7 Ways AI-Driven Product Photography Tools Slash Studio Costs While Boosting Image Quality in 2025
7 Ways AI-Driven Product Photography Tools Slash Studio Costs While Boosting Image Quality in 2025 - How FluxAI Studio Cut Adobe's Monthly Photo Budget From $50,000 to $4,500 During March 2025
One notable instance unfolding in March 2025 saw FluxAI Studio implement its AI tools, reportedly aiding Adobe in reducing their monthly photo budget considerably, shifting from around $50,000 down to roughly $4,500. This significant reduction points to a changing landscape where automated image generation is handling tasks previously requiring more traditional, and expensive, workflows.
The core technology cited is the FLUX1 model, described as a large AI model specifically designed for generating images, capable of taking detailed prompts and producing varied visual outcomes, including what's termed 'photorealistic' images. The idea is that such models can generate a high volume of images or variations needed for e-commerce product listings or campaigns without the associated costs of traditional photography setups, retouching, or staging.
Access to these tools often comes with various structures; in FluxAI Studio's case, this involves different pricing tiers and a system based on monthly credits to use the generation features. They also offer different versions of their model, like 'Pro' or 'Dev', which might cater to different levels of demand or technical integration. While the cost savings are clearly a major draw, the true test lies in whether the generated images consistently meet the necessary quality standards and creative requirements across diverse product categories, or if the technology still faces limitations in capturing specific textures, lighting nuances, or artistic direction that a human might provide. The promise is reduced cost coupled with boosted image quality, but navigating the practicalities of different model variants and credit systems adds layers of complexity to the adoption process.
Observations from March 2025 pointed to a notable shift in the workflow employed by Adobe for generating its product imagery, reportedly utilizing tools provided by FluxAI Studio. This initiative coincided with a significant decrease in their reported monthly expenditure for photo production, dropping from an estimated $50,000 to approximately $4,500 within that single month. This dramatic reduction is primarily attributed to the strategic integration of advanced AI-driven tools into their established processes. The fundamental approach involved leveraging automated systems to handle tasks traditionally requiring manual intervention, a key characteristic of modern generative AI applications in visual media.
The transition towards this highly automated model appears to center around deploying algorithms capable of processing and potentially generating imagery based on predefined parameters or reference materials. Techniques likely included AI-powered enhancements, adjustments to simulated lighting conditions, and sophisticated background management – whether removal, replacement, or full synthetic generation. While the specifics of maintaining Adobe's known high visual fidelity with such a rapid automation scale require deeper technical analysis, the operational outcome was a streamlining of the image production pipeline. This reduced dependence on extensive physical setups, specialized equipment, and sustained manual post-processing efforts, thereby directly impacting labor and resource costs. The structural change also suggests an architecture enabling faster throughput and scalability without proportionally increasing expenditure, allowing capital to be potentially redirected.
7 Ways AI-Driven Product Photography Tools Slash Studio Costs While Boosting Image Quality in 2025 - Ghost Image Labs Makes Hyper Real Product Shadows Without Studio Lights After Major Update
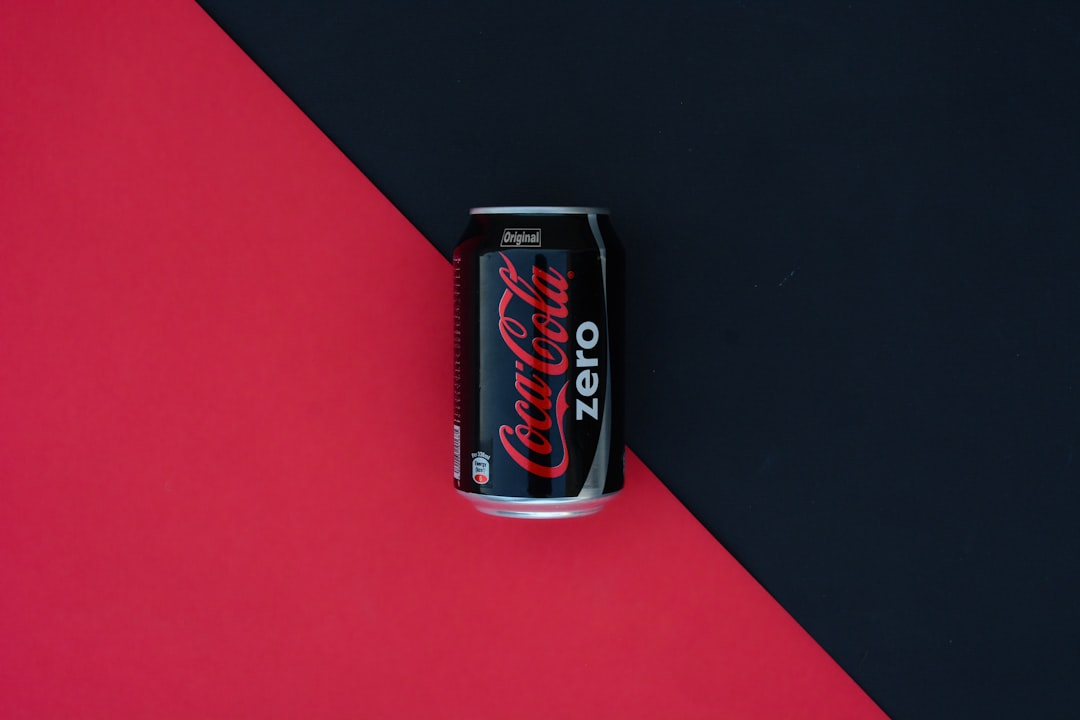
Ghost Image Labs recently introduced a notable update focused on product image generation. The core feature highlighted is the ability to generate what are described as hyper-realistic product shadows without requiring any physical studio lighting setup. Traditionally, crafting accurate and compelling shadows has been a complex part of product photography, often demanding specific lighting techniques during the shoot or considerable effort in post-processing using advanced editing tools to simulate them convincingly. This development shifts that burden, moving the intricate task of shadow creation into an automated, AI-driven process. This capability fits within the broader trend of using artificial intelligence tools to streamline and potentially reduce the resource requirements for creating product visuals. By handling challenging elements like shadow casting computationally, these tools aim to make producing professional-looking images more accessible and less tied to expensive physical setups. However, whether AI-generated shadows can consistently replicate the subtle nuances and interactions of light with various materials and shapes, a skill traditionally refined through experience and artistic judgement, remains a practical consideration for wider application.
Observation regarding a recent development from Ghost Image Labs suggests their tools have been updated, permitting the generation of product shadows that appear highly realistic, ostensibly negating the requirement for physical studio illumination. The implied consequence is a reduction in certain operational costs associated with traditional setups. These AI-powered approaches allow for shadow integration and modification during post-processing. From a workflow perspective, this moves a historically physical setup challenge into a digital manipulation task, potentially altering the image pipeline. The technical goal appears to be enabling visuals that approximate professional studio output without the incumbent investment in physical space, lighting hardware, and associated logistical overhead.
This specific capability is not entirely isolated. Other platforms are also demonstrating facility with digital shadow synthesis. Reportedly, tools from providers like Photoroom or insMind can apply simulated shadows quite rapidly, sometimes within seconds. This points to the computational efficiency achieved. The application spectrum varies; consider scenarios like ghost mannequin imagery where object definition without floor interaction is key versus, say, jewelry or cosmetics, where shadow and light interaction are critical for conveying texture, form, and perceived quality. Generating convincing simulations that capture these subtle interactions accurately across diverse materials remains a technical challenge. Overall, focusing on the specific domain of shadow generation highlights how AI is starting to tackle complex simulation problems within commercial imaging pipelines, presenting alternatives to traditional physical methods.
7 Ways AI-Driven Product Photography Tools Slash Studio Costs While Boosting Image Quality in 2025 - Why DreamStudio Pro Now Auto Generates 360 Degree Spins For Amazon Listings
DreamStudio Pro has reportedly rolled out a function designed to automatically produce 360-degree views of products, with a stated focus on compatibility for Amazon listings. The aim is to generate a set of sequential images or a brief animation that enables online shoppers to virtually inspect an item from multiple perspectives, potentially offering a more complete sense of the product than static images alone. This method relies on automated processes to handle the task of capturing or simulating views from all sides. While giving customers the ability to rotate a product onscreen can enhance visual comprehension, the strategic choice to emphasize this particular format for Amazon at this time might warrant consideration within the context of how platform requirements and interactive display technologies are continuing to develop.
A recent technical development noted among image generation platforms, including capabilities highlighted in tools like DreamStudio Pro, involves using computational methods, presumably based on neural networks and potentially light field synthesis techniques, to produce interactive 360-degree product views. The core idea is to synthesize a seamless sequence of images or perhaps a pseudo-3D representation that allows a viewer to virtually rotate the product online. The anticipated operational benefit lies in speeding up the creation of multi-angle visual data compared to capturing numerous physical photographs. Proponents suggest that allowing potential buyers a more comprehensive view might improve their understanding and reduce uncertainties. However, the technical fidelity of these computationally generated spins, especially regarding fine surface details, reflections, and handling complex geometric shapes, warrants careful examination across diverse product categories. Moreover, the rapidly evolving landscape on major e-commerce platforms, with entities like Amazon reportedly prioritizing the integration of true 3D assets or volumetric data over traditional sequential 360-degree image sets, introduces questions about the long-term technical relevance and required output formats for such spin generation tools.
7 Ways AI-Driven Product Photography Tools Slash Studio Costs While Boosting Image Quality in 2025 - The Rise of Auto Background Removal Through Quantum Computing Advances
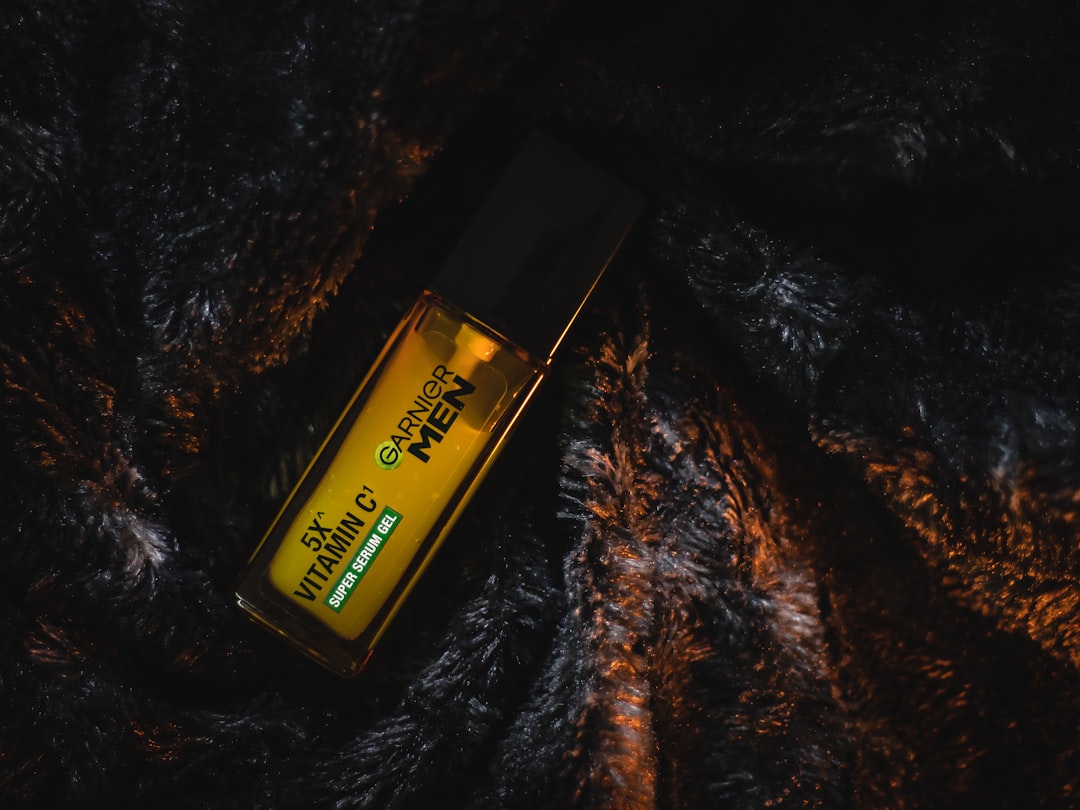
Developments in quantum computing are beginning to influence AI approaches to image processing, offering prospects for more efficient automation, particularly for separating a product from its background. By potentially accelerating the complex computations needed to accurately isolate subjects, even those with fine or intricate edges, quantum-assisted AI could significantly streamline this foundational task in product photography workflows. This streamlining targets reducing the manual effort and associated costs involved in preparing images for online listings. However, while the theoretical speed and precision gains are notable, the practical hurdle lies in consistently applying these advanced techniques across the vast array of product types and textures found in e-commerce, ensuring the output maintains high fidelity and artistic standards without introducing visual inconsistencies. Assessing their reliable performance across this diversity is an ongoing point of focus as this technology develops.
Looking into the confluence of quantum computing and image processing reveals some intriguing possibilities for automated tasks like background removal, a perennial challenge in preparing product visuals for online presentation. The fundamental ability of quantum systems to potentially handle complex computations on vast datasets simultaneously is where the perceived advantage lies. Instead of processing image pixels sequentially or in limited parallel streams as classical computers do, theoretical quantum approaches could manipulate multiple pixel states concurrently by leveraging phenomena like superposition and entanglement.
This parallel processing capability, if harnessed effectively through quantum algorithms tailored for image analysis, could theoretically accelerate tasks like image segmentation – the core process of distinguishing a foreground object from its background. The hope is that quantum algorithms might not only be faster for certain complex operations but potentially more adept at identifying subtle edges and textures, leading to cleaner, more precise cutouts, even with challenging subjects or intricate details.
Furthermore, some research directions suggest that quantum machine learning models might require significantly less training data than their classical counterparts to achieve proficiency in pattern recognition relevant to image analysis. If true, this could ease the burden of curating enormous annotated datasets, which is a substantial cost and time sink in developing traditional AI image tools. There's also speculation about how quantum methods might enhance related elements, like generating more realistic simulated shadows or better capturing depth information, contributing to a more compelling final image without extensive physical setups.
However, it's critical to ground these potential benefits in the current reality of quantum technology development. As of early 2025, building and maintaining quantum computers capable of executing complex algorithms reliably for practical applications remains an immense engineering hurdle. Issues like qubit decoherence – the instability of quantum states – are still significant limitations that make running production-scale image processing tasks on quantum hardware unfeasible today. While fascinating from a research perspective, the widespread integration of purely quantum solutions for something as routine and high-volume as e-commerce background removal appears to be a considerably longer-term prospect, likely requiring further fundamental breakthroughs or the successful development of robust hybrid classical-quantum systems. The discussion highlights a cutting edge area of research, but one where significant practical implementation challenges persist.
7 Ways AI-Driven Product Photography Tools Slash Studio Costs While Boosting Image Quality in 2025 - How Nike's New AI Camera Rig Creates Studio Quality Shots In 3 Seconds
Nike's deployment of a specialized AI-powered camera rig highlights an emerging approach to generating product visuals rapidly. This system is reported to capture images suitable for professional presentation, potentially achieving studio-level quality, in a remarkably short timeframe – specifically noted as just three seconds. This kind of capability points toward streamlining what has historically been a time-consuming and resource-intensive process involving intricate lighting, setup, and multiple shots. Leveraging artificial intelligence in this way allows for the potential automation or significant acceleration of image capture, aiming to meet the high-volume demands of online retail by providing quick access to quality visuals. As brands continue to integrate AI across their digital operations to boost efficiency, tools like this rig exemplify the shift towards automated capture solutions. However, despite the impressive speed, a practical question remains regarding how consistently such a rapid process can adapt to the unique requirements of different product materials, textures, and forms while preserving creative control and fine detail nuances expected in high-quality commercial imagery.
Recent observations point to initiatives within larger organizations leveraging AI not just for generating imagery from scratch, but for potentially accelerating and standardizing the physical capture process itself. Nike, for example, is reportedly utilizing a novel AI-driven camera rig system. The core claim is the ability to produce output described as "studio-quality" images from a physical product setup in a remarkably short timeframe, specifically cited as around three seconds per shot. From an engineering perspective, this suggests a tightly integrated system where capture, preliminary processing, and perhaps some initial automated adjustments or segmentation occur almost simultaneously.
Delving into the reported capabilities, the system ostensibly incorporates advanced depth sensing, crucial for precise focus and composition without extensive manual setup. It also reportedly employs adaptive algorithms that analyze ambient light and adjust integrated artificial lighting in real time. This could significantly mitigate variability and simplify the shooting environment, though maintaining consistent high fidelity across diverse materials and surface types under such rapid adjustments poses interesting technical challenges. Furthermore, the rig is said to utilize machine learning for enhanced texture recognition, which is critical for accurately rendering details like fabric weaves or complex reflections – areas where automated systems have historically struggled compared to experienced human photographers manipulating light and camera settings.
The workflow implications are significant. Reports suggest the rig can handle automated background processing, potentially involving rapid removal or substitution, and may capture data for multi-angle views concurrently. This contrasts with sequential manual capture or purely generative approaches discussed elsewhere and points towards a system optimized for high-volume throughput. Real-time quality assessment is also mentioned, allowing for immediate evaluation of technical parameters like focus and exposure. While promising for efficiency, the definition of "studio quality" and the rig's versatility across Nike's vast and varied product range—from performance footwear with intricate textures to apparel with subtle drapes—in such constrained timeframes warrants careful technical scrutiny. The system is also noted for features aimed at streamlining post-production workflow, such as automatic formatting for e-commerce platforms and potentially using historical performance data to guide shooting parameters, suggesting an approach focused on optimizing the entire visual asset pipeline from capture through online deployment.
More Posts from lionvaplus.com: