paint a richer visual story. However, this level of personalization carries a potential drawback: the risk that it becomes overly engineered and focuses on surface-level customization at the expense of genuine product discovery and exploration. It's important for designers to consider how to integrate these advanced tools without losing sight of the fundamental goal of helping customers truly connect with products and make informed purchase decisions. The challenge lies in finding that sweet spot where technology enhances the experience without overwhelming it.
AI is starting to create product image sequences that change based on how people interact with them. This means we can dynamically test different visual presentations of products, like lighting and angles, in real time using user data. It's surprising how small changes can significantly impact how someone perceives a product.
It's interesting that AI-generated images are often indistinguishable from professional photos. This reduces the need for traditional photography studios and potentially saves a lot of money on setups and related logistics for e-commerce businesses.
These AI models can make images that appear in different environments, like seasonal settings or themed backgrounds. This gives retailers a chance to showcase products in various contexts without needing to shoot a bunch of new photos, hopefully making them more relatable to customers.
AI image generation can also help us understand and predict trends. By analyzing how users interact with images, the AI can adjust the visuals and potentially create dynamic marketing strategies that change with consumer preferences, rather than being stuck with static product presentations.
AI is making products look incredibly realistic. We get hyper-realistic textures and materials, which should lead to fewer returns because customers are less likely to be disappointed when a product doesn't look as it did online.
AI is pushing us towards automated and always-on optimization for online stores. The visual styles of product photos can be adjusted in real-time using data on how shoppers interact. This makes for a much more flexible marketing strategy that can adapt quickly to changing trends and customer preferences.
The use of quantized models in product image creation is improving quality and increasing the speed of generating images. This agility helps retailers quickly react to changes in the market and consumer feedback, which is a definite improvement over traditional methods.
AI systems are always learning and refining their output based on user responses to different image styles. This is potentially leading to much more targeted marketing approaches where businesses can figure out what styles and presentations work best for different groups of people.
I find it fascinating that we're able to build "virtual fitting rooms" using AI-generated images. Customers can see how items might look in their environment, which could lessen the uncertainty that comes with buying products online. It's a step towards making online shopping a more personal experience.
AI-generated images can also be automatically optimized for mobile devices. This means that resolutions and aspect ratios are just right for smaller screens. Considering that many people now shop online on their phones, this feature helps to make the shopping experience consistent across all platforms. This might help increase user satisfaction overall.
AI-Powered Product Image Generation Leveraging Quantized LLMs for Efficient E-commerce Visuals - AI-Powered Product Staging Revolutionizes Visual Merchandising Online
AI is poised to redefine how products are presented online, revolutionizing visual merchandising in e-commerce. With the power of generative AI, businesses can now create dynamic and personalized product presentations. Retailers can customize product displays in real time, adjusting backgrounds, angles, and even lighting based on customer interactions. This dynamic approach extends beyond static visuals, offering a seamless and consistent brand experience that aligns with the user's journey, whether on a website or across social media. The goal is to create more immersive shopping environments that foster a deeper connection between customers and products.
While this ability to create highly customized visuals is a significant advancement, there's a potential downside. There's a risk that the focus on personalization might become excessive, sacrificing opportunities for product discovery and genuine exploration. It's important for companies to approach this technological wave thoughtfully. AI can be a powerful tool for enhancing the shopping experience, but it shouldn't overshadow the core objective of providing customers with clear and insightful information about the products themselves. As the field advances, we need to consider how traditional visual merchandising strategies might change in response to the increasing use of data and AI within the online shopping environment.
AI is revolutionizing how online stores present products, particularly through automated product staging and image generation. This shift can drastically reduce the time it takes to create compelling visuals. We're potentially looking at new product launches going from concept to market in a matter of days rather than the weeks it used to take, a considerable speedup.
One fascinating aspect is the potential for AI to empower user-generated imagery. Imagine customers being able to visualize products within their own settings – it's a compelling way to increase engagement and satisfaction. By giving customers more control over how they see a product, it makes the marketing experience more interactive and perhaps more personal.
AI-powered image generation can deliver incredibly lifelike graphics, accurately replicating real-world lighting and textures. Studies suggest that this heightened realism leads to a better alignment of expectations, ultimately reducing the number of returns from customers who find that the product doesn't quite match what they saw online.
The ability to automatically adjust backgrounds opens doors to dynamic and flexible marketing. AI could easily shift backdrops based on seasonal trends, like switching to a holiday theme, allowing companies to respond much faster to marketing opportunities. It's intriguing how adaptable this technology can be.
There's growing evidence that AI-generated visuals contribute to a more favorable perception of a brand. The consistent quality and compelling visuals can influence consumers to view brands as more modern and reliable. It's a subtle yet impactful change in perception.
A/B testing becomes much easier when AI can swiftly produce numerous product image variations. We can now explore which visual elements are most appealing to customers without needing to conduct traditional photoshoots and wait weeks for results. This accelerated feedback loop is beneficial for marketers who want to optimize visuals quickly.
The integration of AI into visual merchandising creates a more intelligent and personalized experience. AI can analyze customer behavior and suggest related items within product images, making the whole shopping process more streamlined and intuitive. It's interesting to see how AI can encourage complementary purchases.
AI allows for creative storytelling through dynamic image sequences. For example, a sequence could showcase a product's functionality or ease of use in a way that's more engaging than a single static image. It's a shift toward visual narratives that potentially communicate product benefits more clearly.
Research suggests that AI-enhanced product visuals build trust among online shoppers. This heightened realism makes customers feel more comfortable buying without seeing or touching the product in person. It's a necessary step for improving the overall experience of online retail.
Interestingly, AI can act as a form of market research by identifying visual trends in product images and analyzing consumer behavior. This provides valuable external feedback that not only helps improve image creation but can also guide companies toward evolving consumer preferences. It's a powerful way to adapt to market trends. While this sounds incredibly useful, it also brings up questions about the future of human creativity and design in marketing, and raises important issues regarding privacy and data usage in a world of constantly evolving AI.
AI-Powered Product Image Generation Leveraging Quantized LLMs for Efficient E-commerce Visuals - Efficient E-commerce Visuals Through Machine Learning Optimization Techniques
Machine learning is reshaping the way e-commerce platforms create and present product images. AI algorithms can automate the process of image creation, leading to improvements in speed and efficiency. Furthermore, these techniques can be used to refine product visuals through automated adjustments of color, contrast, and other elements, leading to higher-quality, more visually appealing images. The ability to personalize the presentation of products in real-time, by adjusting elements like background or lighting, creates a more dynamic shopping experience. This approach can adapt to evolving consumer preferences and market trends, potentially boosting engagement and sales. But there's a risk. Overly-personalized experiences could reduce genuine product exploration, leading to a more superficial interaction with products. Striking a balance between tailored visuals and clear product representation is key to ensuring AI doesn't detract from the core purpose of e-commerce: providing customers with a strong understanding of the products they are considering. As this field continues to develop, it's crucial to remember that the goal of these advanced techniques is to enhance, not replace, the fundamental values of a brand and the trust customers place in it when they shop online.
1. The capacity for real-time adjustments in product presentation is fascinating. AI can dynamically change the scene around a product—switching backgrounds, adjusting lighting—all in response to how a customer interacts with the page. It's a way to make the experience more personalized and responsive, which could be key to keeping shoppers engaged.
2. The sheer number of product variations that AI can churn out for testing is remarkable. It's like having a never-ending supply of photo variations for A/B testing. Instead of waiting weeks for photoshoots, we can very quickly determine which visuals work best, potentially increasing the effectiveness of marketing campaigns.
3. One of the more compelling aspects of AI-generated images is how convincingly realistic they are. They create visuals that are often indistinguishable from professional photos, particularly in terms of texture and lighting. This higher degree of realism has the potential to cut down on return rates since customer expectations are more closely aligned with the product they receive.
4. The capability to instantly switch out backgrounds in product images based on trends or campaigns is quite ingenious. It's like having a near-limitless array of virtual studio sets. Retailers can instantly transition from a summer theme to a holiday theme without extra photo shoots, providing great flexibility to adapt to marketing needs as they come up.
5. It's an interesting development that we can empower customers with some visual control through AI. Instead of just seeing a staged product in a studio setting, they can see how it might look in their own home or environment. It's a more interactive approach to online shopping, which could improve the shopping experience and boost satisfaction, but it's still early to say how much of an impact this has on sales.
6. AI offers the potential for a high degree of consistency in how product images look, something that can be hard to achieve with manual photography. Each image can be tweaked to maintain a consistent brand look and feel. The automation fosters a sense of consistency and professionalism which builds trust with customers.
7. AI-powered image generation significantly speeds up the time it takes to get new products online. In a fast-changing market, this is crucial. Companies can quickly respond to new trends or consumer feedback by getting product visuals out faster. Potentially, a product idea could go from concept to online store in days, a huge improvement.
8. It's clever how AI can analyze customer interactions to suggest related products alongside the main one. It's a step towards more intelligent upselling within the visual space. However, we need to ensure that these product recommendations aren't too intrusive or pushy. If done well, it makes the browsing experience more fluid and caters to related shopping needs.
9. AI offers a window into what visual trends are developing in online shopping. By analyzing customer interactions, it can predict shifts in how customers respond to certain aesthetics or styles. This can be a powerful tool for adapting marketing efforts to stay ahead of the curve, but we need to be careful about how this data is used.
10. The elevated quality of AI-generated visuals not only improves the look of individual products but can also significantly impact how a brand is perceived. Higher-quality images can make a company appear more modern and trustworthy. It's a subtle effect, but it's definitely worth considering how image quality is tied to customer perceptions and ultimately, conversions. We still need more long-term data to see the full impact of this trend.
Create photorealistic images of your products in any environment without expensive photo shoots! (Get started for free)
More Posts from lionvaplus.com: