AI Image Generation Challenges Creating Photorealistic Chicken Textures in Product Photography
AI Image Generation Challenges Creating Photorealistic Chicken Textures in Product Photography - Neural Networks Struggle With Feather Detail Rendering in AI Generated Poultry Photos
AI-powered image generation, while rapidly evolving, still faces a significant challenge: accurately rendering the fine details of feathers in poultry images. This difficulty is particularly evident when using neural networks like GANs. The struggle to realistically portray these complex textures leads to images that fall short of genuine photorealism, a crucial aspect for ecommerce product photography.
Even with advancements in deep learning architectures, like Vision Transformers and diffusion models, generating convincingly detailed organic structures like feathers remains elusive. This ongoing challenge speaks to a broader issue in achieving true-to-life quality in AI-generated imagery, specifically within the context of product visualization. It's clear that creating photorealistic textures across different products and perspectives is a complex task, emphasizing the ongoing need for refinements in the field of AI image generation within the ecommerce landscape.
1. Current neural networks, despite advancements, face difficulties in capturing the intricate details of textures like feathers, likely due to limitations in their resolution and understanding of complex real-world surface variations.
2. Creating realistic-looking poultry feathers requires a vast amount of high-quality training data showcasing the subtle color shifts and density variations that make them unique. Without sufficient data, the AI models may produce generic, less-than-authentic representations.
3. Product photography relies on accurately representing how light interacts with surfaces. Neural networks, however, often fail to properly mimic how feathers scatter light, contributing to the unnatural appearance of AI-generated poultry images.
4. Sometimes, AI-generated imagery simplifies textures too much, producing a somewhat artificial, "plastic" look instead of the naturally organic look of real feathers. This can be a problem for e-commerce, as it could potentially affect how customers perceive the product.
5. Methods like adversarial training are widely used in image generation, but even these sophisticated techniques haven't completely solved the problem of accurately capturing the subtle textures of feathers. This suggests limitations in their ability to accurately render specific biological features.
6. Generating high-fidelity feather details often needs much higher resolution than what typical product images use. This higher resolution output isn't yet standard for many AI models, which is a hurdle for creating realistic images.
7. When training data is of poor quality, it introduces errors that can amplify when trying to generate fine details like feather patterns. If the training examples aren't clear, the resulting images might have chaotic or distorted textures instead of the intended look.
8. The wide variety of chicken breeds and their diverse feather textures add another layer of complexity for AI. Models trained on images of one breed may struggle to accurately represent the unique feathers of a different breed.
9. While techniques like Progressive Growing GANs have shown improvements in generating textures, they still encounter difficulties in producing consistently realistic feathers and other intricate details.
10. The variability in the quality of AI-generated poultry images presents a potential problem for online sellers. Misleading images can impact trust and possibly affect customer purchase decisions, especially if the images fail to accurately represent the product.
AI Image Generation Challenges Creating Photorealistic Chicken Textures in Product Photography - AI Product Staging Fails to Match Professional Food Photography Light Setup
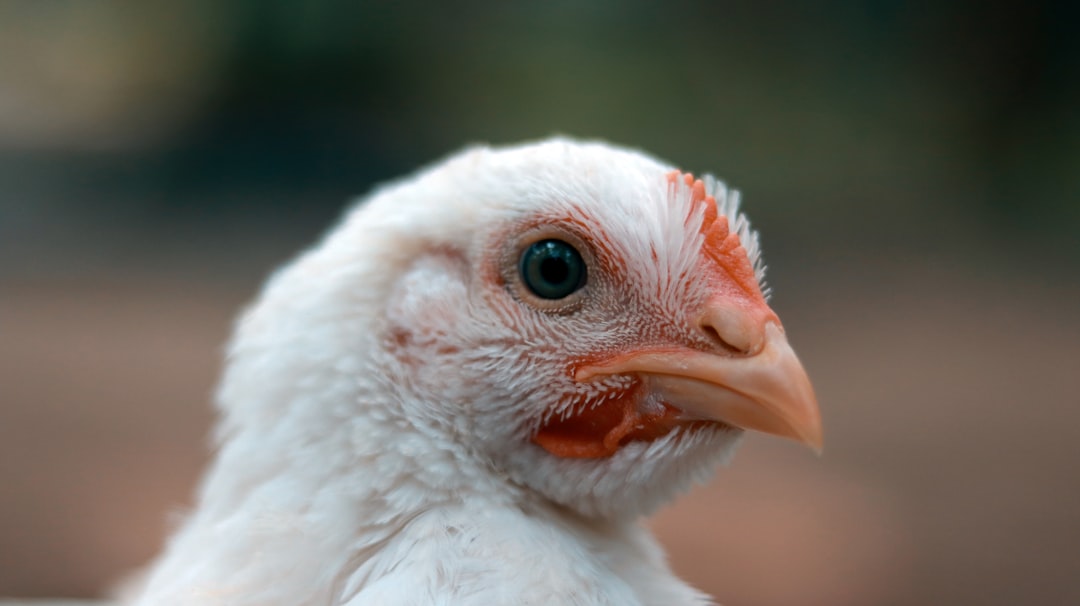
AI image generation tools are making strides, but they're still not quite able to recreate the expert lighting techniques used in professional food photography. This is crucial for ecommerce product imagery because proper lighting brings a product to life, and currently AI generated images often fall short. This is particularly evident when you're trying to showcase intricate textures like those found on poultry, where light plays a key role in making the product look real. Getting the right light and shadow patterns is important to make the image authentic and appealing. While AI can certainly speed up many parts of the product image creation process, these lighting limitations make it tough for AI to truly capture the realism seen in professional food photos. This is something to think about when online retailers are presenting their goods, as customers expect high-quality product images. As AI continues to advance, we'll hopefully see improvements in how AI tools handle light and texture, closing this gap between AI-generated imagery and high-quality photography.
Current AI systems for generating product images often struggle to mimic the intricacies of professional lighting setups commonly used in fields like food photography. This disconnect stems from a fundamental limitation: a relatively incomplete understanding of light's physics. Many AI models fail to accurately capture the interplay of shadows and highlights, resulting in images that lack the depth and realism achievable with a skilled photographer and well-designed lighting setup.
The nuances of color temperature are another challenge. While experienced photographers carefully choose light sources to influence the appearance of a product, AI often assigns colors without considering how different lighting types impact a product's visual appeal. This can lead to images that don't accurately represent how a product would look in a real-world setting.
Furthermore, professional food photography often relies on high-dynamic-range (HDR) techniques to capture a broader spectrum of light and shadow. However, many AI image generation models struggle to reproduce these nuanced renderings due to their reliance on more standard imaging techniques. The absence of this subtlety can lead to AI-generated images that appear flat and lack visual richness.
This issue of realistic lighting becomes particularly relevant for ecommerce, as the visual presentation greatly influences customer perceptions. Images with poorly rendered lighting might appear less appealing and cause consumers to question the quality of the products being advertised. If an image looks excessively bright or lacks depth, it can erode the trust necessary to drive sales.
Complex light behaviors, like iridescence in feathers, are extremely difficult for AI to reproduce accurately. These intricate visual characteristics are often oversimplified by AI, which often misses the subtle variations that professional photographers can capture with specialized lighting techniques and staging.
The challenges don't stop at lighting itself. Staging products effectively, a core component of impactful photography, is also challenging for AI. The subtle ways light diffuses and is absorbed by surfaces aren't always considered by current AI algorithms. This oversight results in unrealistic product images that can mislead both consumers and the sellers hoping to showcase their goods effectively.
Beyond issues with the depiction of light, many AI models also struggle to accurately replicate the stable shooting conditions of professional setups. As a result, AI-generated images sometimes exhibit motion blur or pixelation. This problem is largely due to the model's inability to simulate the steady conditions – like those provided by tripods – that are standard in professional photography.
Furthermore, vital techniques such as backlighting and rim lighting, used to add dimension and visually appealing highlights to food photography, are often overlooked by AI systems. This tendency towards simplistic rendering leads to images lacking depth and visual interest.
Similarly, AI models sometimes falter when it comes to color accuracy under different lighting conditions. For example, AI might incorrectly predict how pigments behave in specific lighting scenarios. This can lead to images where the color representation isn't true to life, potentially misrepresenting the actual product.
Lastly, effective product staging is more than just lighting; it also involves a skillful understanding of composition and perspective. Many AI-generated images stick to basic product placements and angles, lacking the artistic flair that a seasoned photographer can inject into a photograph. This tendency toward generic perspectives can leave a negative impression on discerning consumers who expect more than simply a straightforward, basic representation of a product.
AI Image Generation Challenges Creating Photorealistic Chicken Textures in Product Photography - Machine Learning Models Face Food Texture Recognition Gaps in Raw Chicken
AI's ability to recognize food textures, while improving, faces hurdles in accurately capturing the complexities of raw chicken. Despite advances in deep learning, models struggle to interpret the subtle texture variations crucial for conveying quality and freshness in ecommerce images. While deep learning models outperform traditional methods in classifying food items, they still fall short of accurately representing the intricacies of chicken skin and flesh. This shortcoming can be a concern for online shoppers, as the visual representation of a product is critical for making informed purchasing decisions. The discrepancy between AI-generated imagery and the true-to-life textures of raw chicken highlights the need for ongoing refinements in machine learning algorithms to improve their ability to faithfully capture the visual qualities of food products in online environments. Achieving genuine photorealism for e-commerce images remains a challenge, emphasizing the importance of continued progress in bridging the gap between AI-generated textures and the nuanced reality of the products they represent.
1. Current machine learning models, while improving, still struggle to truly capture the nuances of food textures, especially when it comes to raw chicken. This is partly because their training often relies on 2D images, leading to a lack of depth perception when trying to render the 3D surfaces of things like chicken skin. The result can be images that feel flat and don't effectively communicate the real-world texture of the product.
2. The challenge of capturing texture accurately goes beyond feathers. Chicken skin, for instance, has a range of surface textures – some smoother, some rougher – and reflects light differently depending on that texture. Teaching AI models to recognize and replicate these subtle variations is difficult, as the datasets they're trained on often lack the necessary level of detail.
3. Chicken isn't a single, uniform entity. There are different cuts, marinades, and cooking methods which all affect the final appearance of the chicken and its texture. This wide array of possible textures creates a big problem for AI: it's tough for AI to learn general principles when the training data only focuses on one specific type of chicken presentation. An AI model trained on only roasted chicken might not do a good job at generating an image of raw chicken.
4. Human photographers frequently use techniques like focus stacking to improve texture detail in their shots. This method involves combining multiple photos taken at slightly different focal points to produce a crisper, more detailed final image. However, AI models haven't widely adopted this technique, which potentially limits their ability to capture the same level of realism.
5. In the realm of food photography, freshness is a critical element that influences buying decisions. AI models often miss the subtle visual cues that communicate freshness. As a result, their generated images can feel static and less appealing compared to photos taken by a skilled photographer who understands how to emphasize freshness through texture and other elements.
6. The issue of realistically capturing skin-on textures in AI-generated images becomes even more important when considering how customers perceive products. Consumers generally prefer chicken that looks fresh and tender, and AI's inability to fully replicate these nuances can create a disconnect between the generated image and customer expectations, possibly impacting sales.
7. Many AI image generation algorithms aren't well-equipped to handle the complexities of lighting in professional food photography. The way light interacts with the uneven surfaces of a chicken creates intricate shadow patterns that are tough to capture authentically. Current AI models don't always accurately reproduce these realistic shadows, leading to images that lack visual depth.
8. The challenge of color accuracy under varying lighting conditions is also an issue. Raw chicken requires careful lighting to avoid making it appear unappetizing. Yet, AI models can struggle to reproduce the accurate color tones necessary, which could lead to inaccurate or unappealing images of the product.
9. E-commerce relies on trust, particularly in the food industry where images play a crucial role in influencing purchase decisions. If the images used by an online seller are inaccurate, this can raise concerns about product quality in the minds of the buyer. The imperfections found in AI-generated images can erode this trust, potentially impacting brand perception and sales.
10. While texture is important, it's not the whole story. A truly successful food image needs more than just accurate texture. It also needs artistic composition, clever staging, and the ability to convey a certain emotion or feeling to the viewer. Currently, AI systems lack the artistic finesse of a skilled photographer, and this limitation may hinder their overall effectiveness in online retail situations.
AI Image Generation Challenges Creating Photorealistic Chicken Textures in Product Photography - Training Data Limitations Impact Quality of AI Generated Meat Products
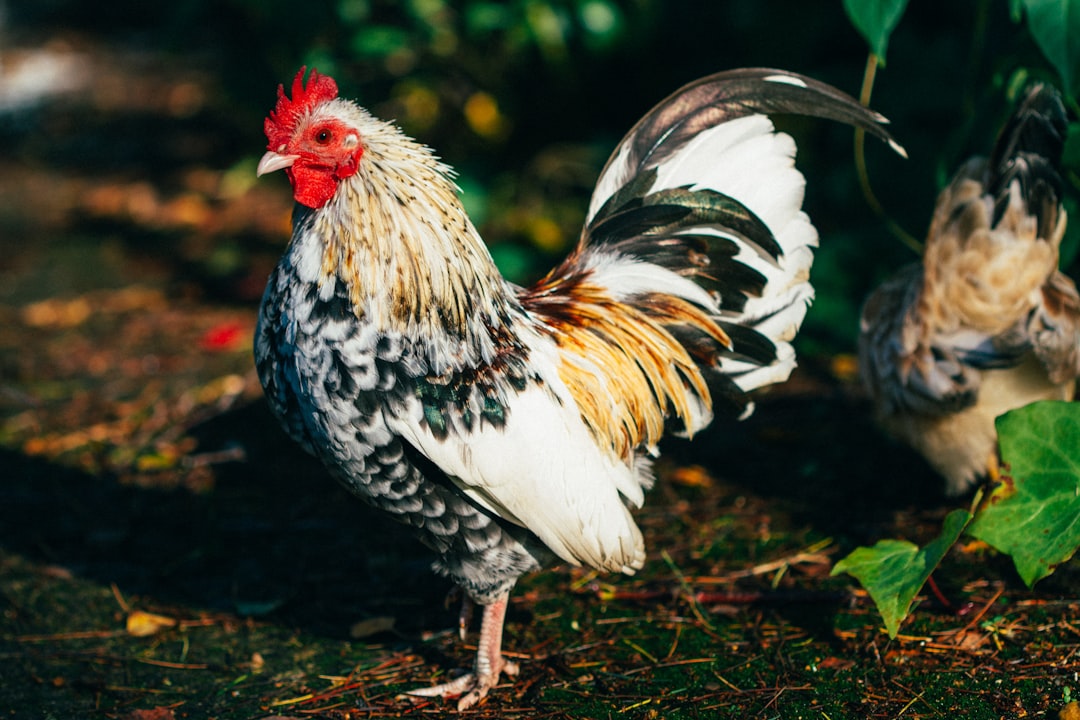
The quality of AI-generated images of meat products, like chicken, is significantly impacted by the limitations of the training data used to create them. If the training data doesn't offer a wide variety of examples and accurate representations, the resulting AI images can have unrealistic flaws, falling short of the photorealistic look crucial for ecommerce. The challenge is further compounded by the growing scarcity of high-quality training data due to restrictions imposed by many major online data sources. With limited and potentially biased data, AI models struggle to generate genuinely innovative and nuanced images, failing to capture the intricate details of textures, such as chicken feathers, and the subtle play of light on the surface of the product. This struggle to replicate the realism of professional food photography can undermine customer trust in online product presentations, potentially affecting sales due to less appealing or inaccurate visual representations. To overcome this, it's vital to find ways to enhance the quality and diversity of training data to improve the ability of AI models to produce realistic and compelling images of meat products in ecommerce environments.
The quality of AI-generated product images, especially those featuring intricate textures like poultry, is heavily dependent on the quality and diversity of the training data used to build the AI models. If the training data lacks a wide range of examples, the AI may develop a limited understanding of the subtle textures needed for photorealistic results.
Many AI image generators rely on standard datasets that don't fully represent the broad spectrum of real-world chicken textures, including common imperfections like variations in skin and subtle inconsistencies that influence how a product appears. As a result, the generated images may not be a truthful representation of what customers could expect when they receive their purchase.
The 3D nature of food textures like chicken skin presents a major hurdle for AI systems that primarily work with 2D images. This limitation often leads to a flattening effect in the generated images, with many details getting oversimplified.
AI models commonly struggle with capturing the full dynamic range of lighting and shadow details. This nuance is crucial for conveying the subtle textural qualities of chicken, and if AI models don't accurately reflect variations in highlights and shadows, the resulting images can lack the realism that's essential for product marketing.
When training datasets lack detailed textures, it can lead to images that miss key features like blood spots or fat marbling that contribute to how consumers judge a product's quality and freshness. This can be problematic for maintaining consumer trust, a vital aspect for successful online sales.
Currently, AI algorithms may oversimplify chicken textures when trained on limited variations of chicken preparation. This simplification results in images that appear overly uniform, failing to capture the visual differences seen in diverse chicken cuts and cooking methods, which are essential elements in driving customer purchase decisions.
Inconsistencies in color representation often arise from limitations in how AI simulates lighting conditions. Factors like the way light scatters through chicken skin significantly impact color perception, which can be misrepresented in AI-generated images if the model doesn't account for this.
The simultaneous need to capture both light interactions and detailed textures makes it difficult for AI to produce a genuine representation of chicken. Experienced human photographers have developed advanced techniques to expertly manipulate lighting angles to create a visually compelling image, a level of finesse that is currently beyond the reach of AI.
As AI models continue to evolve, they should integrate advanced techniques that photographers use such as focused shots and depth of field controls. These methods enhance texture details in a photograph, but aren't standard in most AI training processes, which hinders the realism of AI-generated images.
The diverse preferences of consumers add another layer of difficulty. For example, while some people actively seek organic or free-range chicken, the shortcomings of poorly generated images can fail to effectively communicate these qualities that differentiate high-quality products. This can result in lost sales for online retailers.
AI Image Generation Challenges Creating Photorealistic Chicken Textures in Product Photography - Current Generation Tools Miss Essential Surface Detail in Food Photography
While current AI image generation tools can create visually appealing food photos, they frequently fall short in capturing the crucial surface details needed for authentic product imagery. This is particularly problematic for online retailers, where convincing product shots are essential for building customer trust and encouraging purchases. Despite progress in machine learning, these algorithms haven't fully mastered the ability to accurately recreate the complex textures and subtle lighting that define real food like chicken. This often leads to images that appear overly processed or unrealistic, lacking the depth and realism present in professional food photography. As AI technology progresses, these tools must improve their ability to render intricate details if they want to effectively serve the needs of the ecommerce marketplace. The gap between the realism of AI-generated imagery and what consumers expect from product photography continues to be a challenge, particularly for detailed goods like meat products.
1. Many AI image generation tools are built upon datasets primarily focused on flat, 2D images, which makes it hard for them to capture the intricate 3D variations found on the surfaces of, for example, raw chicken. This is a crucial aspect for making chicken textures look truly realistic.
2. To generate convincingly realistic chicken images, AI needs access to a wide range of training data that showcases various chicken cuts, cooking styles, and textures. Without this variety, AI models can produce generic representations that don't live up to customer expectations.
3. AI models often struggle to incorporate a true sense of depth in their generated images, especially when it comes to foods like chicken. These models need a more nuanced understanding of how light interacts with forms and textures to make chicken look believable.
4. The way AI-generated chicken images represent color can be inaccurate because these AI systems often lack a detailed understanding of how different surfaces interact with light. This leads to pictures that might be too bright or have colors that are off, misleading potential customers.
5. Professional food photography relies on conveying the quality and freshness of food through subtle details like fat distribution, marbling, and other natural imperfections in the meat. AI struggles to pick up on these fine points, which can lead to images that don't accurately represent the actual appeal of the product.
6. Professional photographers use techniques like high-dynamic-range (HDR) imaging to capture the full spectrum of light and color. However, many AI systems haven't yet adopted these techniques, resulting in images that appear flat and less visually interesting.
7. When selling food online, making the product appear fresh is crucial. AI-generated images often miss subtle clues, like sheen and moisture, that are key in conveying freshness and quality. This can lead to a decrease in consumer confidence and potentially fewer sales.
8. When AI systems are trained on limited, unrepresentative data, it can introduce biases into the resulting images. These biases can lead to inaccuracies in the AI-generated product images, potentially impacting how buyers perceive a product's quality.
9. AI often simplifies complex textures into uniform and overly smooth representations. Real chicken has intricate variations in texture, and when AI misses these details, it can lead to a less appealing image and potentially fewer sales.
10. Creating compelling ecommerce product photography involves not just accurate texture and color, but also thoughtful composition and a knack for visual storytelling. Currently, AI doesn't fully grasp these artistic components of photography, limiting its ability to truly engage and appeal to customers.
AI Image Generation Challenges Creating Photorealistic Chicken Textures in Product Photography - Computer Vision Systems Show Limited Understanding of Moisture Levels in Food Products
Current computer vision systems haven't fully grasped how to accurately gauge the moisture content in food items, especially when it comes to images used in ecommerce. This is a problem because correctly showing moisture levels is key to conveying the quality and freshness of things like chicken. Even with improvements in AI, these systems often fail to capture the fine details that suggest a product's quality and freshness. The inability to accurately show moisture can result in images that don't represent the product accurately, which can decrease consumer trust and potentially reduce sales. Since online sales increasingly rely on images that look very real, it's critical that AI models learn to better understand food textures and conditions. We need a better match between the images that AI generates and what people expect to see in the real world. This emphasizes the continued need for AI image generation improvements if they are to effectively represent the details found in food products and increase confidence among online shoppers.
Computer vision systems, while adept at recognizing basic features like color and shape, demonstrate limitations in accurately interpreting and representing the moisture content within food products. This is particularly notable in the context of chicken imagery, where subtle indicators of moisture, like a sheen, can significantly influence a customer's perception of freshness and quality.
The training data used to develop these AI image generation models often lacks the high-resolution images necessary to fully capture the range of moisture variations found in chicken. This deficiency restricts the AI's ability to reproduce the moisture aspect accurately, sometimes leading to images that appear too dry or, conversely, excessively glossy in an unnatural way.
Current neural network architectures tend to oversimplify the intricate relationship between moisture and the surface texture of a product. As a consequence, the subtle nuances, such as the glistening look of freshly washed or rinsed chicken, are often misrepresented or lost entirely. This can erode consumer trust, as it suggests a lack of fidelity to the actual product being presented.
The way light interacts with wet surfaces, causing reflections and refractions, presents another challenge for these systems. The detailed patterns of light created by moisture are frequently lost in AI-generated images, leading to a diminished visual appeal and less realistic depictions.
Human photographers, through years of experience, can instinctively adjust their shots to highlight the most appealing characteristics of food, including capturing moisture levels in a manner that suggests freshness. AI lacks this intuitive understanding of how to showcase freshness, sometimes resulting in images that fail to convey this crucial product quality.
Capturing the interplay between textures and moisture in a way that indicates quality and freshness introduces further complexities for AI algorithms. Representing this intricate relationship is crucial for e-commerce, as it greatly impacts how consumers assess the product's appeal and desirability.
Variations in the lighting used during AI image generation can lead to inconsistent portrayals of moisture levels. This inconsistency can mislead customers, as the generated images may not accurately reflect the actual product's state, potentially leading to lowered expectations or purchase hesitation.
The lack of diverse training data, which incorporates various cooking methods and treatments like marinades, limits the AI's understanding of how moisture presents itself in different scenarios. This ultimately hinders the creation of realistic and relatable imagery.
Research suggests that visual cues related to moisture can trigger positive associations with freshness and quality in consumers. AI-generated images that fail to accurately reflect moisture levels may inadvertently suppress these desirable associations, potentially impacting purchasing decisions.
In e-commerce product photography, particularly in the food sector, visible moisture often serves as a strong indicator of product quality and freshness. The inability of AI to accurately capture and display these nuances can compromise the effectiveness of online marketing efforts and potentially hurt the overall success of online retailers.
More Posts from lionvaplus.com: