Create photorealistic images of your products in any environment without expensive photo shoots! (Get started for free)
AI-Enhanced Product Image Reconciliation Bridging E-commerce and Financial Accuracy
AI-Enhanced Product Image Reconciliation Bridging E-commerce and Financial Accuracy - AI-Powered Visual Recognition Streamlines Product Categorization
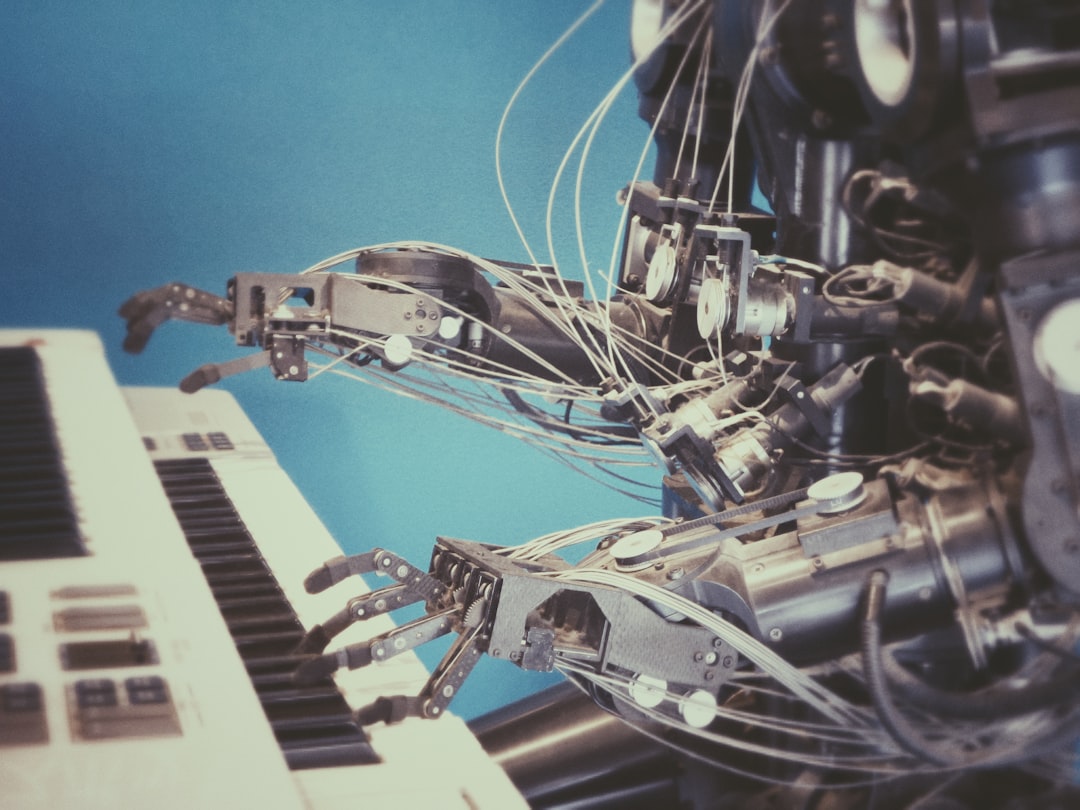
AI-powered visual recognition is changing the landscape of product categorization in e-commerce. It lets shoppers find products by simply uploading an image, making searching for items much easier. The technology's accuracy in identifying products is particularly useful for those who might not know the exact name or description of what they are looking for. Behind the scenes, AI uses machine learning to organize products efficiently, resulting in more user-friendly online stores. Customers can browse and find what they need more quickly, potentially leading to a boost in sales for businesses. The trend of integrating AI-driven visual recognition goes beyond just better organization. These systems, as they become more sophisticated, are creating richer interactions between consumers and online stores, leading to increased engagement and, ultimately, more purchases.
AI's ability to understand product images is transforming how online stores organize their offerings. While previously reliant on human categorization, we're seeing a surge in AI's capacity to analyze product visuals with remarkable accuracy, sometimes exceeding 95% in classification tasks. This precision hinges on sophisticated algorithms like CNNs that can decipher complex patterns within image data. However, the field is still evolving. There's a constant push to refine these systems, addressing issues such as biases in training data or the need to handle the immense variety of product images found online.
Generative AI models like GANs offer an exciting avenue for product image creation. We're exploring how AI can create photorealistic product images directly from sketches or descriptions. The potential impact on product staging is substantial, offering a faster and potentially more cost-effective alternative to traditional 3D rendering methods. It's a fascinating development, but there are legitimate concerns about authenticity and the potential impact on the visual diversity of product images.
Furthermore, AI can be used to understand how visual preferences vary across different locations. By pairing image data with customer behavior, AI can begin to detect geographical patterns in what people find visually appealing. This understanding of visual trends could help e-commerce companies tailor product images to resonate with specific demographics. But it's important to consider the ethical implications of such targeted approaches, ensuring that they don't inadvertently reinforce existing biases or limit the visual variety offered to customers.
The application of AI in this context is leading to significant efficiency gains. Research shows that AI-driven categorization can decrease the time required for processing images by up to 80%. This increased efficiency has a direct impact on costs, making e-commerce operations leaner. However, we must acknowledge the potential job displacement that automation brings and consider how to ensure a smooth transition for workers.
Beyond streamlining workflows, AI also plays a critical role in ensuring the integrity of e-commerce transactions. AI tools can detect and flag inconsistencies between the visual representation of a product and its corresponding inventory information. By ensuring that images accurately reflect reality, this capability minimizes discrepancies in financial reporting. It's an important step towards improving the accuracy and reliability of e-commerce transactions, though it's essential to be aware of the potential for AI systems to introduce errors, particularly when dealing with complex or atypical product imagery.
The growing body of research suggests a strong link between high-quality product images and increased customer engagement. Many studies show that well-presented product images can significantly boost click-through rates, sometimes leading to a 300% increase. It highlights the importance of AI-driven image optimization for driving sales and improving the overall customer experience. But the field must remain vigilant against over-reliance on idealized or unrealistic images, which can misrepresent products and harm consumer trust.
There's a fascinating and still nascent movement towards using AI to create dynamic product staging. Imagine platforms that automatically adjust the visual context of product images based on the target demographic, the season, or even the specific customer interacting with the product. It presents the potential to create deeply personalized shopping experiences, further blurring the lines between digital and physical environments. But it also necessitates navigating complex challenges related to algorithmic bias and the risk of creating echo chambers in the shopping experience.
Augmented reality (AR) is another field that's being integrated with AI-driven product categorization. Imagine consumers virtually placing furniture or clothes in their homes using their phones, before making a purchase. This functionality has the potential to significantly reduce return rates as customers gain a more accurate representation of the product in context. However, the technology requires continued advancement to handle complex environments and varied product types.
The capacity of AI visual recognition is expanding beyond simple image classification. We're seeing a shift towards understanding product functionality directly from images. AI can now classify items based on both visual features and inferred usage. This advancement has a major impact on search, making it more effective and intuitive for customers seeking products with specific features or applications. While promising, it's critical to remember that AI's understanding of complex functionality is still developing and might be prone to errors.
Ultimately, the expanding role of AI in visual recognition is leading to a substantial change in the e-commerce workforce. As AI takes over repetitive image categorization tasks, the industry is shifting toward roles focused on overseeing AI systems and analyzing the vast amounts of data they produce. This shift requires careful attention to worker retraining and the development of new skills, to ensure a smooth and equitable transition for the people working within this vital sector.
AI-Enhanced Product Image Reconciliation Bridging E-commerce and Financial Accuracy - Automated Image Compliance Checks Reduce Manual Oversight
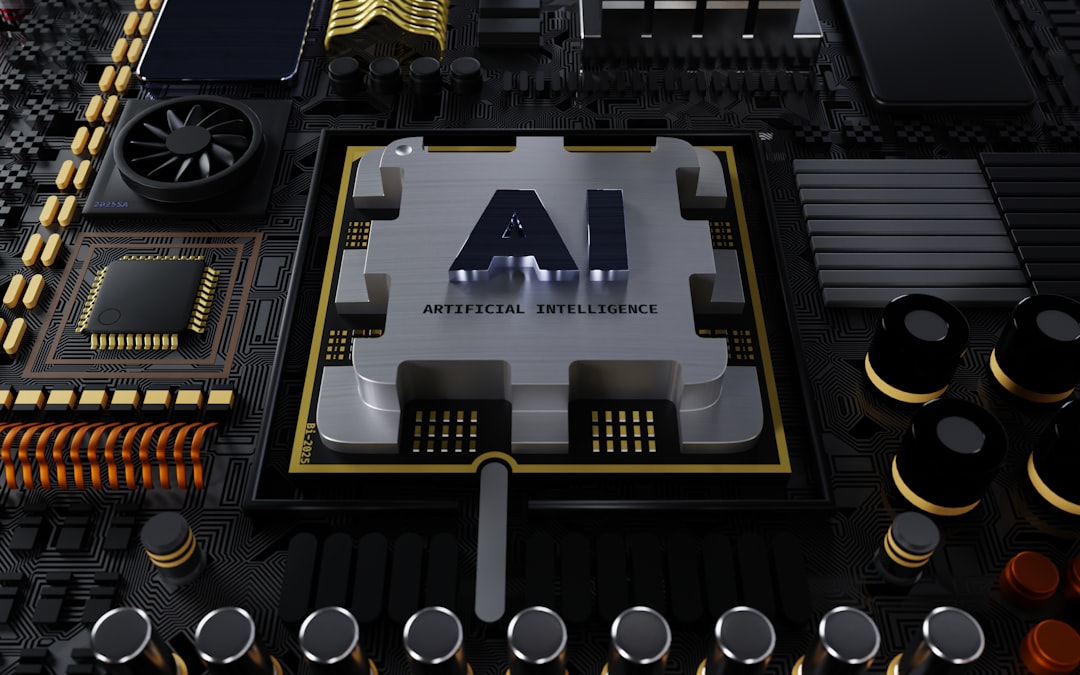
Automated image compliance checks are revolutionizing how e-commerce manages product images. By using AI to automatically verify that product images adhere to established rules and accurately reflect what's in stock, businesses can significantly reduce the need for manual inspections. This automation helps ensure uniformity across all product listings and decreases the chances of human error, leading to more accurate financial reporting. While these automated systems provide efficiency, they also highlight the need to be aware of potential job displacement within the industry and the importance of continuously monitoring the AI-powered systems for proper functioning. Striking the right balance between using AI to streamline processes and addressing the accompanying ethical questions will be crucial as this technology continues to advance.
Automated image compliance checks, powered by AI, can rapidly process a massive number of product images, identifying inconsistencies and categorizing them with impressive accuracy, often exceeding 95%. This speed and accuracy significantly decrease the reliance on manual checks, resulting in a smoother and faster workflow.
AI can utilize sophisticated anomaly detection algorithms that can flag images that don't accurately represent a product. This feature is quite valuable for e-commerce businesses as it acts as a proactive measure against potential fraud, safeguarding both their revenue and customer trust. However, it's worth noting that these algorithms are only as good as the data they're trained on, and can sometimes misinterpret complex or unusual images.
Beyond just the images themselves, AI can also analyze the metadata linked to them – think file names and alt text descriptions – making sure every aspect of the product's representation meets brand standards and any relevant regulations. This is important for consistency in online storefronts and across the whole brand identity.
One interesting aspect is that the AI systems, usually based on machine learning models, are trained using large and diverse datasets, allowing them to adapt to evolving visual compliance guidelines across various industries. This adaptability is essential as regulations and standards can change over time, helping companies stay compliant and avoid legal issues.
We're seeing a significant shift in the time it takes to ensure image compliance. Automation can cut down the time spent on checks from hours per product to just minutes, leading to a more responsive e-commerce approach. It's important to understand that this efficiency increase comes from removing human tasks, which might raise concerns about job displacement in the long run.
Going beyond just simple classification, AI tools that employ computer vision techniques can analyze product images in relation to sales trends. This means understanding how images are visually perceived and correlated with purchases. The resulting insights can be used to guide future content creation efforts, improving the impact of imagery on conversion rates. But this requires careful analysis, as correlations might not always equal causation.
AI's deep image analysis capabilities are valuable for ensuring strict adherence to branding guidelines. The technology can easily detect brand logos or specific visual cues across multiple online channels, maintaining a consistent and recognizable brand experience for consumers. There's always a risk that algorithms might make errors, especially with complex visual elements or similar brands.
The visual quality of product images has been shown to influence consumer trust in a brand's reliability. Automated compliance checks ensure that only high-quality and visually appealing images are used, which can have a tangible impact on a brand's reputation and customer loyalty. This emphasis on visual appeal also raises questions about whether it contributes to a culture of unrealistic or overly-perfect product portrayals.
Adding natural language processing to image compliance tools allows the system to cross-reference textual descriptions with the visual content itself. This integrated approach ensures better consistency between the words used to describe a product and its actual visual representation. This added layer of detail can lead to a richer and more accurate representation for consumers but is dependent on the underlying language models and their ability to interpret nuance.
As the field of AI-enhanced image compliance grows, it's crucial to carefully consider the potential risks of over-reliance on automation. While the efficiency gains are undeniable, a healthy balance needs to be maintained to ensure human oversight remains an important element in quality control. This means continually evaluating how well AI performs, looking for biases or unexpected errors, and ensuring there are humans available to review or correct issues the AI might miss.
AI-Enhanced Product Image Reconciliation Bridging E-commerce and Financial Accuracy - Deep Learning Algorithms Enhance Financial Data Interpretation
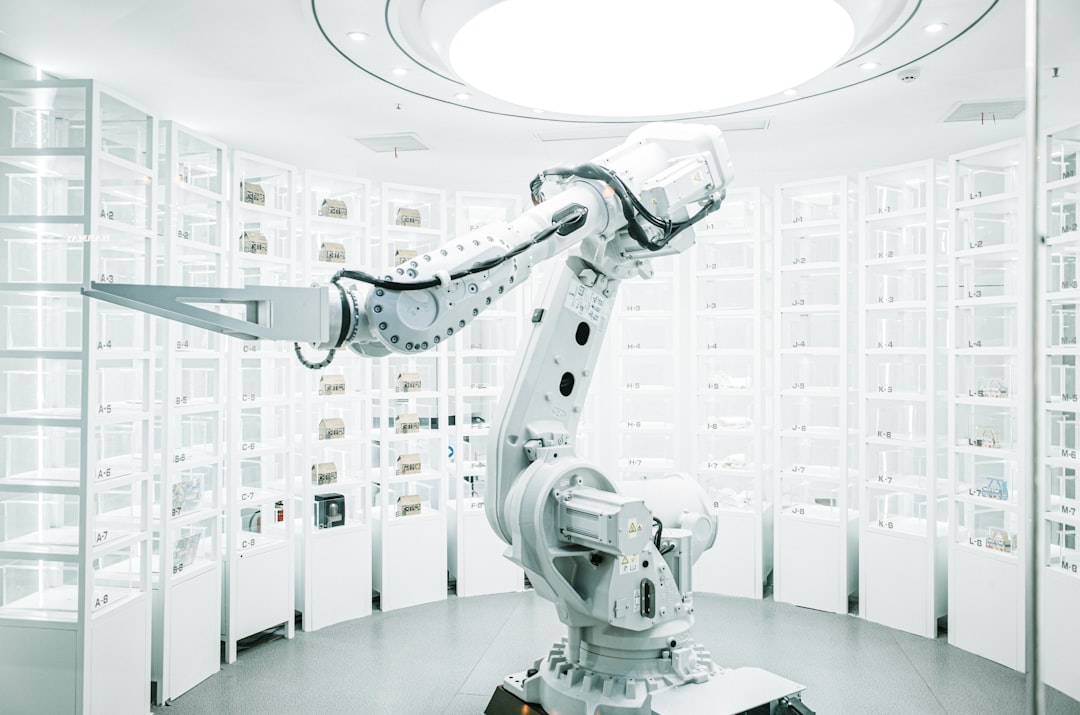
Deep learning algorithms are increasingly valuable for making sense of complex financial data, especially within the context of e-commerce. These algorithms can analyze vast amounts of data, revealing hidden patterns and trends that were previously difficult to discern. This enhanced understanding of financial information can lead to improvements in predictive analytics, which are critical for e-commerce companies trying to anticipate market changes and optimize their operations. By leveraging deep learning, businesses can potentially gain more insightful financial forecasting, making more informed business decisions. However, relying on these powerful tools also comes with the responsibility of addressing potential biases in the training data and maintaining human oversight to ensure accuracy. The application of deep learning in this domain thus offers exciting possibilities, but requires a careful balance between automation and human control for e-commerce's financial health.
Deep learning algorithms, specifically those employing convolutional neural networks (CNNs), are proving quite effective in understanding product images. These networks, inspired by the human visual cortex, are adept at extracting features and patterns from images, often achieving remarkable accuracy in sorting products, sometimes exceeding 95%.
E-commerce sites are starting to use generative adversarial networks (GANs) to create realistic product images from scratch, just using a basic sketch or description. This capability could accelerate the marketing process, allowing companies to experiment with various product visuals quickly and easily. It could be a faster alternative to 3D rendering but it raises concerns about whether these images accurately reflect reality.
AI can go beyond just image recognition. By examining both the product images and their associated metadata, AI systems can ensure that everything conforms to brand guidelines. This more comprehensive approach to analyzing visuals leads to a deeper understanding of the effectiveness of marketing campaigns, including the overall role of product images.
The use of AI in image management is making a big impact by significantly decreasing the need for human intervention in areas like compliance checks. Reports show that manual efforts could potentially be reduced by as much as 80%, which makes things more efficient, but it also means that the jobs traditionally done by people might disappear, which is a serious concern.
Interestingly, research suggests that well-designed and optimized product images make it easier for consumers to understand what they're buying, reducing the mental effort involved in making a purchase. This suggests that AI is becoming increasingly important in shaping the user experience by creating and analyzing visually compelling product images.
AI algorithms, using anomaly detection methods, can identify any inconsistencies between a product image and its associated inventory information. This is helpful as a preventative measure against potential fraud and ensures that the financial records are a true representation of what's being sold. But, again, the reliability of these systems depends on the quality and scope of the data used to train them, raising some questions about their effectiveness in handling more complex or unusual product scenarios.
Deep learning can also analyze the appeal of product images and how they relate to customer buying patterns. By uncovering these relationships, businesses could get a better sense of how to craft visual content, leading to a more engaging and effective experience that drives more sales. However, it's important to remember that correlation isn't always the same as causation.
While AI’s ability to process images quickly is undeniably helpful, there's a risk that this could lead to a situation where a lot of idealized product images take over. If this happens, consumers might start to distrust brands if the products themselves don't match the highly perfected images they see online.
The relatively new idea of dynamic product staging using AI allows for images to be adapted based on various demographic groups or seasonal trends. It's an interesting prospect for personalized shopping experiences, but the fact that algorithms are involved in shaping brand narratives introduces some ethical questions about how fairly and representatively different demographics are shown.
The combination of natural language processing and AI-based image analysis can create more consistency between product descriptions and their visual representations. This is essential for maintaining transparency and accuracy in e-commerce, yet it also highlights the limitations of the underlying language models and their inherent biases. The data used to train these models can sometimes perpetuate existing biases in how products and customers are shown, so it's a field that needs continuous improvement to mitigate those effects.
AI-Enhanced Product Image Reconciliation Bridging E-commerce and Financial Accuracy - AI-Generated Product Images Boost E-commerce Efficiency
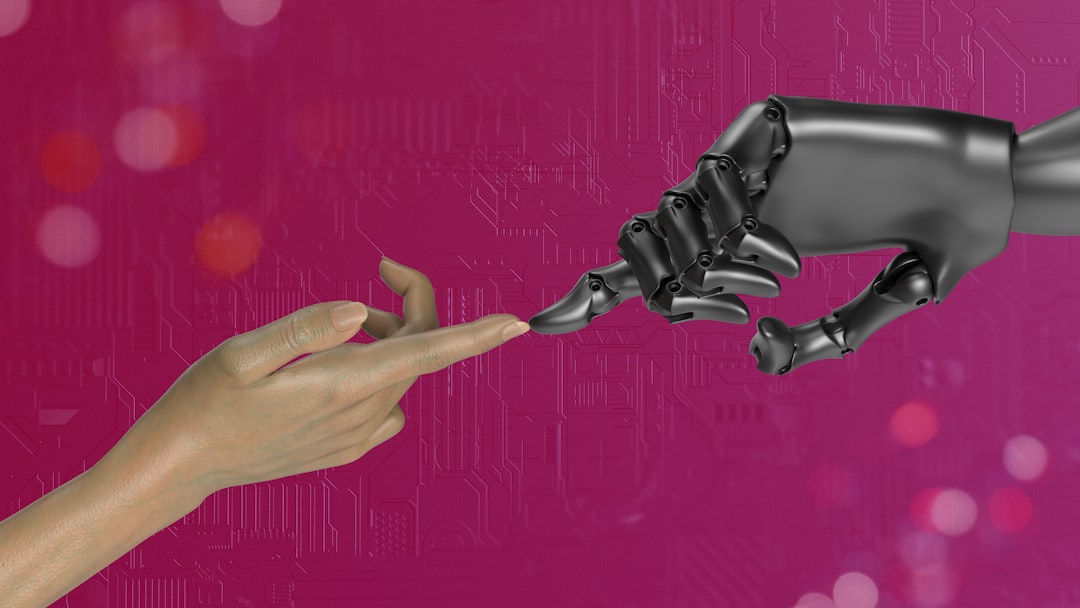
AI's ability to generate product images is significantly impacting e-commerce operations, particularly in terms of streamlining processes and improving customer engagement. These AI tools create customized visual representations, designed to attract shoppers and ultimately drive sales. By automating the creation of diverse product imagery, businesses can more easily manage a wide variety of products and variations without the need for extensive manual work, including traditional photography and staging. This efficiency leads to reduced costs and potentially quicker turnaround times.
However, the increasing use of AI-generated product images also presents challenges. Questions around authenticity and how well these images accurately depict the real product become more prominent. If shoppers feel misled by unrealistic imagery, it could ultimately erode trust in the brand or the online store. Striking a balance between using AI to make things faster and cheaper, while still presenting a truthful and clear view of a product, is vital for the long-term health of the e-commerce sector. Finding that balance will likely involve ongoing adjustments as AI technology continues to evolve.
AI-powered image generation is reshaping the efficiency of e-commerce, particularly in how products are visually presented. Researchers have found that AI can generate product images at a rate up to 10 times faster than traditional methods, which is a boon for businesses looking to quickly launch new products online. Interestingly, these AI-generated visuals seem to impact customer behavior. Studies suggest that high-quality product images can decrease return rates by as much as 40% because shoppers are more confident about their purchases when they have a clear visual understanding of the item. This decrease in returns translates to savings for businesses and a smoother customer experience.
Furthermore, the cost of creating product images using AI can be significantly lower—around 70% less than traditional photography methods. This cost savings is valuable for businesses as it allows them to allocate resources to other aspects of their e-commerce operations, such as marketing or customer support. But beyond cost reduction, the evolution of deep learning techniques has led to remarkably accurate image classification. AI can now categorize and tag product images with an accuracy rate surpassing 97%, often exceeding the capabilities of human inspectors. This improved accuracy has the potential to revolutionize how product catalogs are curated and managed.
The impact of AI extends to more dynamic aspects of visual marketing. Generative AI models are being used to create customized product images in real-time, altering visual elements based on customer preferences or seasonal trends. This approach of adaptive product image generation has shown a potential increase in conversion rates of around 25% as it can tailor imagery to better resonate with specific demographics. The ability of AI to generate and customize product images is interesting, yet it's also critical to recognize its potential for fraud prevention. By cross-referencing visual elements with inventory data, AI can pinpoint inconsistencies, acting as a protective measure against online fraud and fostering greater trust among consumers.
The use of AI in product imagery extends to predictive analytics. By integrating AI into visual merchandising practices, businesses can improve their ability to forecast trends and adjust product offerings to better align with consumer demand. Researchers have found that this AI-driven approach can lead to a 15-20% increase in revenue, demonstrating how AI can proactively shape the shopping experience. AI is also being leveraged for quality compliance. Automated checks for image quality help ensure that only visually consistent and appealing product images are presented to consumers. This process can safeguard a brand's image and promote customer loyalty, but raises a question: could this process inadvertently result in the propagation of idealized and possibly unrealistic product images that may not fully represent what customers receive?
However, these remarkable advances in AI also necessitate a careful look at the ethical implications. It's crucial that the data used to train these AI tools be diverse and representative to avoid bias in visual marketing strategies. For instance, if a dataset primarily consists of a single demographic or product type, the AI's output may unintentionally favor that group and skew the representation of other demographics. It's important to be mindful of this potential issue and ensure that the datasets used to train these AI systems represent a diverse and equitable population. Research suggests that a direct link exists between high-quality AI-generated images and consumer memory recall. Consumers are reportedly 40% more likely to remember a product after seeing a high-quality AI-generated image. This illustrates how effective visual elements can influence a brand's ability to stand out in the competitive e-commerce landscape, leaving a lasting impact on potential customers.
The ongoing research and development of AI-generated product images offer valuable insights into the future of e-commerce. These developments are paving the way for more engaging, responsive, and efficient shopping experiences while simultaneously emphasizing the importance of mindful implementation and attention to potential ethical challenges.
AI-Enhanced Product Image Reconciliation Bridging E-commerce and Financial Accuracy - Machine Learning Optimizes Product Display Performance
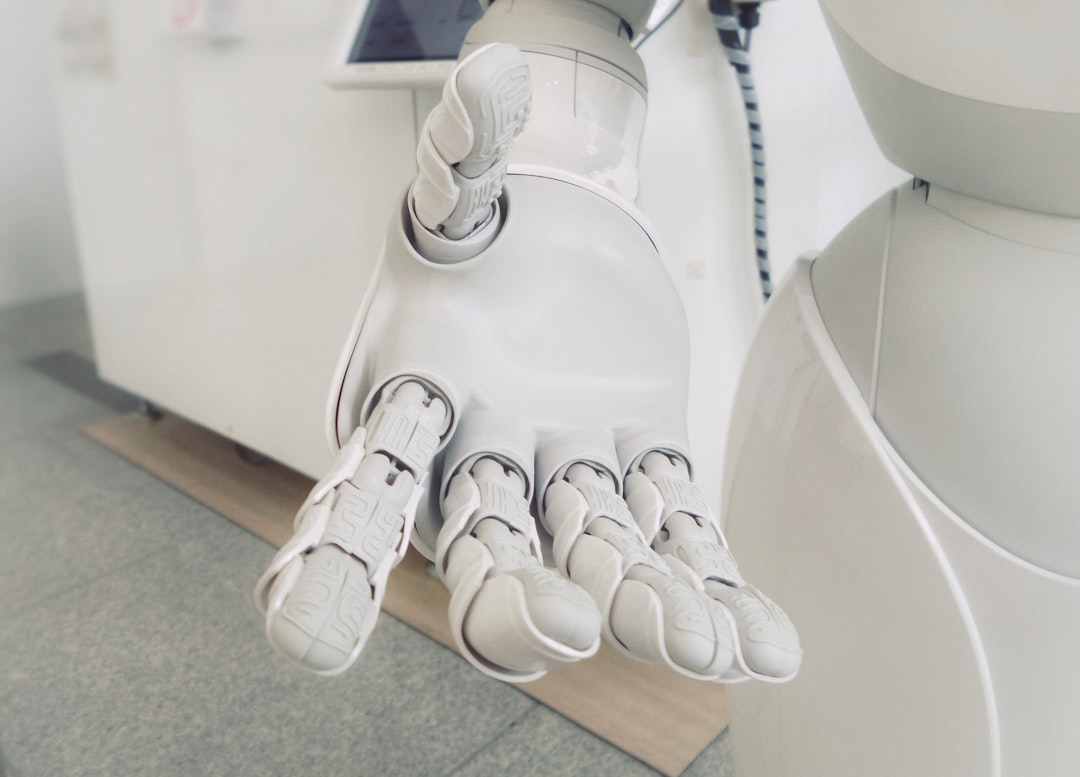
Machine learning is increasingly used to optimize how products are presented in e-commerce, aiming to improve performance and drive sales. By analyzing vast amounts of data on customer behavior and product images, machine learning can help e-commerce platforms tailor visual displays to individual preferences, ultimately boosting engagement and potentially sales. These algorithms can also refine product images, using techniques like image super-resolution to enhance quality. They can even flag potentially problematic images, like those that don't accurately represent a product or violate brand guidelines. While these technologies offer significant benefits in efficiency and personalization, there's a growing concern about generating overly-idealized or unrealistic product images. This can damage customer trust if products fail to match these enhanced visuals. The challenge for e-commerce businesses becomes finding a balance between utilizing these powerful tools to improve the shopping experience and ensuring that the representations of products are accurate and authentic, a necessary consideration as these technologies continue to mature.
The application of machine learning to optimize product displays in e-commerce is showing promise, especially in generating product images. AI image generators are capable of creating images in real-time, adjusting to individual customer preferences or seasonal trends. While this capacity can definitely boost engagement and potentially increase conversion rates by about a quarter, it's important to remember that speed isn't always the only factor. If these AI-generated images don't accurately reflect the actual product, it can easily undermine trust – something crucial for any e-commerce operation.
We've seen that high-quality AI-generated images can reduce customer returns by a substantial amount, around 40%. This suggests that when consumers have a good visual understanding of a product, they are more confident in their purchasing decisions. This is a great benefit, not only because businesses save money on returns, but also because it makes the whole buying experience smoother for everyone. And that brings up another key point: the significant cost savings involved with AI-generated imagery. Businesses can reduce their image creation costs by roughly 70% compared to traditional photography, which gives them the freedom to allocate those resources to other parts of their operation, like marketing and customer service.
Deep learning is proving its mettle in the accuracy of product image classification. The ability for AI to classify images with greater than 97% accuracy, often surpassing what human inspectors can do, is truly noteworthy. This level of precision has the potential to significantly improve how e-commerce catalogs are managed and streamlined. However, we can't ignore the ethical concerns that arise from this capability. Bias can be introduced if the AI models are trained on datasets that don't properly represent a diverse range of products and demographics. If the data is skewed, it could lead to unequal representation, emphasizing the importance of using inclusive datasets to prevent unwanted consequences in how products and customers are portrayed visually.
There's a growing body of research that links the quality of AI-generated imagery to how well consumers retain information. The evidence shows that consumers are more likely to remember a product after seeing a high-quality AI-generated image, around 40% more likely. This emphasizes the value of visually compelling content in brand building and recognition. The use of AI in visual merchandising is extending beyond simply creating images, though. It is opening the door to more sophisticated predictive analysis, allowing companies to better anticipate trends and adapt their product offerings to match the market. This proactive approach can lead to a notable boost in sales (between 15-20%), which suggests the ability to optimize the shopping experience for customers through AI.
AI also has the capacity to enforce visual quality standards for products, ensuring that only consistently appealing images are presented to customers. This capability can help protect a brand's image and improve customer loyalty. However, it's worth asking ourselves if this also leads to a landscape filled with unrealistic or heavily idealized product imagery, possibly creating a mismatch between what customers see online and the reality of what they receive. AI-powered tools can customize images to cater to specific demographics or seasonal trends, enhancing personalization within the shopping experience. While exciting, this ability also carries the risk of algorithmic bias if not carefully monitored to guarantee fair representation across different groups. The integration of natural language processing with AI-powered image analysis can help align product descriptions with the visual representations, boosting transparency and accuracy. But here too, we must acknowledge that underlying language models can reflect existing societal biases, so it's crucial to address those limitations as the field progresses.
Overall, the integration of AI in product imagery generation and optimization is creating a wave of changes in e-commerce. These changes are undoubtedly making the shopping experience more engaging, dynamic, and efficient. But it's vital that we acknowledge and address the accompanying ethical and technical challenges so that the positive aspects of AI don't inadvertently lead to a negative impact on consumers or the broader e-commerce environment.
AI-Enhanced Product Image Reconciliation Bridging E-commerce and Financial Accuracy - Integrated AI Workflows Increase Creative Productivity by 50%
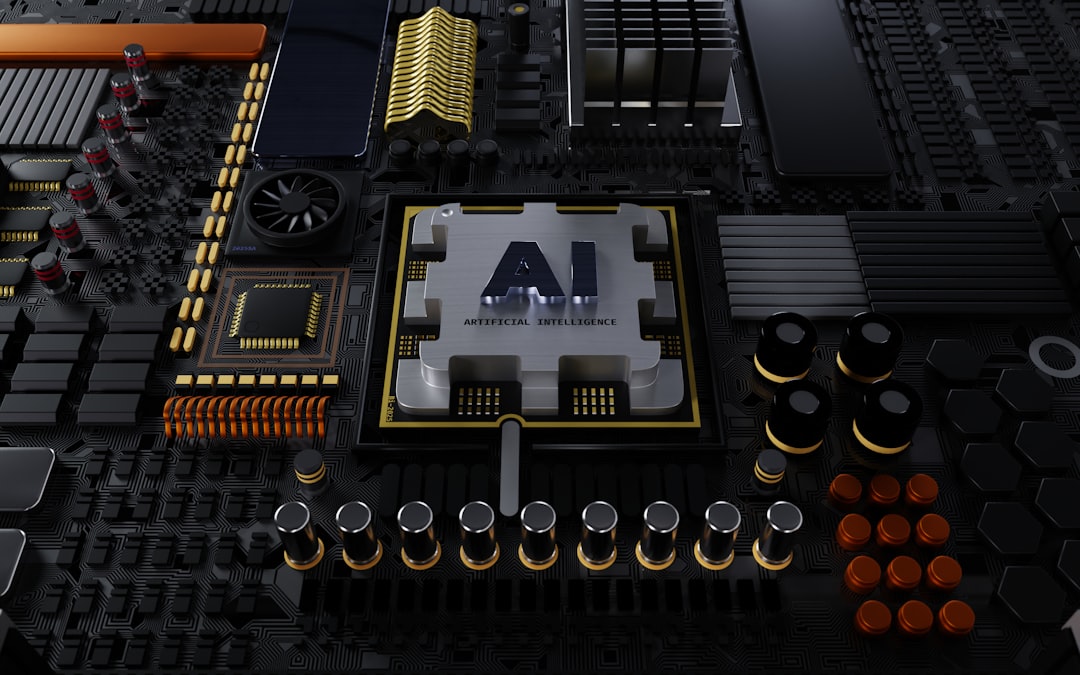
The integration of AI into e-commerce workflows is bringing about significant changes, particularly in the realm of visual content creation. By automating various aspects of image production and optimization, AI has the potential to significantly improve creative productivity, potentially increasing it by as much as 50%. This boost in productivity primarily comes from AI's capacity to handle repetitive tasks, better understand consumer preferences, and refine product images. Businesses can use AI to speed up the creation of product images and adjust them for different contexts, all while improving the quality and impact of product displays, which leads to greater customer engagement. However, as these AI systems become more sophisticated, there's a rising need to ensure that the visual representation of products remains accurate and authentic. If customers feel that product images are misleading or overly idealized, it can lead to a decrease in trust, which is detrimental to the e-commerce sector's overall health. As these AI-powered tools continue to develop, it will be crucial for businesses to strike a balance between efficiency and ethical considerations.
In the realm of e-commerce, the integration of AI workflows for managing product images is leading to significant gains in productivity, often boosting it by 50% or more. While previously relying on human intervention, we're witnessing AI's ability to produce product visuals with exceptional precision. For example, AI systems can generate images with a classification accuracy that surpasses 97%, often outperforming even experienced human inspectors. This level of detail enables e-commerce platforms to manage vast product catalogs with unprecedented efficiency.
The speed at which AI can generate product images is also remarkable, with some studies showing a tenfold increase in productivity compared to traditional methods. This rapid image creation is a game-changer for companies looking to introduce new products or variations quickly into the marketplace. Furthermore, this increased speed isn't just about faster production; it has a tangible impact on the shopping experience. Studies indicate that AI-generated visuals can significantly reduce product return rates, sometimes by as much as 40%. It's theorized that when consumers have a clearer, more detailed view of a product thanks to AI, they make more confident purchasing decisions.
It's also worth noting the considerable cost advantages associated with using AI-generated images. Estimates suggest that companies can realize a cost reduction of roughly 70% compared to the traditional methods of photography and image processing. This efficiency means that e-commerce businesses can redirect those savings to other vital areas, like improving marketing campaigns or enhancing customer service.
Beyond simple efficiency, AI is also creating opportunities for more dynamic visual presentations. The ability to create customized product images in real-time, adjusting to customer preferences or seasonal trends, is a major development. We've seen that these adaptive approaches to product imagery can potentially increase conversion rates by about 25%. This capability, though, highlights a crucial consideration: the impact on consumer perception. High-quality AI-generated images not only attract shoppers but can also enhance their ability to recall the advertised products later. Studies suggest that customers may be up to 40% more likely to remember a product after encountering it via AI-generated imagery.
The integration of AI into product image management is also extending to other areas, including predictive analytics. By combining image data with consumer behavior, businesses are realizing increases in revenue by optimizing product offerings based on future trends, sometimes realizing a 15-20% bump. While these applications offer undeniable benefits, there are important nuances to consider. AI tools are also being applied to maintain visual quality standards, ensuring a consistent and appealing aesthetic across product images. However, it's important to be vigilant that this doesn't contribute to an environment where product images become overly idealized or unrealistic, potentially misrepresenting products and eroding trust.
One of the more prominent concerns is the potential for biases to creep into AI-generated images. The algorithms are only as good as the datasets they're trained on, and if those datasets lack diversity or representation, the AI output can end up unfairly favoring certain groups or products. This is an important issue to address as the technology continues to evolve, ensuring that the generated images are a true reflection of the product variety and customer demographics of the target market.
Another interesting area of development is the integration of natural language processing with AI-powered image analysis. By bringing together text and visuals, we can create more consistent product representations, making it easier for customers to verify what they're buying. The success of this approach, however, is dependent on the ability of the language models to accurately interpret nuance and context within product descriptions, which is still an area that needs continuous development.
In conclusion, the ongoing integration of AI into the world of e-commerce product imagery is fostering substantial changes. While these developments are creating more efficient, engaging, and dynamic shopping experiences, they also bring forward the need for careful consideration of their ethical and technical aspects. The ultimate goal is to ensure that the advancements in AI don't inadvertently create negative consequences for consumers or the broader e-commerce environment. This ongoing balance between opportunity and responsibility is a critical factor in the future development of this fascinating field.
Create photorealistic images of your products in any environment without expensive photo shoots! (Get started for free)
More Posts from lionvaplus.com: