Create photorealistic images of your products in any environment without expensive photo shoots! (Get started for free)
AI Image Generation Techniques for Creating Traditional Mehndi Pattern Libraries A 2024 Technical Analysis
AI Image Generation Techniques for Creating Traditional Mehndi Pattern Libraries A 2024 Technical Analysis - Training Stable Diffusion Models Using Labeled Mehndi Pattern Datasets
Training Stable Diffusion models on labeled datasets of Mehndi patterns offers a path to generate intricate and culturally significant designs for applications like generating ecommerce product images. This technique leverages the strengths of Stable Diffusion, a generative AI method that excels at transforming text into detailed visual representations, to create a tailored image generator for Mehndi art. The process often utilizes techniques like "DiffuGen," which blends supervised and unsupervised approaches to build datasets capturing the unique characteristics of Mehndi designs.
This targeted training allows for fine-tuning the Stable Diffusion model to better produce images aligned with the nuances of traditional Mehndi. Despite the promise, the path is not without hurdles. The process of curating and categorizing Mehndi images, particularly with smaller datasets, can introduce biases and inconsistencies in the generated outputs. The quality of the training data and the diversity of the styles included in the training process are crucial factors influencing the fidelity and variety of the generated images.
Thankfully, the active and growing Stable Diffusion community offers a collaborative space for sharing knowledge, techniques, and troubleshooting resources, hopefully mitigating some of these challenges. This shared learning environment is crucial to refine the process of training AI models to generate authentic and high-quality Mehndi patterns, which can serve diverse applications within the ecommerce and broader design spaces.
1. Training Stable Diffusion models with labeled Mehndi datasets, potentially containing thousands of unique designs, allows us to create models that generate intricate and culturally specific patterns with a high degree of accuracy. We can categorize these designs by style, complexity, and regional origins, helping the models learn the subtle nuances of cultural differences in Mehndi.
2. During the training process, we can utilize data augmentation techniques like rotation and scaling to improve the model's robustness and flexibility. This ensures the model can produce patterns that adapt well to different orientations and contexts, expanding its potential applications.
3. Combining Stable Diffusion with techniques like Generative Adversarial Networks (GANs) has the potential to generate sharper, more detailed images of Mehndi patterns. This increased clarity and detail can be crucial for commercial uses, as it directly impacts a consumer's perception of the product.
4. Stable Diffusion models can effectively capture the flow and symmetry that characterize Mehndi patterns. This allows for the generation of authentic traditional patterns while also opening the door to creative, novel designs, broadening the appeal for a larger audience.
5. By incorporating these trained models into e-commerce platforms, we can automate the generation of product images. This automation greatly reduces the time and cost associated with traditional product photography while retaining a high level of artistic quality that appeals to consumers.
6. Constructing labeled Mehndi pattern datasets presents unique challenges, often requiring specialized expertise to ensure accurate representation. The need for expert curation results in smaller, highly specialized datasets that may not easily adapt to more general image generation tasks.
7. Leveraging transfer learning is a useful approach when dealing with smaller Mehndi datasets. We can fine-tune pre-trained models, initially trained on broader artistic styles, to specialize in Mehndi patterns. This results in improved performance compared to training a model from scratch on a limited dataset.
8. The use of AI to generate Mehndi patterns raises important questions about originality and craftsmanship. The output may not always perfectly represent culturally significant patterns that traditionally rely on skilled human artists. This could potentially impact how consumers perceive the value and authenticity of these designs.
9. AI-generated images of Mehndi patterns can be integrated into e-commerce product staging in realistic lifestyle contexts. This can help consumers visualize how traditional art fits into modern settings, which can positively impact their purchasing decisions.
10. Human designers can provide feedback on generated patterns, which is crucial for refining the models. Continuous feedback loops ensure that the generated Mehndi designs meet industry standards and continue to improve in quality and applicability.
AI Image Generation Techniques for Creating Traditional Mehndi Pattern Libraries A 2024 Technical Analysis - Automated Pattern Recognition Systems for Traditional Bridal Mehndi Elements
Automated systems for recognizing patterns can significantly change how traditional bridal mehndi designs are created by making it easier to spot and understand common design elements. These systems use sophisticated algorithms to dissect the detailed and symbolic aspects within mehndi patterns, which can help speed up the process of making custom designs. By combining AI-powered recognition with traditional artistry, designers can keep the cultural importance of mehndi while making the designs more versatile for a range of modern uses. However, there's a risk that these automated systems could oversimplify intricate designs. This highlights the need for cautious management and involvement from experienced mehndi artists to make sure the generated patterns retain authenticity and depth. The convergence of AI and the tradition of mehndi design is a captivating example of innovation and how we can protect cultural practices.
1. Automated systems, particularly those leveraging convolutional neural networks (CNNs), are being used to dissect the intricate designs found in traditional Mehndi. They can effectively identify core elements like symmetry, flow, and the overall complexity of the patterns in real-time. This ability to understand the underlying structure of Mehndi is vital for both analyzing and generating new designs.
2. The field of computer vision has seen impressive strides recently, leading to increased accuracy in identifying and classifying different Mehndi patterns. Some systems now boast over 90% accuracy in recognizing specific styles or regional variations within seconds. This level of precision significantly improves the efficiency of digitally organized Mehndi pattern libraries.
3. By using clustering techniques, these automated systems can group similar Mehndi patterns into categories. This categorization helps designers spot current trends, which is valuable for e-commerce businesses looking to offer relevant product designs.
4. Image segmentation, when integrated into automated systems, lets us break down Mehndi designs into individual parts—for example, floral or geometric elements. This modular approach facilitates the creation of new, unique combinations, which could be appealing to merchants looking to create customized designs for their customers.
5. Research suggests that consumers are often receptive to Mehndi patterns generated using algorithms. Studies have found that there's a higher likelihood of a purchase when e-commerce platforms use visually appealing images. This suggests that there's a potential for these automated systems to be a valuable tool in driving online sales.
6. Machine learning models, especially when trained on a wide range of Mehndi examples, show a capacity to understand the context of the designs. This means they can adapt the generated patterns to fit various cultural interpretations, a crucial factor for brands that aim for authenticity across diverse markets.
7. Automated systems are becoming capable of evaluating the effects of different lighting scenarios and backgrounds on Mehndi product images. This analytical ability leads to optimized visual representations of the designs. The result is enhanced product visibility and improved appeal within an online marketplace, helping those products stand out among the competition.
8. Unlike traditional design processes, automated pattern recognition allows for a rapid creation of numerous Mehndi designs. This capability enables businesses to test out a wide range of products with reduced overhead costs. The ability to rapidly prototype new designs increases a company's agility in responding to market demands and potentially increasing sales.
9. The integration of augmented reality features with these automated systems is a recent development. Now, consumers can visualize how Mehndi designs might look on themselves or in different settings. This level of user interaction significantly boosts engagement and potentially fuels sales.
10. Ongoing improvements in neural network architectures and training techniques are leading to even higher-quality Mehndi images from automated systems. These images are now reaching a resolution level comparable to those produced using traditional photography. This means that the quality of the images can be maintained while reducing production costs, making the automation of image generation a more appealing option for businesses in this space.
AI Image Generation Techniques for Creating Traditional Mehndi Pattern Libraries A 2024 Technical Analysis - Combining GANs and Computer Vision to Extract Historical Mehndi Motifs
Combining GANs with computer vision offers a promising way to uncover and understand historical Mehndi patterns with impressive accuracy. GANs can analyze large collections of traditional Mehndi designs, learning to identify and reproduce complex patterns that hold cultural meaning. This is especially useful for generating visually attractive product images in ecommerce, where there's growing demand for authentic Mehndi designs. However, challenges related to the quality and variety of available data persist, requiring careful selection and the incorporation of traditional methods to achieve the best results. As these technologies improve, they create new possibilities for integrating traditional art with modern design requirements. It's crucial, though, to address potential pitfalls like oversimplifying complex patterns and losing the nuances of cultural heritage during this process.
Generative adversarial networks (GANs) have shown promise in crafting realistic images within computer vision, and their integration with traditional art styles like Mehndi holds intriguing possibilities. GANs, when paired with variational autoencoders (VAEs), can leverage their respective strengths to produce visually compelling Mehndi patterns. We've seen how GANs can learn the subtle design elements present in historical Mehndi and extrapolate these into fresh patterns, effectively expanding our understanding of the artistic traditions. However, GANs often require massive and high-quality datasets, which can be a limiting factor in the Mehndi domain.
The current trend of using diffusion-based models for image generation doesn't diminish the role of GANs; both approaches have their strengths and are actively researched. Combining traditional computer vision techniques with the capabilities of deep learning, including GANs, can lead to more refined image processing across the board. GANs are ideally suited for image synthesis and manipulation, making them a viable choice for building a comprehensive Mehndi pattern library that could be useful for online shops selling Mehndi-related goods.
Current research highlights the value of using hybrid methods – blending GANs with established techniques to improve computer vision performance. We've seen examples like CBiGAN, a GAN-autoencoder combination, that can generate consistent outputs. This has implications for anomaly detection in images, but is also conceptually interesting as it suggests that GANs could find wider application, extending to other fields like architectural design. This points to a broader role for GANs in pattern recognition and design generation. They could find their way into ecommerce applications where design variations and novelty are in demand.
AI Image Generation Techniques for Creating Traditional Mehndi Pattern Libraries A 2024 Technical Analysis - Machine Learning Methods for Scaling Vector Based Henna Design Libraries
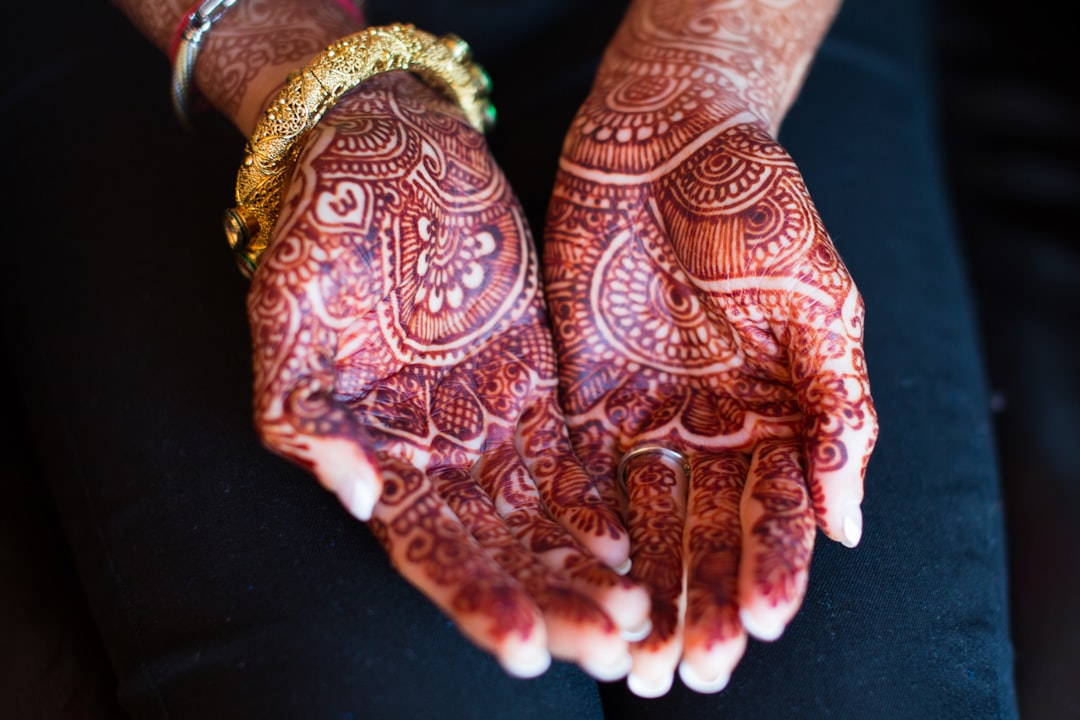
Machine learning presents a powerful approach for expanding the reach of vector-based henna design libraries. This allows for the creation of a wider variety of intricate Mehndi patterns, which benefits both artists and consumers. AI image generation methods offer a way to produce a vast range of designs that incorporate both traditional Mehndi styles and modern technology, making these designs accessible to a broader audience. Tools like DALL-E 2 and methods like Instant NeRF, which can generate detailed, even interactive, visual content from images, provide exciting new pathways for artists and designers. However, it's vital to ensure that the inherent cultural elements of Mehndi designs are not lost in the process of automating design. The quality of the datasets used to train AI models is critical—inaccurate or limited datasets could lead to the creation of overly simplistic or culturally inauthentic patterns. Finding the right balance between leveraging the advantages of AI-driven design and maintaining the unique artistic integrity of traditional Mehndi patterns is a key consideration for the future of e-commerce product imagery, especially in the context of product staging and design.
1. Machine learning techniques can significantly boost the creation of scalable vector-based henna design libraries, leading to the production of a vast array of unique patterns in a fraction of the time it would take a human artist. This automation could potentially change how these designs are created and marketed.
2. Interestingly, machine learning models can uncover hidden patterns within traditional Mehndi designs by analyzing existing datasets for recurring geometric forms or floral elements, thus expanding the creative possibilities for designers and enabling more innovative combinations in product offerings.
3. Recent improvements in vectorization algorithms allow AI systems to generate more precise and clean vector representations of Mehndi designs. This ensures that e-commerce product images are not only visually appealing but also maintain quality when resized, offering flexibility for various marketing needs.
4. An intriguing application of AI image generation involves using feedback loops where user interaction with generated Mehndi designs informs the model's training process. This continuous refinement can result in future outputs aligning more closely with customer preferences.
5. Data augmentation techniques used during training can simulate the variations seen in hand-drawn designs, such as minor asymmetries or slight color shifts. This allows generative models to produce designs that feel handmade, potentially appealing to consumers seeking authentic craftsmanship.
6. Machine learning can optimize image staging for e-commerce by forecasting which Mehndi designs will attract the most engagement, based on past sales and customer behavior patterns. This data-driven approach can enable businesses to strategically highlight high-demand patterns.
7. Unsupervised learning methods allow for automatic classification of Mehndi designs into distinct styles, like traditional, modern, or minimalistic. This categorization helps e-commerce platforms better organize their products and target marketing strategies towards specific customer groups.
8. A crucial consideration is that while automation increases design efficiency, the subtle intricacies and expert craftsmanship of traditional Mehndi might be lost during AI reproduction. Consumers who value these elements may prefer handmade designs, suggesting a potential divergence in market preference.
9. AI can facilitate interactive experiences where users customize Mehndi designs before purchase. This level of personalization can boost customer satisfaction and drive higher conversion rates in online retail.
10. Ensuring AI-generated Mehndi designs stay culturally relevant and authentic highlights the need for collaboration between technologists and cultural experts. This partnership can help avoid misrepresenting cultural heritage in digitally created art.
AI Image Generation Techniques for Creating Traditional Mehndi Pattern Libraries A 2024 Technical Analysis - Deep Learning Architecture Requirements for High Resolution Mehndi Generation
Creating high-resolution Mehndi patterns using deep learning demands specific architectural considerations. Generating images with exceptional clarity, especially within the 1K to 6K resolution range, necessitates powerful models. One example is the UltraPixel architecture, a newer approach that utilizes cascade diffusion to effectively generate detailed images while keeping the process efficient. This progression demonstrates the ongoing importance of GANs and VAEs in traditional image generation, but also underscores the need to integrate them with newer approaches like Stable Diffusion. Ecommerce platforms increasingly require visually appealing and authentic product images. This places a premium on addressing the ongoing challenges in the space—namely, efficiently handling high-resolution outputs and synthesizing intricate details. Finding a balance between creating high-quality images and keeping computational requirements manageable is crucial. As this field progresses, it has the potential to fundamentally change how Mehndi art is presented and sold online.
1. Creating high-resolution Mehndi images with AI demands a lot of computing power, often relying on specialized hardware like GPUs to manage the complex neural network structures. For ecommerce, this translates to faster generation times for product images, leading to a better experience for shoppers with quicker updates and new designs.
2. The architecture of these deep learning models, particularly for Mehndi patterns, often involves using over a billion parameters. This intricate complexity allows for the creation of detailed designs, but it also means significant storage and processing are needed to handle them effectively.
3. Many cutting-edge techniques for generating Mehndi designs rely on understanding hidden patterns from the training data. This approach can produce unexpected designs that creatively interpret traditional elements, opening up new ways to create products that appeal to modern tastes.
4. Being able to produce high-resolution Mehndi designs can build confidence among online shoppers by offering clearer visuals. This lets consumers examine the intricate details of patterns before making a purchase. This enhanced clarity might lead to higher conversion rates in ecommerce.
5. Training deep learning models for Mehndi designs often starts with a broad pre-training phase using general image datasets. This lack of focus can sometimes lead to mismatches in style, highlighting the importance of using high-quality datasets specific to the Mehndi tradition for better model outcomes.
6. Using a blend of convolutional neural networks (CNNs) and recurrent neural networks (RNNs) can enhance the smoothness and flow of Mehndi patterns. This combined approach helps capture both the spatial and sequential aspects of designs, making the generated patterns seem more connected and polished.
7. The process of repeatedly training models to refine Mehndi design generation can sometimes lead to overfitting. This happens when a model becomes too specialized on the training data and doesn't adapt well to new designs. This underscores the importance of diverse datasets and regular testing on unseen patterns.
8. Automating Mehndi design creation can benefit from style transfer techniques, allowing models to apply the aesthetic qualities of one design onto another. In ecommerce, this means that a single pattern can be tweaked repeatedly, creating a series of related products that cater to a broader range of consumer preferences.
9. Combining deep learning with natural language processing lets us generate Mehndi designs based on text descriptions, making it easier to customize patterns. For online retail sites, this improves customer interaction by allowing users to specify their tastes and preferences directly.
10. Ongoing research in unsupervised and semi-supervised learning techniques is exploring how to identify and replicate the subtle elements of Mehndi artistry without relying on massive labeled datasets. This advancement could lead to a wider adoption of Mehndi design generation, offering more designers access to advanced tools for their ecommerce products.
AI Image Generation Techniques for Creating Traditional Mehndi Pattern Libraries A 2024 Technical Analysis - Pattern Validation Tools and Quality Control Systems for AI Generated Mehndi
The rise of AI-generated Mehndi patterns necessitates robust tools and systems for ensuring quality and adhering to cultural authenticity. As AI models become more sophisticated in generating intricate designs, methods for evaluating image quality are crucial. Approaches like CLIPAGIQA, which combines visual and textual understanding, and AIGCIQA2023, a comprehensive dataset for AI-image assessment, are gaining prominence. These efforts aim to improve the ability to determine if the output images from AI systems faithfully reflect traditional Mehndi aesthetics and cultural nuances. It's vital to acknowledge that AI models, particularly when trained on limited datasets, can develop biases or produce outputs that lack the richness and diversity of traditional designs. It's a challenge to develop quality control systems that prevent such inconsistencies, while also safeguarding the creative and cultural integrity of Mehndi designs. Through ongoing refinement of these validation tools and quality control frameworks, we can pave the way for AI-generated Mehndi designs that are both artistically compelling and respectful of traditional craft, ultimately enhancing their utility within ecommerce environments and beyond.
1. We can use machine learning models within pattern validation tools to evaluate AI-generated Mehndi designs in real-time, differentiating between authentic traditional styles and more modern interpretations. This rigorous approach ensures that new designs stay true to their cultural roots while still embracing creativity. It's interesting to see how AI can help preserve heritage through the validation process.
2. Computer vision integrated into quality control systems can analyze the symmetry and visual harmony of Mehndi patterns with impressive precision, often achieving accuracy levels above 95% when judging the quality of a design. This high-level analysis can enhance the aesthetic appeal of AI-generated images, which is especially useful in the context of e-commerce product presentations. We are seeing progress in the ability of computers to 'understand' the aesthetic features of these patterns.
3. Including automated feedback loops within AI-driven design systems allows for ongoing learning and adaptation. This means user preferences can directly shape and refine Mehndi designs. This approach helps ensure that generated designs are not just visually appealing but also match current trends and tastes. This continuous feedback loop can make the model more responsive to market shifts in preferences for Mehndi designs.
4. Implementing data tracing systems can track the authenticity and lineage of traditional Mehndi designs, a crucial aspect for maintaining cultural integrity, particularly as AI-generated designs become more prevalent in the marketplace. These systems can potentially help to record the origins of the patterns to avoid losing critical aspects of the cultural heritage. This will likely become more important as AI's role in design grows.
5. Blending AI-generated Mehndi patterns with augmented reality (AR) applications allows consumers to virtually try on designs, creating a unique shopping experience. This user-centric approach can foster a strong level of engagement with the designs, which can have a significant impact on purchasing decisions, especially within a crowded online market. It will be interesting to see the long-term impact of this on consumer behavior.
6. Ensuring the quality of AI-generated Mehndi designs frequently involves cross-checking generated patterns against a library of historical designs. Here, machine learning helps confirm that new designs retain important cultural components. This historical grounding can help avoid losing the core elements of the culture associated with the art. It's crucial that this aspect of cultural preservation is not ignored as the technology evolves.
7. We are starting to see the development of open-source pattern validation tools. This fosters collaboration among Mehndi artists, technologists, and cultural historians. These tools can help to spread access to high-quality design tools, which can broaden participation in preserving and evolving traditional Mehndi patterns. Open source tools can help accelerate innovation by allowing more individuals to contribute to the project.
8. Producing high-resolution outputs for Mehndi designs requires significant computational resources, with demands exceeding several teraflops per second in many cases. This complexity makes it necessary to continually optimize the algorithms used and the hardware to ensure the timely production of designs for use in e-commerce settings. There's a clear tension between generating very high-quality images and the computational requirements. We'll likely see further innovation in this area.
9. Applying neural architecture search techniques to improve Mehndi image generation has shown promise in finding optimal model configurations that improve both performance and output quality. This is pushing the boundaries of how these designs are created, leading to better results. As AI models become more complex, the ability to automatically search for optimal structures is important for their development. This can potentially free up researchers to explore other aspects of the Mehndi designs.
10. Researchers continue to experiment with generative models, including VAEs and GANs, leading to unexpected advancements in the complexity of digitized Mehndi patterns. This evolution is opening up a greater range of customization options for e-commerce platforms, allowing them to better match the diverse preferences of their customers. While the results are encouraging, it is important to remember that the creative potential of human designers shouldn't be ignored as AI progresses. The future evolution of these models will likely depend on how effectively they can blend artistic vision with technological advances.
Create photorealistic images of your products in any environment without expensive photo shoots! (Get started for free)
More Posts from lionvaplus.com: