Create photorealistic images of your products in any environment without expensive photo shoots! (Get started for free)
7 Essential AI Image Generation Techniques for Creating Realistic Adventure Product Mockups
7 Essential AI Image Generation Techniques for Creating Realistic Adventure Product Mockups - Mastering Midjourney Style Transfer for Adventure Product Backgrounds
Midjourney's style transfer feature is a powerful tool for creating visually appealing backgrounds for adventure products. It allows you to maintain a consistent brand look and feel by incorporating your own design elements into the generated images. The ability to use reference images helps Midjourney understand the desired context, ensuring the generated backgrounds are relevant to the product. Tools like Inpainting and Outpainting give you granular control over the final image, allowing you to refine details and adjust the canvas size.
Crafting the right prompts is vital to getting the results you want. Experimentation with prompt engineering and understanding the different parameters available within Midjourney are key to mastering this process. You can iterate through various versions using rerolls and variations, finding the perfect balance of style and visual appeal. Midjourney delivers high-quality images, making it a strong contender for creating realistic and engaging mockups that capture the essence of an adventure product. While accessible through Discord and a new web interface, becoming proficient with this platform requires practice. The future of ecommerce product visuals is likely to be heavily influenced by AI tools like Midjourney, so learning to leverage these capabilities is becoming increasingly important for brands.
Midjourney's strength lies in its ability to adapt and blend artistic styles into product backgrounds. It's not just about creating images; it's about controlling the visual language used to represent a product within a specific scene. This capability is particularly valuable for adventure product imagery where maintaining a consistent brand identity across diverse settings is essential.
Users can feed reference images into Midjourney, influencing the output and ensuring that the AI-generated background aligns with a specific aesthetic. Furthermore, tools like Inpainting and Outpainting offer the ability to make precise alterations, allowing for more control over the final composition. This is quite powerful for refining the aesthetic and integrating product mockups smoothly.
Prompt engineering, the art of crafting effective instructions for the AI, becomes a key factor in producing desired results. Midjourney allows for exploration and experimentation with prompts, offering a ‘reroll’ option to generate multiple versions of the same concept. The platform also includes a range of parameters, essentially code-like commands that influence visual elements and help fine-tune the AI’s output. It's like having a set of controls to dial in the exact look and feel.
Interestingly, though the primary interface uses Discord, there's a web interface in development. While currently in a limited alpha testing phase, it indicates a future where accessing Midjourney's tools may become more streamlined. The journey of mastering Midjourney, though, requires consistent practice. It's about experimenting with different prompts, understanding how parameter changes impact the final image, and developing an intuitive grasp of how Midjourney interprets artistic styles.
Looking at the bigger picture, the broader AI art field is rapidly developing, and Midjourney seems to be at the forefront of tools offering realistic results for e-commerce applications. This highlights a shift in how visual content can be generated for product marketing and representation, with tools like Midjourney becoming crucial components of an effective visual strategy.
7 Essential AI Image Generation Techniques for Creating Realistic Adventure Product Mockups - Engineering Lighting Effects in AI Generated Product Mockups
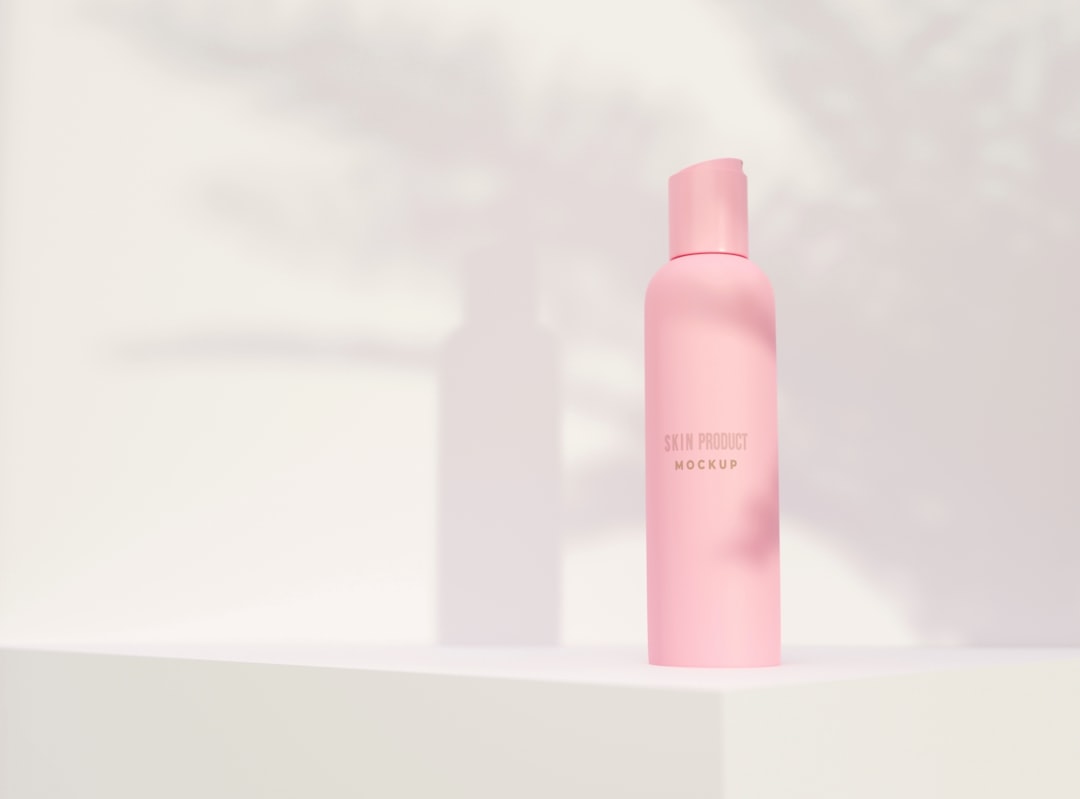
**Engineering Lighting Effects in AI Generated Product Mockups**
When using AI to generate product mockups, effectively controlling lighting is crucial for producing impactful visuals. Lighting isn't just about making products look good – it's about crafting a mood and telling a story through the image. By skillfully adjusting light sources and the resulting shadows, designers can evoke specific feelings in viewers, showcase the textures and features of a product, and guide attention where it's most desired. The continuous advancement of AI tools provides new opportunities to fine-tune these lighting effects, allowing brands to craft unique visual experiences for their products and establish a strong brand identity. It's worth remembering that while AI makes design simpler, a thorough grasp of lighting principles remains critical to creating truly realistic and captivating mockups. Without this understanding, AI-generated images can sometimes fall short of achieving the desired level of visual appeal and realism.
AI-generated product mockups are changing how we visualize products, allowing for customization and unique visuals that weren't previously possible. It's fascinating how these tools can impact entire industries and how businesses market themselves. When it comes to AI-generated imagery, lighting is crucial in setting the tone and telling a story. It's become a key design element.
These tools allow us to explore different design options quickly through text prompts. If you're specific and detailed in your descriptions, the AI can generate images with accurate poses, expressions, lighting, and backgrounds. There are numerous AI mockup generators, each with its own features and pricing. It's interesting that there are choices to fit a wide range of products and needs.
Beyond visual appeal, lighting is a powerful way to control the atmosphere of a generated image. This could be a dramatic, almost moody, feel or a bright and vibrant style. AI-powered design tools can also adapt to established brand guidelines, ensuring consistency in product visuals, which is really helpful in maintaining a strong brand identity.
The process of using these tools is usually straightforward—pick a product type, describe your design, and generate your mockup in a few steps. There is, however, a question of how we use AI responsibly. We should think about the sustainability and ethical implications of AI-generated images.
Lighting direction can impact how we perceive depth and texture in product mockups. Lighting from the top can emphasize the height of a product, while side lighting helps with seeing three-dimensionality. The color temperature—cool or warm—influences our perception of a product. Cooler tones often give a sense of cleanliness, suitable for electronics perhaps, while warmer tones evoke feelings of comfort and relaxation, maybe more appropriate for home goods.
The way a surface is depicted as reflecting light is key to how we understand the materials. A high gloss look can imply luxury, while a matte finish suggests something simpler. The accurate creation of shadows can contribute to a mockup’s realism and help it feel like it belongs in a real environment. Shadows indicate where light is and help to ground the product.
Smart use of highlights can guide a viewer’s eye to particular parts of a product. Lighting helps with visual hierarchy. Ambient lighting is also very impactful in e-commerce photos—low light can give a dramatic effect, while a bright environment communicates energy.
AI tools are developing to the point where they can simulate how light bounces off surfaces—something called global illumination. This makes the mockups more like how we experience things in reality. We're at a point where we can tweak brightness and contrast in real-time. This gives designers a dynamic way to refine mockups.
From a psychology perspective, how we react to different light environments influences how we feel about products. So, understanding the emotional connection that can be formed through lighting is a key element to consider. AI tools are now equipped to handle lighting setups that used to require extensive work after image creation. The future of e-commerce visuals appears to be closely intertwined with AI tools. They offer significant time and resource savings, which will enable the creation of even more high-quality images for online marketplaces.
7 Essential AI Image Generation Techniques for Creating Realistic Adventure Product Mockups - Creating Depth and Shadow Maps for Realistic Product Placement
Generating realistic product placements in e-commerce imagery relies heavily on creating depth and shadow maps. These maps essentially simulate how light interacts with objects, giving products a three-dimensional feel that draws the viewer in. Shadows play a crucial role in anchoring products within their environment, adding a sense of depth and realism that enhances the overall impression. Without effectively incorporating shadows and depth cues, AI-generated product images can fall short of expectations, potentially diminishing the perceived quality and desirability of the products themselves. The ability to create realistic lighting effects through depth and shadow maps is becoming increasingly important as AI tools continue to advance, allowing brands to produce more engaging and persuasive product mockups. It's clear that for e-commerce visuals to be convincing, understanding how to manipulate light and shadow using these techniques is a critical skill moving forward.
Generating realistic product placements within AI-created images relies heavily on depth and shadow maps. These tools offer a fascinating way to imbue our digital scenes with the same depth cues that our eyes naturally use to understand the 3D world. Let's explore some key ideas.
Firstly, our perception of depth is largely built on things like shadow and perspective. AI image generators can create a stronger sense of realism by mimicking these visual cues, essentially tricking our brains into believing a flat image has volume. This, in turn, might lead to a higher degree of trust from potential buyers.
Secondly, techniques like shadow mapping, which uses textures to store depth information, are used in computer graphics to create more believable shadows. Instead of having flat, unrealistic shadows, we can create softer, more nuanced ones that adapt to the shape of an object and the environment. This significantly improves the quality of the generated image.
Interestingly, the depth and shadow maps don't have to be static. They can dynamically react to changes in the light sources in the scene. This means we can showcase the same product under different lighting conditions without re-rendering the whole image each time. It's a significant time-saver for those creating e-commerce visuals.
The way shadows are rendered can impact how we perceive products. Studies have shown that deeper shadows might make a product seem bigger or more substantial. This is a subtle psychological effect that can influence buying decisions. The interplay between surface materials and shadow depth is important, too. Glossy surfaces reflect light differently than matte ones, affecting how the product's texture is perceived.
Beyond making things look better, depth maps also provide a way to guide the viewer's eye. By carefully adjusting depth, we can steer attention to specific features of a product, a crucial aspect of online shopping where capturing attention is paramount.
Currently, we're seeing more AI tools capable of real-time shadow and depth rendering, which speeds up the design process significantly. Designers can iterate quickly and adjust images dynamically, making the process of creating compelling product images far more efficient.
The emotional impact of shadows is also a fascinating aspect. It's believed that softer shadows can create a feeling of warmth and comfort, while harsher ones can evoke a sense of drama or urgency. These subtle emotional cues can affect how consumers react to a product.
This new technology can drastically reduce the cost associated with traditional product photography, making high-quality images accessible to a wider range of businesses. It also opens the door to innovative applications like augmented reality shopping. Imagine seeing a product realistically placed in your own home before buying it. Accurate depth and shadow information will be critical to creating that sort of immersive experience.
As we continue to refine depth and shadow mapping in AI image generation, we're likely to see even more sophisticated e-commerce visuals in the future. This area of research seems to hold a lot of potential for shaping how we interact with and experience products online.
7 Essential AI Image Generation Techniques for Creating Realistic Adventure Product Mockups - Using ControlNet Techniques for Accurate Product Perspectives
ControlNet techniques provide a way to create more realistic and accurate product perspectives in AI-generated images. They help ensure products look believable in different settings by precisely managing how depth, lighting, and shadows work together. Essentially, these techniques enhance the AI's understanding of spatial relationships, which is crucial for avoiding the common issue of unrealistic-looking product placements in generated images. This is especially important for online businesses since the quality of a product image often affects whether or not a customer decides to buy it. As these AI image generation tools continue to improve, understanding and using ControlNet techniques could become more vital for businesses aiming to create convincing and trustworthy product presentations online. While the technology still has limitations, ControlNet shows promise for tackling some of the challenges currently faced in creating realistic ecommerce product images.
ControlNet's contribution to accurate product perspectives within AI-generated images is intriguing. It's essentially about giving us finer control over how the AI understands and renders depth and spatial relationships in a scene. This can be quite useful in ecommerce because we want shoppers to see products in a way that feels realistic and trustworthy. This ties in with the way we, as humans, instinctively process visual information. We rely on things like shadow and perspective to understand if something is near or far, big or small. When an AI image accurately represents those cues, it creates a more convincing and believable visual experience.
One of the more fascinating aspects is that AI tools are starting to be able to dynamically adjust shadows and depth in response to lighting changes. Instead of having to re-render a whole scene every time we change a light source, we can simply update the shadow map. This means that, in theory, we can create diverse looks for the same product using a wider range of light conditions – from warm, inviting lighting for home goods to the clean and bright aesthetic common for tech products – without a major time investment.
Beyond just aesthetics, this ability to adjust material properties and shadow depth is starting to unlock new avenues in the way we understand products. The way light interacts with a surface – be it glossy or matte, rough or smooth – influences how we perceive a product's texture and quality. A high-gloss finish can look luxurious because of the way light reflects off of it, producing crisp highlights and sharp shadows. In contrast, a matte finish, which diffuses light more, might convey a sense of casualness or practicality. These subtle visual cues, guided by the precise control afforded by techniques like ControlNet, have the potential to have a real influence on the overall perception of a product.
There's also a compelling psychological component at play here. Interestingly, studies have shown that shadows can alter our sense of a product's size. Deeper shadows, for example, can sometimes make a product appear bigger or more substantial. This isn't something we consciously realize, but it can impact how attractive a product feels to us. The same principle can be used to guide our attention to specific details. By using shadow to create visual cues, we can direct a viewer's eye to the most important parts of a product, increasing the chances that they'll notice the details that we think are most impactful.
The advancements in AI tools are starting to allow for real-time interaction with these shadow and depth parameters. This is quite powerful. It means that designers can tweak and fine-tune images rapidly, allowing for much quicker iteration cycles. This is a huge improvement for e-commerce, where quickly creating compelling product images is so important to a business’s success. It's also important to consider the broader implications. As this technology becomes more sophisticated, there will be implications for AR shopping experiences. If we can generate realistically rendered products within a real-world environment, we'll get much closer to creating immersive retail experiences that blend the physical and digital in new ways. That capability relies heavily on shadow and depth maps functioning accurately, to make things appear authentic.
In essence, understanding these techniques becomes key for brands seeking to produce the most persuasive product imagery in the ecommerce space. It’s not just about creating a pretty picture; it's about using tools like ControlNet to engineer more meaningful visual connections with products. It's about influencing how consumers perceive and react to those products. And it's about exploring new avenues in interactive experiences, all underpinned by the ever-improving ability of AI to represent visual information in a way that aligns with our innate ways of understanding the world.
7 Essential AI Image Generation Techniques for Creating Realistic Adventure Product Mockups - Implementing Image Inpainting for Seamless Product Integration
Image inpainting offers a powerful way to seamlessly integrate products into existing visuals, especially within e-commerce contexts. It essentially uses artificial intelligence, specifically deep learning algorithms, to intelligently fill in or remove parts of an image. This is helpful for ensuring a cleaner, more refined look for product placements, eliminating unwanted elements or seamlessly incorporating products into diverse backgrounds.
The use of generative AI approaches alongside inpainting opens up new possibilities for marketing and product presentation. Instead of clunky or unnatural-looking product insertions, businesses can leverage inpainting to integrate products into diverse scenes and contexts more realistically, making the shopping experience more engaging. Techniques like BrushNet, which utilizes a dual-branch diffusion process, offer more control over the inpainting process, leading to higher-quality results.
The ability to easily manipulate image elements with inpainting is particularly relevant for e-commerce. Businesses can create consistency in their marketing materials by using inpainting to refine visuals and maintain a particular style across various platforms and channels. In an era where consumers are inundated with visual content, the ability to create immersive and convincing product images using AI-powered tools like inpainting has become vital. As e-commerce continues to evolve, the significance of inpainting for crafting compelling product visuals that connect with shoppers will likely increase.
Image inpainting, a technique within computer vision, uses machine learning to intelligently fill in missing or damaged parts of an image. It's like having a digital artist that can seamlessly patch up a photo, making it look complete and natural. These AI models learn complex patterns and textures directly from pixel data, enabling them to accurately recreate missing details, whether it's a product being added to a scene or repairing a damaged area of the image.
One popular approach to inpainting is the BrushNet model, which employs a clever dual-branch diffusion method. This allows for a more flexible and modular approach, making it easier to adapt to different inpainting tasks. The ability to integrate products into existing images smoothly through AI opens up possibilities for richer and more impactful marketing materials.
While traditionally image editing tools were often limited, the flexibility of Python for AI image generation is notable. It gives developers the tools to customize the algorithms and outputs, allowing for a wide range of applications. Interestingly, these techniques aren't restricted to still images. They're also finding their way into video processing, potentially revolutionizing how we create and edit video content.
Furthermore, the use of contextual attention within inpainting models is a game-changer. The AI doesn't just focus on the immediate surroundings of the missing area; it can also look at more distant parts of the image for clues. This broader awareness helps it generate more accurate and realistic fills. Another intriguing aspect is the role of the WGAN (Wasserstein Generative Adversarial Network) loss function. This helps improve the quality of generated images, making them look even more realistic and natural.
The range of applications for inpainting techniques is also noteworthy. It's not just for e-commerce product images, they can be leveraged in diverse fields like game development to create more immersive virtual worlds. In essence, it allows for the creation of alternate visuals or entirely new images from existing ones. In a sense, it's like having a digital creative tool that can seamlessly integrate or enhance content in a way that previously was labor-intensive and technically challenging. The potential for inpainting to reshape how digital content is generated and presented is exciting, though it's also crucial to consider the potential biases and ethical implications of these technologies, particularly in e-commerce scenarios where trust and product representation are crucial factors.
7 Essential AI Image Generation Techniques for Creating Realistic Adventure Product Mockups - Training Custom Models for Brand Specific Product Details
Training custom AI models for brand-specific product details is becoming increasingly crucial for e-commerce. By feeding AI models datasets of images carefully labeled with information unique to a brand, we can guide the AI to generate visuals that truly reflect the brand's identity. This involves not just the products themselves, but also the overall aesthetic and look of how the products are presented. This process can be significantly improved by using short, varied descriptions (captions) that describe the kind of images you want. This helps the AI learn to better understand what makes your brand unique and allows for more tailored results. As the field of AI image generation advances, using techniques like GANs and diffusion models will become more important for creating highly realistic images of products. This has the potential to reshape how brands market themselves, offering new and more persuasive ways to showcase their goods. The future of visual branding within e-commerce likely rests on mastering these custom AI training methods to stand out in a marketplace saturated with visuals.
Training custom AI models to generate product images tailored to a specific brand is a really interesting area of research within ecommerce. It's essentially teaching an AI system to understand the unique visual style and elements of a particular brand.
One of the initial observations is that **the quality of the training data is critical**. If you only train a model on a few pictures from a single brand, it might not be very flexible. Ideally, you want to give it a diverse range of images, showing different lighting, product angles, and contexts. Otherwise, the model might not be able to create convincing mockups that are distinct to the brand but also generalizable enough for various product lines.
There's also the concept of **maintaining brand consistency**. Companies want their product images to look cohesive, and AI is a promising way to achieve that. By training a model on a brand's visual language, it can learn to recreate those design elements. This is quite valuable in a field like ecommerce, where maintaining a recognizable visual identity across all your products can significantly improve brand recognition. It's interesting to think about how AI could help automate visual branding across a large catalog of products.
Interestingly, **using high-resolution images during training seems to make a significant difference in the quality of the output**. It allows the model to learn intricate details, textures, and features which leads to images that look much more realistic. This idea of image resolution has interesting implications for how AI models are trained, particularly in domains where product details and accuracy are crucial.
One exciting idea is **feeding consumer data into the training process**. This includes things like customer preferences, purchasing behavior, and click-through rates. With this type of data, AI models can become increasingly adaptive, learning what types of imagery resonate most with certain consumer segments. It could eventually lead to AI systems that can optimize images dynamically in response to what shoppers are looking at and purchasing.
Going a step further, **real-time feedback** mechanisms could give AI models the ability to adjust their output immediately based on user interactions. Think of it like an AI image generator that continually learns what works best for a specific customer. This kind of dynamic feedback loop could improve the quality of the images over time and help to personalize online shopping experiences.
Beyond visuals, there's also the idea of **teaching AI models the 'meaning' behind product imagery**. This means not just focusing on the colors and shapes, but also training them to understand product features, benefits, and how people typically use a product. For example, if you're selling camping gear, the model could learn to generate images that showcase the practicality and durability of products in realistic outdoor environments. That level of semantic understanding could improve image quality in ecommerce.
Training models to be sensitive to **light and shadow** is also important. It helps AI systems to create a more three-dimensional look and feel in product images. The interplay of light and shadow can actually have subtle psychological effects on the way customers perceive a product. For example, certain lighting might make a product seem more substantial or luxurious.
Another trend is the growing **integration of AI models with augmented reality**. This is becoming increasingly important for online shopping, as customers want to be able to see how products look in their own homes or environments. A trained AI model that is sensitive to the nuances of shadow and depth would play a crucial role in creating this immersive AR experience.
Furthermore, **AI models are being developed to be more resilient to errors**. The learning process involves refining the model's ability to correct mistakes, improving the quality of the images over time. This feedback loop is essential in building more effective AI systems.
Lastly, it's interesting that researchers are exploring **multifaceted training objectives** which involve balancing factors like aesthetic quality, brand consistency, and image accuracy. This approach moves away from just prioritizing the technical quality of image generation and considers more human-centered aspects. This holistic approach could pave the way for truly effective custom models in ecommerce that can meet the multifaceted needs of both businesses and shoppers.
It's clear that the ability to create custom AI models for product details in ecommerce is a fast-evolving area. These models have the potential to reshape how online shoppers perceive products and influence purchasing decisions. As these technologies develop, it will be interesting to see how AI evolves and adapts to the changing landscape of online retail.
7 Essential AI Image Generation Techniques for Creating Realistic Adventure Product Mockups - Optimizing Stable Diffusion Parameters for Material Textures
When crafting realistic product visuals using AI, especially for online stores, understanding how to adjust Stable Diffusion's settings to control material textures becomes very important. The Classifier-Free Guidance (CFG) scale, often used in the 7-15 range, plays a crucial role in managing image detail. While aiming for a lot of detail can be tempting, using a CFG of 16 or higher is typically not a good idea as it can negatively affect how cohesive and clear the image looks. Fortunately, Stable Diffusion can generate highly detailed and realistic textures of a wide variety, such as stone covered with moss or intricate woven baskets, which benefits ecommerce product presentations. It's noteworthy that you can influence the resulting visuals by adjusting the image resolution and incorporating artistic styles or elements into your prompts. The creative possibilities expand when combining these aspects, allowing designers to shape product imagery to effectively represent a brand's visual language. For brands hoping to improve their product presentations in the highly visual world of online shopping, learning how to control these parameters is no longer just a matter of technical proficiency, but a key part of creating engaging and impactful visuals.
When it comes to crafting realistic product visuals for e-commerce, Stable Diffusion's potential to generate convincing material textures is quite exciting. However, it's not just about slapping a prompt into the system and expecting perfect results. There's a hidden layer of control that can significantly elevate the quality of the output: parameter optimization. Let's dive into some fascinating observations about how manipulating Stable Diffusion's settings can be used to improve the quality of material textures.
One of the first things that's become clear is that the level of detail in generated textures is closely tied to parameters like noise level and how many sampling steps the AI takes. By tweaking these settings, you can significantly refine the appearance of materials like fabric and glass, bringing them closer to the look of real-world counterparts. It's not a one-size-fits-all solution, though. Different materials, we've discovered, seem to respond better to certain parameter combinations. For example, if you're aiming for a realistic metallic sheen, you might need to crank up the sampling steps to capture those reflective qualities properly. Softer materials, like cotton, might not require as much tweaking.
Furthermore, it's become apparent that you can scale parameters adaptively depending on the complexity of the texture you're trying to achieve. Imagine you're trying to replicate a very intricate woven fabric. In those cases, fine-tuning settings like learning rates is crucial to making sure the AI captures all the tiny details without introducing strange visual artifacts. There's a fine balance to be struck here, and it's definitely an area that needs more experimentation.
It's also intriguing to consider the potential of higher-dimensional parameter arrays. Currently, we're mostly dealing with 2D textures. However, it's possible to encode additional information into the system. Imagine including things like the reflectivity or light absorption properties of a material directly into the parameters. This could open up a whole new level of detail, allowing for the creation of truly hyperrealistic textiles that mimic the way light interacts with real-world surfaces.
Another important aspect of optimization involves addressing potential issues like interference artifacts. These glitches can show up in complex materials, particularly woven ones, where visual inconsistencies can be noticeable. Thankfully, you can often mitigate these issues by carefully adjusting Stable Diffusion's parameters.
A promising technique involves a form of transfer learning, where you take a model that's been trained on a large dataset of different textures and then fine-tune it on a specific material, like wood or leather. This approach seems to result in a significant improvement in texture quality and visual coherence, making it easier to achieve consistently realistic results.
Another fascinating aspect is the potential for incorporating spectral data into the process, particularly for materials like glass. By considering how different wavelengths of light interact with the material, we can get a better representation of how glass would look in various lighting conditions. This could potentially provide a richer, more realistic representation of transparent materials in rendered scenes.
From a practical perspective, automating some of the parameter optimization would be immensely helpful, particularly for businesses with a wide range of products that need consistent material representation. Scripts could be written that streamline the process, reducing the manual work involved and ensuring a consistent brand aesthetic.
Interestingly, implementing techniques like cyclic redundancy checks during the generation process might be a way to detect inconsistencies in texture application across a series of images, further enhancing quality control. It's a subtle but potentially very valuable tool.
Perhaps most intriguingly, we're starting to see the potential to connect user behavior with parameter settings. By analyzing how people interact with images, and by observing engagement metrics, we might be able to glean insights into which parameter combinations are most effective for specific products. This dynamic, data-driven approach could be a powerful way to ensure that AI-generated product visuals are as engaging as possible for customers.
These insights, though preliminary, paint a compelling picture of how the development of Stable Diffusion could be leveraged for ecommerce. There's a lot of potential here to craft a truly immersive online shopping experience through the creation of convincing product imagery, but it will require a much deeper understanding of how these parameters influence the AI's creative process. This is a field that's ripe for research, and these first insights only suggest the possibilities that might lie ahead.
Create photorealistic images of your products in any environment without expensive photo shoots! (Get started for free)
More Posts from lionvaplus.com: