7 Quantifiable Ways AI Image Generation Reduces Product Photography Costs in 2025
7 Quantifiable Ways AI Image Generation Reduces Product Photography Costs in 2025 - Studio Equipment Cost Drop As DALL-E 4 Creates Multiple Product Angles Under $2
A significant economic factor impacting product visualization in 2025 is the arrival of tools capable of delivering multiple product views for very low costs. Systems like DALL-E 4 offering the generation of various product angles for less than two dollars apiece are fundamentally challenging the established costs of traditional product photography. This dramatic price point directly diminishes the need for extensive investment in physical studio equipment, such as lighting rigs, high-end cameras, and elaborate backdrops. The financial requirement for renting space or maintaining a dedicated in-house studio, along with the associated depreciation and logistics of managing physical gear, becomes less compelling when comparable visual assets can be produced digitally on demand. This shift allows businesses to reallocate resources previously tied up in physical production towards potentially more impactful areas like marketing channel diversification or enhancing the digital customer experience. While still maturing and not a perfect fit for every creative need, the cost-effectiveness and speed of generating numerous standard product angles digitally represent a major change in operational budgets for companies needing high-volume product imagery.
The current iteration of tools like DALL-E 4 presents an interesting economic model for image generation compared to the traditional capital expenditure and operational costs of a physical studio setup. Observing the pricing structures, such as the roughly 115 credits offered for $15, we see a system where generating images, including variations and different perspectives of a single product, can fall below a couple of dollars per output sequence. This moves the core expense from acquiring and maintaining tangible assets – cameras, lighting rigs, backdrops – and coordinating physical labor, towards a computational utility cost.
Looking ahead from 2025, the implication of this shift is not merely a minor saving but a potential restructuring of how visual assets are produced, particularly for e-commerce at scale. By drastically reducing the dependency on rented or owned studio equipment and the associated logistical burden, businesses are presented with an opportunity to reallocate capital. The efficiency gain here isn't just in speed, but in removing entire cost centers related to physical space and specialized hardware. However, it's worth noting that while the equipment cost might evaporate for certain use cases, the challenge shifts to defining precise visual requirements computationally, which itself can require new skills and iterative refinement that might not always be trivial or perfectly captured by a low per-image generation cost. The economic calculus becomes one of compute cycles and prompt engineering effectiveness versus traditional photographic expertise and physical resources.
7 Quantifiable Ways AI Image Generation Reduces Product Photography Costs in 2025 - Zero Background Setup Fees Through Microsoft Designer Product Generator
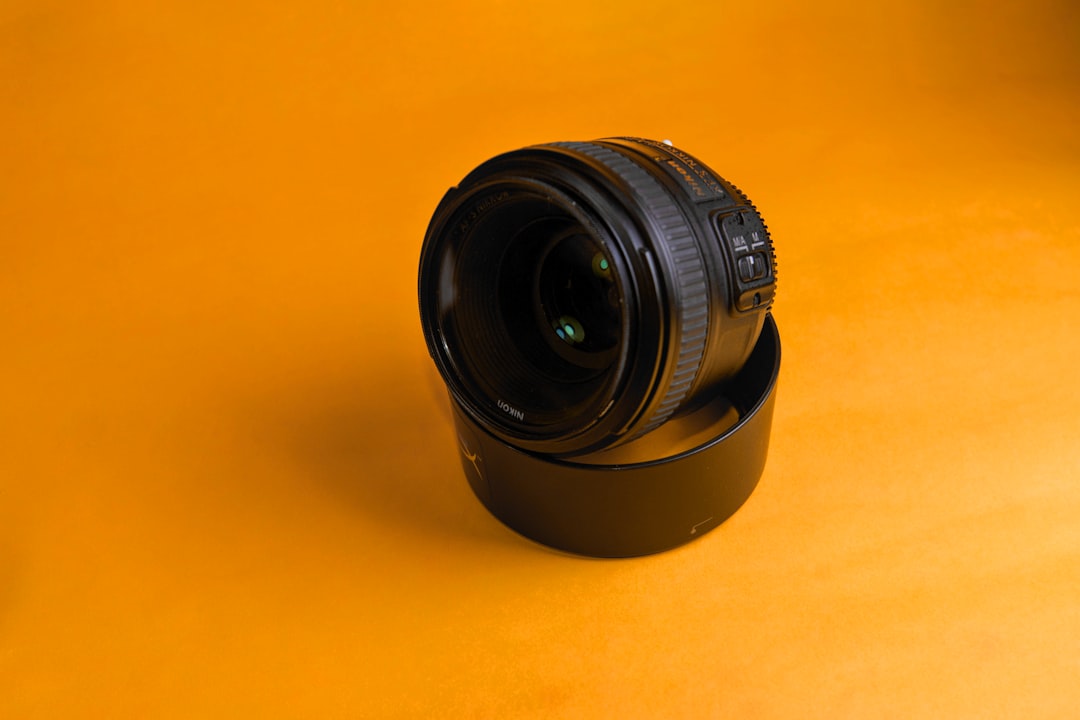
Simplifying the creation of product visuals by tackling the common challenge of backgrounds is a key aspect of some AI tools in 2025. Platforms like Microsoft Designer, offering a product generator function, approach this by allowing users to describe the desired image, effectively bypassing the need for physical backdrops or complex lighting setups. The core idea is to eliminate the time, effort, and cost associated with traditional studio staging purely for the sake of the background environment. Generating images this way reduces dependency on hiring photographers specifically for shoots requiring clean or styled backgrounds, thereby cutting down associated fees. The inclusion of features designed to isolate the product further streamlines the post-production workflow often needed to remove or refine backgrounds from conventionally captured images. While promising accessibility and cost reduction during its current phase, this method relies heavily on the user's ability to articulate the visual requirements through text prompts and the AI's capacity to interpret them accurately, which isn't always a perfect or immediate process, potentially requiring iteration and adjustments to achieve the desired result.
The notion of eliminating physical background staging costs through artificial intelligence generators presents an interesting departure from conventional product photography workflows. Observing tools like Microsoft Designer, powered by systems akin to later DALLE iterations, we see a mechanism for generating or placing products against digitally constructed environments. This effectively bypasses the need for procuring, setting up, and managing physical backdrops, props, or location rentals. From an engineering perspective, the complexity shifts from coordinating physical light, material properties, and spatial arrangements in a studio or on location, to defining desired scene attributes and product placement via textual prompts or digital interfaces. The promise here is a significant reduction in the operational expenditures tied directly to the tangible aspects of creating a scene for a product.
Investigating further, this digital approach offers inherent flexibility and scalability that is difficult to replicate physically. The capability to instantaneously swap a product from one simulated environment to another, adjusting scene elements with simple inputs, allows for rapid iteration and testing of various visual contexts. This contrasts sharply with the time and expense involved in dismantling and reassembling physical sets. Furthermore, for operations requiring high volumes of imagery across diverse product lines, removing the physical setup constraint means production isn't limited by studio availability or the manual labor of staging each shot. While the execution might not always perfectly replicate the subtle realism achieved by expert physical staging and lighting, the removal of this specific cost center, and the resulting increase in digital manipulation flexibility, represents a notable change in the economic model for producing certain types of product visuals.
7 Quantifiable Ways AI Image Generation Reduces Product Photography Costs in 2025 - Seasonal Campaign Costs Reduced By 80% With Midjourney Business Package
Focusing specifically on seasonal marketing initiatives, the advent of AI platforms like Midjourney providing tiered access for businesses introduces a distinct economic model for creative asset production. Reports suggest significant reductions in seasonal campaign costs, with some estimates reaching as high as 80%. Unlike per-image computational costs or tools primarily focused on background manipulation, this approach typically involves a fixed monthly subscription, often ranging between $30 and $60 depending on usage scale. This structure enables the rapid generation of a high volume of diverse visual concepts tailored to specific seasonal themes, often within minutes. Nevertheless, successfully translating intricate campaign narratives and achieving precise, consistent brand aesthetics across numerous AI-generated images still requires considerable skill and iterative refinement in the prompting process, presenting its own set of challenges compared to directing a physical shoot. This shift represents a reallocation of resources from traditional production logistics towards computational expertise and creative AI guidance in 2025.
Analysis of AI image generation platforms like Midjourney indicates a notable impact on the cost structure of producing visual assets for seasonal campaigns. Reports circulating as of May 2025 suggest that businesses leveraging these tools have seen reductions in expenses, in some cases reportedly up to 80%. This observed efficiency gain appears tied to bypassing many traditional costs associated with physical product photography and staging for specific promotional periods.
From an operational standpoint, the cost to access such capabilities typically involves a subscription fee. Current observed pricing models range from around $30 to $60 per month for standard access, with larger organizations exceeding a certain revenue threshold (reportedly over $1 million annually) often directed towards a higher-tier 'Corporate' package. This structure represents a shift from potentially variable project-based physical production costs to a more predictable, subscription-based computational utility expense.
The speed of output is a significant factor in this cost dynamic. The platform offers different generation modes, including rapid 'fast hours' allowing for image creation in mere minutes. This accelerated turnaround time is particularly impactful for time-sensitive seasonal campaigns, enabling quicker iteration and deployment of diverse visual content compared to scheduling and executing traditional photoshoots. This capability also allows for greater flexibility in tailoring visuals precisely to campaign themes or specific audience segments without incurring significant additional time or financial overhead for reshoots. While the ability to quickly generate multiple variations holds promise for iterative design and testing, the consistency of output across these rapid generations and the effectiveness of translating complex staging ideas into prompts remain ongoing technical considerations. Furthermore, this approach inherently reduces the need for many traditional labor components involved in a physical shoot – photographers, stylists, set builders – redirecting resource allocation. The platform's ability to facilitate exploring novel or imaginative staging concepts digitally could also allow businesses to pursue creative directions previously deemed too expensive or logistically challenging with physical methods.
7 Quantifiable Ways AI Image Generation Reduces Product Photography Costs in 2025 - One Click Product Variations Replace Manual Color Adjustment Budgets
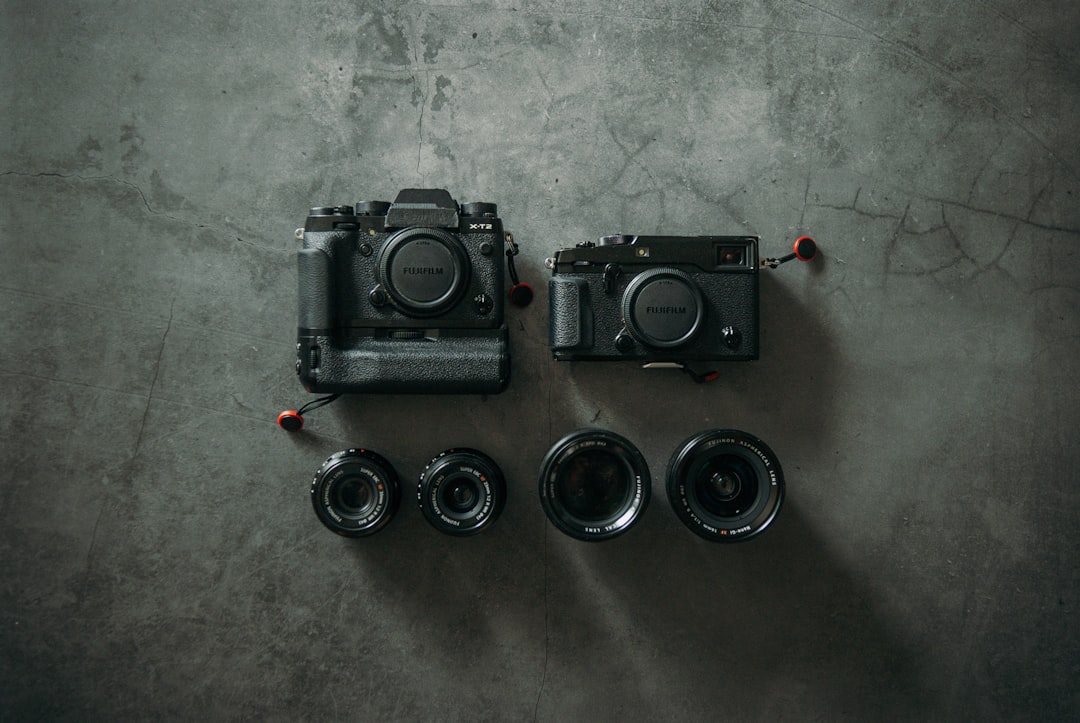
Producing imagery for every single color variation of a product used to require significant time and expense dedicated specifically to post-production adjustments. Manually tweaking hues, saturation, and luminescence across potentially dozens or hundreds of individual image files consumed considerable budget allocated for editing labor. By 2025, advanced AI image generation and editing platforms are offering capabilities that fundamentally alter this process. These tools now allow for the near-instantaneous generation of multiple color variations of a product within an existing image, often through simple, highly automated functions described as 'one-click'. This capability directly replaces the need for extensive manual color correction work. Instead of paying editors for hours spent isolating products and carefully adjusting colors one by one, businesses can leverage these AI features to generate the required variations rapidly. While these automated tools dramatically reduce the labor and associated costs for straightforward color swaps, achieving nuanced or highly specific color rendering across complex textures or materials can still sometimes require iterative prompting or adjustments, presenting a different kind of workflow challenge. Nevertheless, the direct elimination of a dedicated budget line item for manual color variation editing is a tangible cost reduction for businesses requiring diverse product representation in their visual assets.
The ability to generate numerous product variations near-instantly, particularly differing colors, represents a significant pivot in how product visuals are created as of May 2025. This moves away from the labor-intensive manual process of adjusting images or the logistical complexity of staging physical products in every conceivable color option. Algorithms can now quickly render a digital product asset in a spectrum of colors, bypassing the need for retakes or extensive post-production work on traditional photographs.
These systems also aim to bake in a degree of visual uniformity. By applying learned parameters, they attempt to maintain consistent color representation and style across vast collections of images, crucial for maintaining brand identity. However, achieving subtle color fidelity or ensuring consistent visual weight across a diverse range of hues programmatically still requires careful oversight and refinement in the generative process.
There's also the intriguing possibility this offers for visualizing product lines earlier in the development cycle. Generating photorealistic images of different color concepts, for instance, can be done without the time and cost associated with creating physical samples or prototypes, potentially accelerating design exploration and reducing material expenditure before committing to manufacturing.
Beyond just color, these tools offer enhanced flexibility in presenting the product within simulated environments. While the removal of basic background setup was noted previously, the dynamic capability here lies in the ability to quickly place the same digital product model into a wide *variety* of different staged contexts or scenes virtually. This enables showcasing versatility or targeting different consumer demographics with tailored visual narratives without the physical logistics and costs tied to transporting and staging items repeatedly for traditional shoots.
This transformation naturally affects traditional roles, potentially reducing the demand for standard, static product photography labor for high-volume catalog needs. The sheer scale and speed of output are notable; producing thousands of product visuals, covering all required variations for a large e-commerce inventory, can be achieved in a fraction of the time compared to conventional studio workflows.
From an engineering perspective, the ability to manipulate attributes like color or simulated size within the digital image asset itself opens possibilities for more interactive product displays online, allowing customers to customize visualizations, though integrating this real-time rendering into front-end retail platforms is an ongoing challenge. Analyzing the observed unit costs, some reports suggest generation costs can fall significantly lower than traditional methods – figures like $0.15 per image have been cited when amortized over certain usage models. This contrasts sharply with the typical per-shot costs in conventional photography, though it's important to recognize this metric often doesn't fully capture the investment in initial model creation, iterative prompting expertise, and computational resources required to achieve a satisfactory output. Furthermore, the inherently structured nature of these digital images makes them potentially more amenable to processing and optimization for visual search algorithms, which rely heavily on analyzing image attributes like color and form.
More Posts from lionvaplus.com: