7 Proven AI Techniques for Converting Traditional Economy Illustrations into Dynamic Product Visuals
7 Proven AI Techniques for Converting Traditional Economy Illustrations into Dynamic Product Visuals - Smart Background Removal Transforms Basic Product Photos into Professional Studio Shots
The ability to swiftly remove and replace backgrounds has become a game-changer in product photography for online stores. These smart tools are transforming ordinary product shots into professional-looking images that would normally require a dedicated studio setup. Software like Photoleap and Pincel offer users options to effortlessly swap out backgrounds with a range of scenes – from urban backdrops to natural landscapes. This capability enhances the visual appeal of products and aids in creating effective marketing materials. By allowing businesses to experiment with different environments and styles, these AI-driven tools deliver greater flexibility for showcasing items in a more compelling way for various online channels. The ongoing development of this technology promises to significantly lower the barrier to entry for businesses that aim for higher quality visuals, regardless of their resources or design expertise. While the results can be impressive, it's worth noting that the tools themselves are constantly evolving, and their effectiveness may vary depending on the image quality and desired outcome.
It's fascinating how these AI-driven background removal tools are revolutionizing product photography. We can now effortlessly transform basic product shots into sophisticated studio-style images simply by replacing or removing the background. Tools like Photoleap are particularly interesting, offering the ability to seamlessly integrate products into diverse digital environments, from bustling cityscapes to tranquil natural landscapes.
Services like Pixyer take it a step further, not just removing the background but also optimizing the image specifically for e-commerce platforms and marketing materials. Other tools, such as Pincel, offer a similar level of scene creation, providing a fast and accessible route to professional-looking images. There's also Magic Studio, whose 'Create AI Product Images' feature demonstrates how AI can specifically target e-commerce needs.
Platforms like iFoto highlight the growing sophistication of these tools – they can improve image quality before generating a new background, supporting multiple file formats. The trend is clear: companies like Pebblely are building entire systems around AI-powered background generation, removing the need for manual edits or traditional photography setups.
Beyond the background swap, there's a remarkable level of control emerging. You can influence color palettes to match brand guidelines, leading to more cohesive visuals. And we're not just limited to a single background, either. Tools can create a whole series of different scenes, providing immense flexibility for marketing and online store layouts.
Ultimately, this integration of AI is streamlining the entire workflow, making professional-looking visuals more attainable for businesses. The barrier to entry is decreasing, and this opens up opportunities for more small companies to compete on a visual level previously only available to larger companies. It remains to be seen exactly how this wave of technological innovation will continue to impact the landscape of product presentation and online sales.
7 Proven AI Techniques for Converting Traditional Economy Illustrations into Dynamic Product Visuals - AI Scene Generation Places Products in Custom Digital Environments
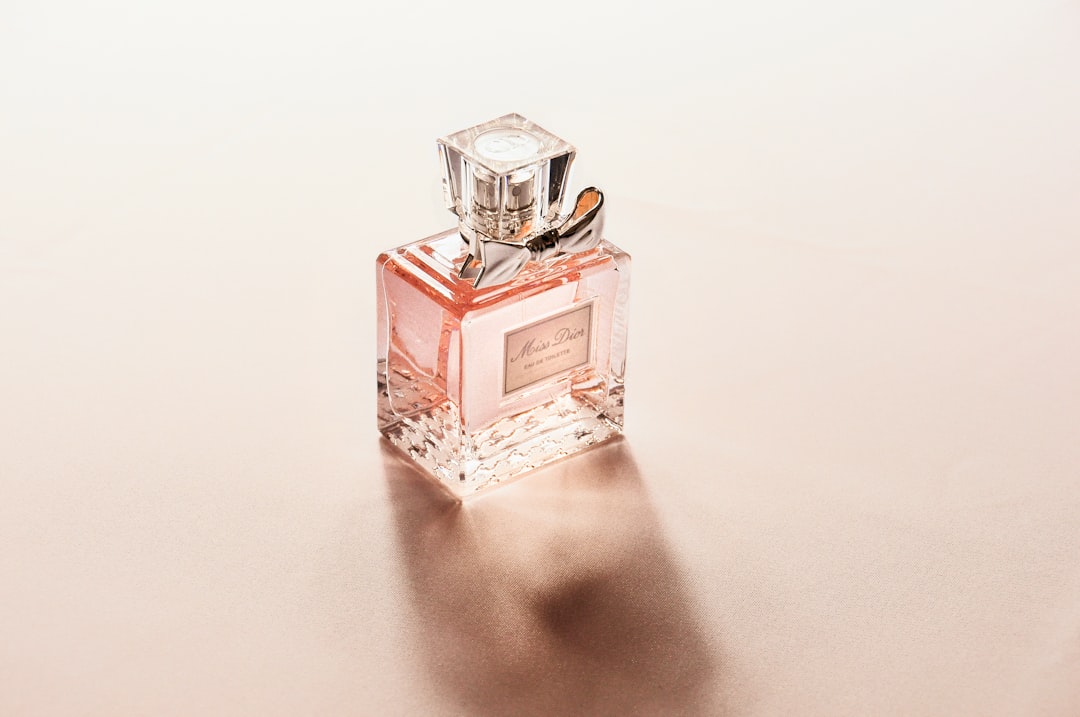
AI is now capable of placing products within custom-designed digital scenes, transforming how we visualize them online. This technology uses methods like inpainting and text-to-image generation to seamlessly integrate products into unique environments. Essentially, AI can now create entirely new contexts for products, allowing businesses to showcase them in ways that were previously difficult or expensive.
Imagine being able to describe a scene, like a cozy living room or a bustling city street, and having AI automatically place your product within that scene. This is becoming a reality with new tools like Google's Product Studio, which utilizes text prompts to create the desired product image. Beyond just enhancing the look and feel of product images, these techniques also streamline the entire process of creating visuals, reducing time and cost.
This ability to customize environments gives businesses more creative freedom when presenting their goods. They can adapt product visuals for different platforms and audiences, ensuring a consistent and engaging brand experience across their online presence. However, the quality of the generated images and the potential for them to look artificial remain a concern. As this field rapidly develops, we need to pay close attention to ensure the output stays realistic and helps strengthen the brand, rather than diluting it. This evolution of visual presentation has the potential to significantly impact e-commerce, although the long-term consequences and the evolving standards for image quality are still uncertain.
AI is making it possible to create incredibly realistic product scenes digitally. Just a few years ago, this level of visual quality would have required a lot of time, effort, and money using traditional photography methods. As AI algorithms get smarter, the backgrounds they create can mimic the details and lighting of real environments, making product images much more attractive to buyers.
These capabilities are based on sophisticated neural networks that learn from countless images. They not only understand what makes a scene visually engaging but can automatically design new backgrounds that match the product's style and the overall message you want to convey.
Research suggests that customers are more likely to buy a product when it's shown in a realistic setting. In fact, some studies show conversion rates can increase by up to 30% when using AI-generated environments compared to standard product shots. This underlines the importance of showing a product within a relevant context when selling online.
Some AI image tools even use augmented reality (AR) concepts. This allows shoppers to place a product in their own spaces before deciding to buy. This can greatly improve engagement and, in some cases, significantly reduce returns.
However, it's important that these AI tools place products in a way that makes sense within the generated environment. If things are positioned in an unnatural way, it can raise questions about the product's quality in the minds of customers. So, it's key for these AI systems to be able to grasp the relationships between objects and how they look in 3D space.
One of the things that's helped make these tools so popular is their ease of use. Many are designed to be accessible, even for people with no graphic design experience. This means even smaller companies can use them to compete visually in the e-commerce world.
The technology continues to advance and is getting better at seamlessly incorporating things like product dimensions, colors, and textures for a harmonious look. Some tools can even suggest the best settings for a product based on its type or the target customer, further simplifying the design process.
It's important to acknowledge the data dependency of these systems. The accuracy of the generated scenes really depends on the quantity and diversity of images the AI was trained on. If the training data isn't good, the results can be strange or inappropriate, possibly hurting a company's reputation.
The ongoing evolution of AI is fostering a move toward more personalized marketing visuals. Images can be tweaked based on user behavior and individual preferences, potentially leading to higher engagement and sales.
Lastly, the growth of AI scene generation tools introduces new complexities concerning intellectual property. Since these systems create unique visual content, there are questions about ownership and copyright. Businesses using AI-generated images may face unforeseen legal challenges in the future.
7 Proven AI Techniques for Converting Traditional Economy Illustrations into Dynamic Product Visuals - Machine Learning Algorithms Enhance Product Textures and Materials
Machine learning is now influencing how we create and present product textures and materials, particularly within the context of online shopping. These algorithms are trained on vast amounts of data, allowing them to understand the relationships between different materials and their visual properties. This understanding enables designers to predict how materials will behave under different lighting and conditions, ultimately leading to more realistic and visually compelling product images.
The advantage of using these algorithms is that it can significantly shorten the development process for new materials and textures. Instead of relying on time-consuming trial-and-error, designers can use AI to quickly evaluate and refine material choices, leading to cost savings and faster product launches. This access to sophisticated material design tools potentially levels the playing field for smaller businesses that may not have the resources to invest in traditional material development methods.
Furthermore, the application of AI in this domain allows for a higher degree of customization. It becomes easier to design materials that meet very specific requirements, whether it's achieving a particular sheen, mimicking the look of a natural material, or even creating entirely novel textures. This creates possibilities for unique product aesthetics, which in turn can enhance a product's appeal and differentiation in the crowded online marketplace. However, it is crucial to remember that the success of these algorithms hinges on the quality and diversity of the data used for training. If the data is biased or limited, the outcomes may not be representative of the real world, leading to suboptimal design decisions or unrealistic product representations. While these algorithmic approaches promise a future of richer, more engaging product imagery, it's essential to acknowledge the challenges and limitations inherent in the technology as it continues to develop.
Machine learning algorithms are becoming quite good at analyzing the textures found in product images. They can recreate the intricate details of materials like fabric, wood, and metal with impressive accuracy. This ability is improving the online shopping experience by giving shoppers a better idea of what the products and their textures would look like in person, even from a distance.
Recent breakthroughs in convolutional neural networks (CNNs) are allowing for the creation of product images that not only show realistic textures, but can adapt to changing lighting conditions. For example, the same product could be shown in sunlight in one image and under softer indoor lighting in another. This flexibility broadens a product's appeal in the marketplace.
The ability to generate textures using machine learning offers tangible advantages for businesses. By producing high-resolution images of different material finishes, companies can rapidly test various marketing approaches without needing to create physical prototypes. This speeds up the time it takes to get products to market.
Algorithms can examine user interaction data to identify which textures and materials are most appealing to potential customers. By adjusting the visuals of their products to these preferences, businesses can potentially increase sales, as they are now using data to guide their marketing approach.
Machine learning approaches allow for the simulation of complex textures, such as the reflective nature of glass or the soft feel of velvet. This can result in images that more effectively engage buyers by appealing to a more tactile sense, even though the product is only seen on a screen.
Research into how the human brain reacts to textures in product photos indicates that images with visually complex textures can create an impression of luxury and high quality. This insight helps AI systems fine-tune product images by focusing on surface details that match customer's psychological preferences.
Certain machine learning models utilize GANs (Generative Adversarial Networks) not just to make realistic product images, but also to test out different textures and materials. This gives companies a larger range of choices to test in their marketing without spending a lot of money or taking a long time to do it.
Texture transfer algorithms are capable of applying one visual style to another product image, enabling marketers to quickly create many versions of an existing product image. For instance, a gray product could be shown with a brushed metal finish, providing customers a new visual without the associated cost and production time.
Machine learning algorithms are now able to isolate textures and materials, leading to precise editing abilities. Specific aspects, like scratches on wood or the weave pattern of a fabric, can be separately enhanced or changed, giving unprecedented levels of control and flexibility in how products are presented online.
Though machine learning has shown remarkable results in enhancing product textures, there is still a disconnect between images created with AI and real-world experiences. Customers might be disappointed if the texture they see online doesn't match what they receive. This could potentially lead to more returns.
7 Proven AI Techniques for Converting Traditional Economy Illustrations into Dynamic Product Visuals - Neural Networks Convert 2D Product Sketches into Realistic 3D Models
Neural networks are transforming the way we visualize products digitally by enabling the conversion of 2D sketches into realistic 3D models. AI-powered platforms are emerging, like Kaedim, that utilize machine learning to rapidly generate detailed 3D models from basic sketches, photos, or even technical drawings. This process significantly accelerates the design workflow and can help businesses bring new products to market faster.
Furthermore, techniques like NeRFs and depth estimation models allow users to interact with the resulting 3D models, exploring them from different perspectives and gaining a more comprehensive understanding of their features. This ability to easily generate interactive 3D product models can be especially valuable for showcasing products online, enhancing user engagement and potentially leading to higher conversions.
However, it's important to be mindful that these neural network-based systems rely heavily on the quality of the training data used. The accuracy and realism of the generated 3D models are directly related to the depth and diversity of the datasets employed during the AI's learning process. If the training data is limited or biased, the outcomes might not be entirely accurate, leading to a mismatch between the digital representation and the actual product.
Despite the potential limitations, the ability of neural networks to turn rough sketches into sophisticated 3D models represents a significant leap forward in product visualization for e-commerce. As this technology matures, we can expect to see more dynamic and interactive online shopping experiences, transforming how customers interact with and evaluate products before purchasing.
Neural networks are showing promise in transforming simple 2D product sketches into fully realized 3D models. This is quite remarkable, considering the complexity involved in translating a flat drawing into a three-dimensional object. These networks rely on sophisticated algorithms that dissect aspects like shape, perspective, and even the implied proportions within a sketch. It's fascinating how they can infer depth and dimensions from what's essentially a 2D representation.
The process typically involves advanced neural network architectures like Variational Autoencoders or 3D Generative Adversarial Networks. These models are trained using enormous datasets of 3D shapes and structures, allowing them to learn intricate patterns and relationships. The result is the ability to create 3D models that are remarkably accurate and realistically portray the intended design. A crucial aspect of this process is maintaining the physical characteristics of materials. The 3D model needs to capture elements like reflectivity and texture, not just for aesthetic reasons but for physical accuracy.
The training of these networks is deeply intertwined with computer vision principles. The algorithms learn to identify and recreate elements like shadows and lighting conditions, crucial for creating realistic product images. One interesting approach involves the creation of synthetic data to enrich the training process. By expanding the dataset with artificially generated examples, these models become more robust, better equipped to handle a wider array of product designs and variations.
The potential benefits are significant. Generating a 3D model from a 2D sketch can drastically cut down the time it takes to create marketable visuals. Traditionally, this was a highly manual process that required specialized skills and tools. Now, with AI, the process is automated, potentially saving companies a great deal of time and resources. This ability to rapidly translate ideas into 3D models also has implications for emerging technologies like virtual try-on and augmented reality. Customers could potentially view products within their own spaces, potentially boosting engagement and sales.
However, like any automated system, there are limitations. One challenge is the potential for mistakes. AI systems may misinterpret a sketch, leading to inaccurate proportions or features. This could have consequences, potentially impacting consumer trust and purchasing decisions. Moreover, the growing reliance on AI for product visualizations raises questions about authenticity. There's a growing discussion about the difference between real and AI-generated images, which can impact brand integrity and customer expectations.
While neural networks are clearly transforming product visualization, it's essential to understand their current capabilities and limitations. As these systems evolve, it will be important to develop strategies that maintain consumer trust while leveraging the incredible power of AI to create more engaging and realistic product experiences online.
7 Proven AI Techniques for Converting Traditional Economy Illustrations into Dynamic Product Visuals - Style Transfer Technology Updates Old Product Catalogs with Modern Aesthetics
Style transfer technology offers a powerful way to breathe new life into older product catalogs. Essentially, AI can take existing illustrations and apply a modern aesthetic, effectively transforming dated images into visually engaging visuals that resonate with modern shoppers. This process, often called Neural Style Transfer, lets brands update their product imagery without losing the original essence of the product. It's a way to inject new energy into old catalogs, enhancing their visual impact and aligning them with current brand identity trends.
Tools that allow for this type of style transfer, like Adaptive Instance Normalization, provide incredible flexibility in the process. They let you experiment and control how the style is applied in real-time, essentially opening up a whole new realm of creative expression with these images. Given the ever-increasing demand for captivating product visuals in the digital world, businesses are starting to see that adopting these kinds of AI techniques is crucial for staying competitive in today's e-commerce environment. While the technology is continually evolving, it's clear that the potential for style transfer in image updates is significant, with the ability to improve the visual quality and emotional connection with consumers.
AI-powered style transfer is transforming how we revitalize older product catalogs, breathing new life into traditional illustrations by applying modern design trends. This technology essentially allows us to take a classic product illustration and infuse it with the aesthetic elements of contemporary visual styles. It's like taking a vintage photograph and giving it a fresh, modern look without losing the original essence of the image.
This ability to automatically adapt product presentations to current design aesthetics is incredibly useful for brands looking to quickly refresh their catalog visuals. Imagine being able to easily switch between different styles – from minimalist to vibrant, or even incorporating trendy design elements from unrelated fields like architecture or fashion. This allows businesses to tailor their product presentations for different demographics or seasonal themes without the need for extensive manual redesigns.
Moreover, this technology seamlessly integrates with existing product data. We can effectively update traditional product catalogs without discarding the brand recognition built up over time. This is especially relevant for businesses with a long history, as it allows them to preserve brand identity while simultaneously adapting to evolving customer expectations.
The core mechanism behind style transfer often involves the use of techniques like Neural Style Transfer (NST), where the AI system essentially learns to separate content from style. It then uses this knowledge to apply a desired style to a base image. More advanced techniques like Adaptive Instance Normalization (AdaIN) and Artistic Universal Style Transfer via Feature Transforms (WCT) expand this capability, enabling the transfer of styles across a broader range of images and providing greater control over the creative process.
There's evidence suggesting that this style transfer approach can be highly beneficial for e-commerce. Studies have shown that products presented with updated visuals in line with current trends can significantly increase customer engagement, with some research suggesting an up to 20% jump in conversions. The visual appeal of these images, aligned with contemporary preferences, seems to play a key role in this increased engagement.
Beyond mere aesthetic improvements, style transfer can contextualize products within environments that resonate with target audiences. This means products can be shown in settings that enhance their appeal and build a narrative around their usage, a much more effective marketing strategy in the digital age.
Furthermore, consistently applying a unified style across different product images helps improve brand cohesion and recognition. This technique strengthens brand identity across the entire product catalog, leading to a more unified and memorable brand experience for customers, something that can foster loyalty.
The continuous evolution of these AI systems is leading to real-time adaptability. Businesses can now leverage current market data and customer preferences to instantly adapt their product visuals, ensuring a perpetually relevant online presence. This adaptability is crucial in today's fast-paced e-commerce environment, where trends shift rapidly.
The scalability of these systems is another advantage. Updating large product catalogs can be a significant undertaking. AI-driven style transfer can significantly reduce the time required for this process, enabling brands to react quickly to changes without relying on a large design team. This translates to reduced costs and more agile marketing efforts.
Finally, traditional methods of updating product aesthetics were often extremely resource-intensive, requiring significant manpower and time dedicated to photoshoots and intricate manual edits. Style transfer significantly streamlines this process, reducing these demands and leading to a greater efficiency in the creative workflows.
While the current capabilities of AI-powered style transfer are very promising, it's essential to remain mindful of the inherent limitations. For instance, the quality of the output is dependent on the quality and diversity of the training data. Additionally, the potential for the resulting images to appear artificial or fail to align with the desired aesthetic still needs to be carefully considered.
Nevertheless, the advancements we're seeing in style transfer technologies suggest that this trend will continue to grow in significance within e-commerce. It will be fascinating to see how this technology continues to evolve and shape the future of product visualization.
7 Proven AI Techniques for Converting Traditional Economy Illustrations into Dynamic Product Visuals - Advanced Image Upscaling Brings Low Resolution Product Photos to Life
Artificial intelligence is revolutionizing how we enhance product images, particularly those captured in low resolution. Advanced AI-powered image upscaling tools are now capable of taking blurry, pixelated images and transforming them into sharp, high-quality visuals. These techniques, driven by machine learning models trained on vast datasets of image pairs, can intelligently recognize and recreate missing details, effectively improving the resolution and visual quality of existing product photos.
Tools like Nero AI Image Upscaler and PromeAI's HD Upscaler are becoming increasingly popular among e-commerce businesses. They offer the ability to improve the clarity and richness of product photos, making them more appealing to online shoppers. However, it's important to acknowledge that while the technology is very capable, it's not without its limitations. Maintaining a natural appearance while upscaling can be tricky. There's also the potential for inconsistencies in output quality, which is something to keep in mind when applying these techniques.
Despite these challenges, AI upscaling holds significant promise for e-commerce. It empowers businesses of all sizes to enhance the visual appeal of their products, leading to a more positive customer experience. By creating higher quality images, these tools can help differentiate a brand's online presence in a crowded market, potentially boosting sales and customer satisfaction. As AI continues to improve, the ability to revive low-resolution images will become a crucial aspect of creating engaging and effective online product experiences.
AI-powered image upscaling has become quite interesting for improving e-commerce product visuals, particularly when starting with low-resolution source images. These advanced methods utilize deep learning approaches to essentially 'fill in the blanks' of missing detail, resulting in higher resolution images that don't suffer the usual blurriness or pixelation seen with more basic methods. It's like having a digital artist meticulously refine blurry edges, making the products look more appealing and detailed.
The training process behind these algorithms is also noteworthy. They learn to differentiate and recreate high-quality images by examining massive datasets of image pairs—one low-res and its corresponding high-res counterpart. This allows the AI to understand the nuances of how various elements in images relate and how to build those same features within the upscaled versions. It's similar to how a student might study a large collection of paintings to learn the masters' techniques, allowing them to apply what they've learned to their own work.
Furthermore, these newer upscaling techniques prioritize human perception over simple pixel-by-pixel accuracy. It's like judging a painting not just by its technical aspects, but by how it makes you feel and what it communicates. This focus helps the AI produce images that are more attractive and meaningful to customers, leading to more engaging online shopping experiences.
Many of these advanced upscalers utilize deep learning neural networks, specifically convolutional neural networks (CNNs). They operate in stages, analyzing and enhancing different aspects of the images, which gives a level of precision that simply wouldn't be possible with older methods. It's like a master craftsman who polishes and refines a piece of art step-by-step, each adjustment leading to a more perfected whole.
And the application of these AI-based tools is increasingly instantaneous. Many platforms can automatically upscale product images as they are uploaded, essentially eliminating manual labor for many companies and making the entire process more efficient. For companies trying to get products online as quickly as possible, or for smaller operations without a large team of dedicated editors, this capability can be a huge benefit.
Perhaps unsurprisingly, the quality of these upscaled images has a positive impact on customer satisfaction and, in turn, return rates. When online images provide an accurate representation of the actual product, buyers are less likely to be disappointed upon receiving their purchases. This can save businesses considerable costs in terms of handling returns and replacements. The data itself can even be used in feedback loops to fine-tune the algorithms over time, ensuring that the AI continually improves.
Additionally, there's a growing emphasis on creating a more natural and realistic appearance in the upscaled product images. For instance, techniques like texture synthesis can be used to create the look of detailed fabric, wood grain, or reflective surfaces. This extra level of visual appeal can be particularly important for selling certain product types where texture is a key aspect of the customer's perception.
This visual enhancement can be valuable in making products work better with augmented reality applications as well. AR applications need high-resolution images to work properly, and these upscaling tools can play an important role in ensuring that online visuals have the quality necessary to provide a convincing experience for potential buyers.
And ultimately, a greater focus on high-quality visuals in e-commerce has a positive influence on a company's ability to attract a wider range of customers. In the increasingly competitive world of online shopping, high-quality product images, especially when compared to lower-quality or less compelling competitors, stand out.
All of these capabilities highlight the extent to which image upscaling has become a powerful tool for modern e-commerce. It's no longer just about improving visual aesthetics but also about contributing to customer satisfaction, reducing costs associated with returns, and enhancing marketing and sales potential. The continuous development of these AI-based techniques promises a future where online product presentation is both visually appealing and commercially advantageous. However, as with most powerful technologies, it's crucial to monitor how these tools are deployed, to avoid unintended consequences or misleading customers.
7 Proven AI Techniques for Converting Traditional Economy Illustrations into Dynamic Product Visuals - Deep Learning Systems Create Multiple Product Angles from Single Photos
Deep learning technologies are increasingly vital for e-commerce, specifically in the ability to generate a variety of product angles from just one photograph. This allows shoppers to get a more complete picture of the product, which in turn leads to more interactive and informative online shopping experiences. AI systems can use neural networks to create multiple product images that show different viewpoints, making it feel like customers can interact with the product in person. The benefit to online stores is a way to engage customers with more realistic visual information.
However, it is important to be aware that relying on AI-generated imagery raises a few questions. The degree of authenticity is always a factor with AI images. The systems are also highly dependent on the quality of their training data. If the data is flawed or inadequate, it can lead to less-than-ideal results, which can hurt the reputation of the company. E-commerce businesses will need to carefully consider how to adopt these deep learning systems while balancing innovation with the need to maintain consumer trust. It will be important to track how this technology develops and ensure that it is used responsibly.
Deep learning systems are increasingly capable of generating multiple product views from a single photograph, a technique that has the potential to transform how we present products online, especially in e-commerce. These systems, often employing techniques like Generative Adversarial Networks (GANs), can essentially create a virtual 3D model of a product from a 2D image, allowing businesses to generate a range of perspectives without the need for additional photography sessions. This capability can streamline the creation of marketing materials and make product presentations more comprehensive.
One of the primary benefits of this approach is its ability to enhance the online shopping experience. When customers can see a product from multiple angles, they can gain a better understanding of its design, features, and dimensions, reducing the uncertainty that can often lead to returns. Furthermore, these AI-driven systems can dynamically adapt the generated views based on user behavior and preferences, personalizing the shopping experience and potentially leading to increased engagement and sales conversions. For example, the system could showcase a different angle of a shoe based on whether the user is mainly interested in the heel design or the toe cap.
It's intriguing how these models can sometimes produce a variety of viewpoints from just a handful of initial photos, sometimes even just one or two. This reduces the need for extensive photoshoots and significantly reduces the costs associated with creating comprehensive product visuals. We are seeing advancements in real-time rendering where users can manipulate the views of products instantly, creating a dynamic, interactive shopping environment. This type of interactivity is comparable to handling a physical product in-store, potentially bridging the gap between the online and offline shopping experiences.
The quality and consistency of product visuals are essential for establishing brand identity and consumer trust. AI-generated images created from multiple angles using deep learning offer the possibility of maintaining a consistent brand aesthetic across all product presentations, enhancing the customer's sense of continuity and familiarity with a brand's visual identity.
Beyond just generating different angles, these systems can also seamlessly place products within a variety of realistic scenes. This ability to contextualize a product eliminates the need for separate lifestyle photoshoots, significantly reducing costs and production time. Furthermore, this allows businesses to quickly generate variations for a product's look and feel without physical prototypes, streamlining product design cycles and allowing for more innovative product development.
However, there's a developing ethical and legal landscape surrounding these new image generation capabilities. As AI-generated images become more prevalent in e-commerce, questions arise regarding copyright and intellectual property. The ownership of an image generated by an AI based on a single source photo is unclear and might be contested. E-commerce businesses may encounter legal challenges in this area as the use of AI-generated images becomes more common.
It's still early days for this technology, but it's evident that it has the potential to profoundly change how we present and interact with products online. As the technology evolves, we can expect to see more dynamic and interactive shopping experiences, blurring the lines between traditional product photography and AI-powered visual generation.
More Posts from lionvaplus.com: