Create photorealistic images of your products in any environment without expensive photo shoots! (Get started for free)
SWAV's Impact on AI-Driven Product Image Generation A Technical Deep Dive
SWAV's Impact on AI-Driven Product Image Generation A Technical Deep Dive - SWAV's neural network approach to unsupervised learning in image generation
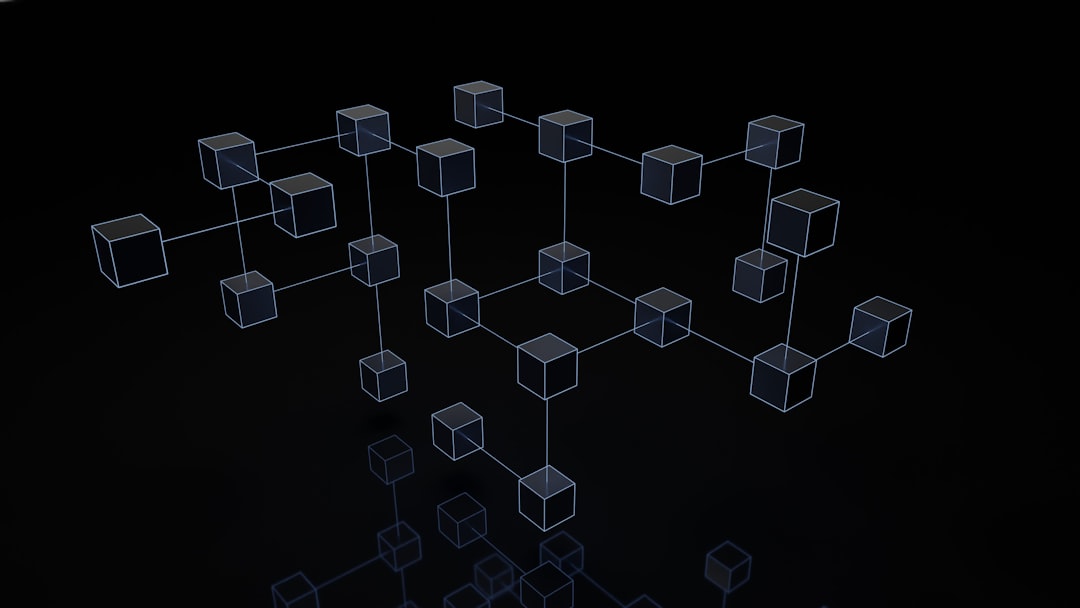
SWAV's approach to image generation tackles the challenge of unsupervised learning with a neural network that bypasses traditional clustering methods. This allows for a completely deep learning-based process, eliminating the need for separate feature learning and clustering steps. Instead, SWAV leverages self-supervised learning, extracting information directly from the data itself without requiring pre-labeled examples. This self-supervision allows the network to discover intricate relationships within the image data, effectively learning to represent visual information without the need for human intervention. While still a relatively new approach, SWAV has demonstrated promising results in closing the performance gap compared to traditional supervised learning techniques. This advancement holds significant potential for AI-driven product image generation, where the ability to efficiently learn from unlabeled data could lead to more realistic and appealing imagery.
SWAV is an interesting approach to unsupervised learning that sidesteps the traditional methods like K-means. This means it operates on a purely deep learning approach, which is exciting for image generation. The focus is on unsupervised image classification, which means it doesn't need labeled data. This is particularly interesting for e-commerce, where the sheer volume of images can be overwhelming for manual labeling.
SWAV's contrastive learning methodology is quite impressive, especially how it learns by comparing different views of the same product. This approach allows for higher-quality product images, which can be very useful for capturing consumer interest. The ability to learn from unlabeled data is a huge advantage, making it more efficient than traditional supervised models. I'm particularly intrigued by its potential to create new product representations, like style variations or seasonal adaptations, which could really help keep product catalogs fresh and trendy. This technology could be a game-changer for the e-commerce landscape, especially as we see the growing importance of visually engaging content. However, it's important to consider that while SWAV is quite powerful, there are still challenges in accurately capturing nuances of product detail and handling complex product variations.
SWAV's Impact on AI-Driven Product Image Generation A Technical Deep Dive - Deep learning advancements in AI-driven product visualization
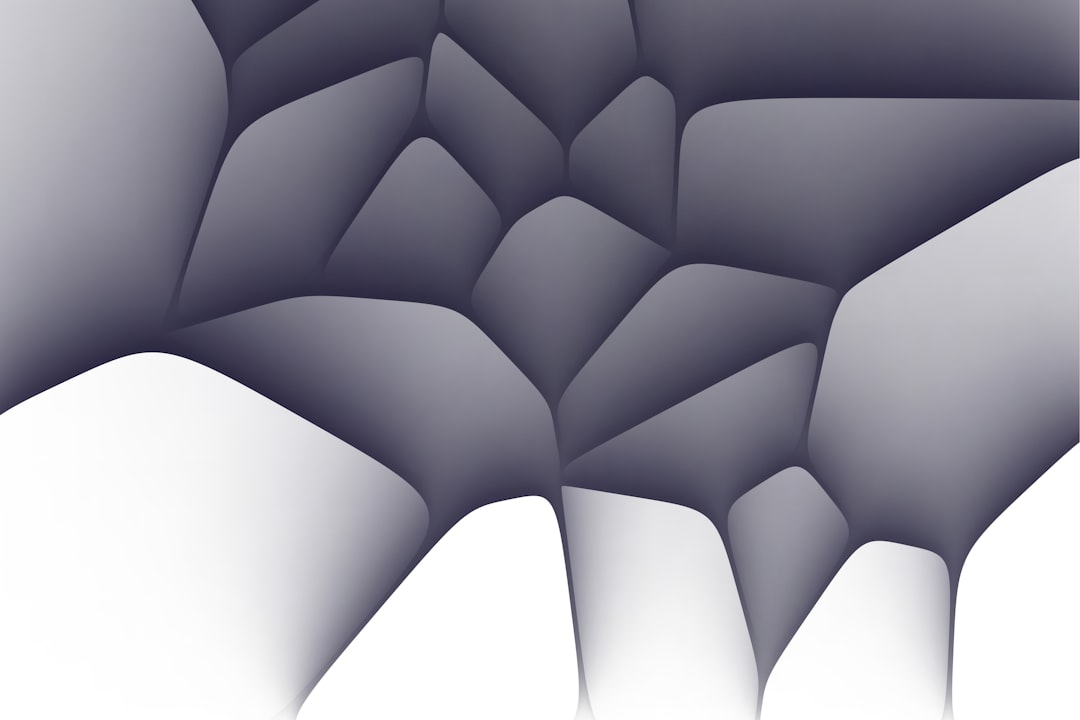
Deep learning has revolutionized how products are visualized in e-commerce, particularly through AI-powered image generation. Gone are the days of relying solely on traditional methods; deep learning offers much greater control and flexibility, leading to more personalized and engaging experiences for customers. Generative AI models are now capable of creating incredibly realistic product visuals, even simulating the experience of trying on clothes. The possibilities are exciting as algorithms like SWAV continue to evolve, potentially changing the very way we shop online. However, there's still work to be done. Deep learning models need to become even more adept at handling the intricate details and subtle variations inherent in many products. It's a constant challenge to capture the essence of a product and translate it into an image that truly captivates potential buyers.
SWAV's approach to image generation is a really interesting concept that departs from traditional methods in some key ways. Instead of separating feature learning and clustering, it combines these into one unified system. This means that information is preserved throughout the entire process, avoiding potential inefficiencies that could occur when these steps are handled separately.
Furthermore, SWAV's approach to image generation is quite memory-efficient, making it more accessible for smaller e-commerce players who might not have the same computational resources as larger corporations. This is quite significant, as it could potentially democratize the use of AI-driven product image generation within the e-commerce landscape.
However, the ability to adapt to different dimensionality is where SWAV truly shines. It can learn to represent products with varying degrees of complexity, something that has historically been challenging for standard algorithms. Imagine being able to generate images of complex textile patterns or intricate multi-part electronic devices – that's the kind of capability that SWAV offers.
This adaptability is also reflected in its ability to generate a wide variety of product images from a relatively small dataset. This is particularly helpful for e-commerce businesses where having multiple representations of the same product can greatly increase customer engagement and potentially drive more sales. Moreover, SWAV can learn in real-time, meaning that it can quickly adapt to new products without needing extensive retraining. This real-time adaptation is essential in the fast-paced world of e-commerce.
Beyond simply generating visually appealing images, SWAV can also develop a semantic understanding of products. This means that it can learn the relationships between different products based on their visual features. This capability could lead to the generation of images that show accessories that are relevant to a particular main product, offering opportunities for cross-selling.
One of the most exciting aspects of SWAV is its ability to handle complex product scenes. This stands in contrast to traditional algorithms, which often struggle with cluttered or multi-object scenarios. SWAV, on the other hand, can understand and generate realistic representations of complex product arrangements, providing a more accurate view of how items would look in a real-world setting. This ability is quite valuable for presenting products in a way that is both visually appealing and true to life.
It's also worth noting that SWAV's ability to generate augmented data samples is a huge advantage. This could result in datasets that are more diverse, including images that capture different angles, lighting conditions, and backgrounds. A more diverse dataset can help improve the generalization and robustness of models, making them less susceptible to variations in real-world conditions.
SWAV's capability to handle significant intra-class variability is also significant, particularly for sectors like fashion and lifestyle, where products can vary widely in terms of patterns, colors, and other characteristics. This reduces the need for exhaustive labeling, making the whole process more efficient. Finally, SWAV's output can be readily integrated with AR applications, further enhancing the online shopping experience. This allows consumers to interact with generated images in a virtual environment, bridging the gap between online and physical retail experiences.
Despite all of these exciting advancements, it's important to acknowledge that SWAV still has challenges to overcome. For example, accurately capturing fine product details and handling complex product variations requires further development.
In conclusion, SWAV's potential in AI-driven product image generation is quite evident, especially considering its unique combination of strengths: its ability to learn from unlabeled data, generate a wide range of product images, adapt in real-time, and handle complex product scenes. This combination makes it a truly exciting technology, offering a glimpse into a future where AI seamlessly enhances e-commerce experiences.
SWAV's Impact on AI-Driven Product Image Generation A Technical Deep Dive - Enhancing product image quality through SWAV and related techniques
Enhancing product image quality through SWAV and related techniques is a significant step forward in AI-driven image generation, especially for e-commerce. By using self-supervised learning, SWAV doesn't just improve image clarity but also ensures that key details stand out while irrelevant elements are toned down. Its adaptability lets it create a wide range of product visuals from relatively small datasets, making it ideal for fast-paced markets. This advancement promises to make product representation more efficient and engage shoppers more effectively. However, there are still challenges in capturing intricate details and handling complex variations. As these techniques become more sophisticated, the potential to transform online shopping experiences will continue to grow.
SWAV's approach to image generation is a fascinating departure from traditional methods, with a unique focus on combining feature learning and clustering into a single process. This is intriguing because it minimizes the loss of vital information during transitions between these stages, which is a common issue in more traditional approaches. This suggests that SWAV might excel in retaining crucial details like textures and contours in product images.
Furthermore, SWAV's ability to learn and adapt in real-time is extremely promising. This means that it can adjust to new products or variations without needing extensive retraining, a huge advantage for the dynamic world of e-commerce. Imagine having the ability to instantly update product visuals as inventory changes—SWAV might be able to deliver this level of speed and efficiency.
Another noteworthy aspect is SWAV's memory efficiency. Compared to other deep learning models, it requires less computational power, making it more accessible for smaller e-commerce companies without the hefty resource demands typically associated with this technology. This could be a game-changer in democratizing the use of AI-powered image generation across the e-commerce landscape.
The ability to generate images in complex scenes with multiple objects is particularly exciting. This allows retailers to present products in realistic settings, potentially boosting consumer relatability and driving sales. It's like bringing the product experience closer to reality for the customer, even in the digital realm.
What truly sets SWAV apart is its capacity for semantic understanding. Beyond generating visually appealing images, it can grasp the relationships between different products based on visual features. This opens the door for creating complementary images, like suggesting accessories that match a specific product, which is great for enhancing the shopping experience and cross-selling opportunities.
SWAV's strengths extend to its ability to manage a significant range of variations within a product category. In industries like fashion, where products come in a wide variety of colors and patterns, this capability is essential for accurate representation. It's a significant step towards ensuring that product visuals reflect the actual diversity found in the real world, boosting customer confidence in what they see online.
The fact that SWAV can generate augmented data samples, encompassing variations in angles, lighting, and backgrounds, is a huge asset. It makes the datasets more diverse, which in turn improves model performance in real-world conditions. This is crucial for maintaining consistent image quality, regardless of the context.
It's important to acknowledge that SWAV is still evolving, particularly in its ability to represent intricate product details accurately. However, its continued progress in this area is promising, especially in industries like jewelry and tech, where these details are crucial.
The ability to generate a wide range of product images from a smaller dataset is a significant advantage, both in terms of resource efficiency and customer engagement. Customers are more likely to make a purchase when presented with diverse perspectives on a product. This translates to potential sales growth for e-commerce businesses.
In conclusion, SWAV offers a compelling combination of capabilities, particularly its learning from unlabeled data, generating diverse images, adapting in real-time, and handling complex scenes. This technology has the potential to completely reshape the way we experience e-commerce.
SWAV's Impact on AI-Driven Product Image Generation A Technical Deep Dive - Generative AI's role in personalized product image creation
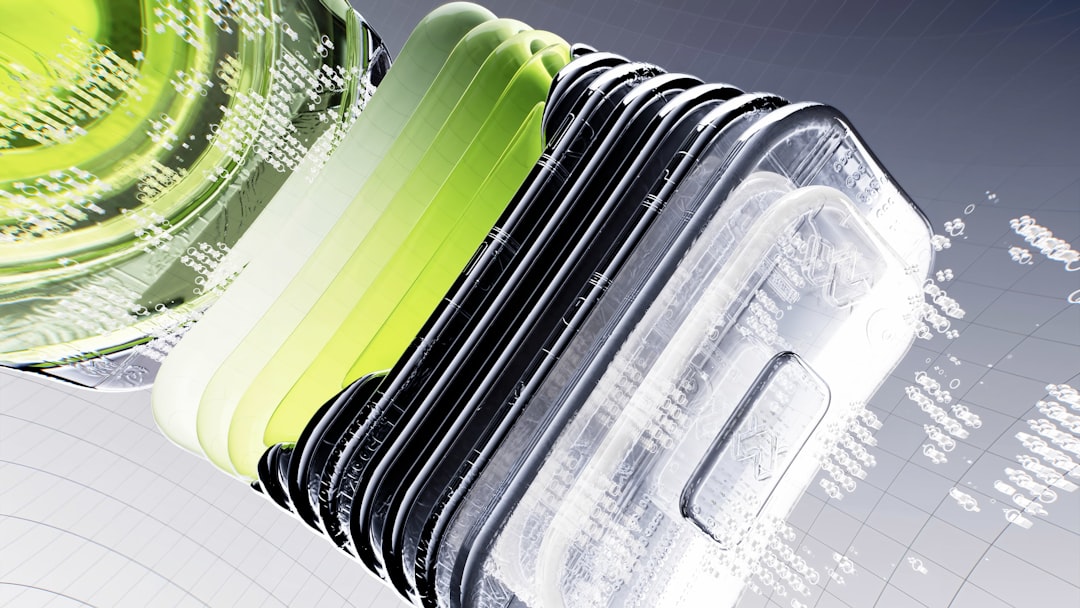
Generative AI is changing the way we create personalized product images. Instead of relying on professional designers, consumers can now customize visuals themselves using these powerful algorithms. Tools like SWAV are making it easier than ever to create unique and engaging product images. SWAV, specifically, can learn from vast amounts of unlabeled data, allowing it to quickly adapt and generate high-quality visuals without the need for extensive human intervention. This is a game-changer for e-commerce, where compelling product imagery is crucial for capturing customer attention and driving sales. However, while the potential is exciting, it's important to consider the ethical implications and the need to address the challenges of accurately capturing fine details and handling complex variations. As generative AI technology continues to improve, it has the potential to transform the online shopping experience, making it more dynamic and personalized for every customer.
Generative AI, through frameworks like SWAV, is leading to a more personalized approach to product imagery. Imagine being able to tailor visuals based on a customer's browsing history or past purchases – this level of personalization could dramatically improve conversion rates. The technology also allows us to create 3D models from simple 2D images, making it easier for consumers to visualize products like clothing and furniture. This means fewer expensive photoshoots and more efficient product catalogs. Beyond aesthetics, these models can be used to create realistic settings for product staging, immersing customers in a simulated shopping experience.
The ongoing learning capabilities of these models mean they're constantly refining their output based on consumer interaction. This feedback loop ensures that the generated images align with consumer expectations, which is critical in a world where visual appeal is paramount. And, we're seeing the emergence of augmented reality (AR) overlays that use AI-generated imagery to help users visualize products in their own spaces. This goes beyond simple visual enhancement – it's about creating a more engaging and interactive shopping experience.
Research suggests that AI-generated product images often surpass traditional photographs in terms of subtle features like lighting and texture. These algorithms can manipulate these elements to make products more visually appealing without introducing distortion. They also allow for the creation of multiple variations of the same product – different angles, settings, and even custom configurations – offering a more comprehensive understanding of the product for the consumer.
SWAV's approach is particularly exciting because it can analyze the visual relationships between products, suggesting complementary items based on a user's interests. This aligns perfectly with marketing strategies that emphasize cross-selling and upselling. And, as the model continues to evolve, we can expect to see even more customized product variations, catering to a growing demand for personalized experiences.
Despite these promising advancements, there's still a lot to learn about handling intricate details and complex product variations. But, as we continue to explore the potential of AI in product image generation, we're moving towards a future where shopping is not only more convenient but also a truly personalized and immersive experience.
SWAV's Impact on AI-Driven Product Image Generation A Technical Deep Dive - AI applications transforming visual content and development processes
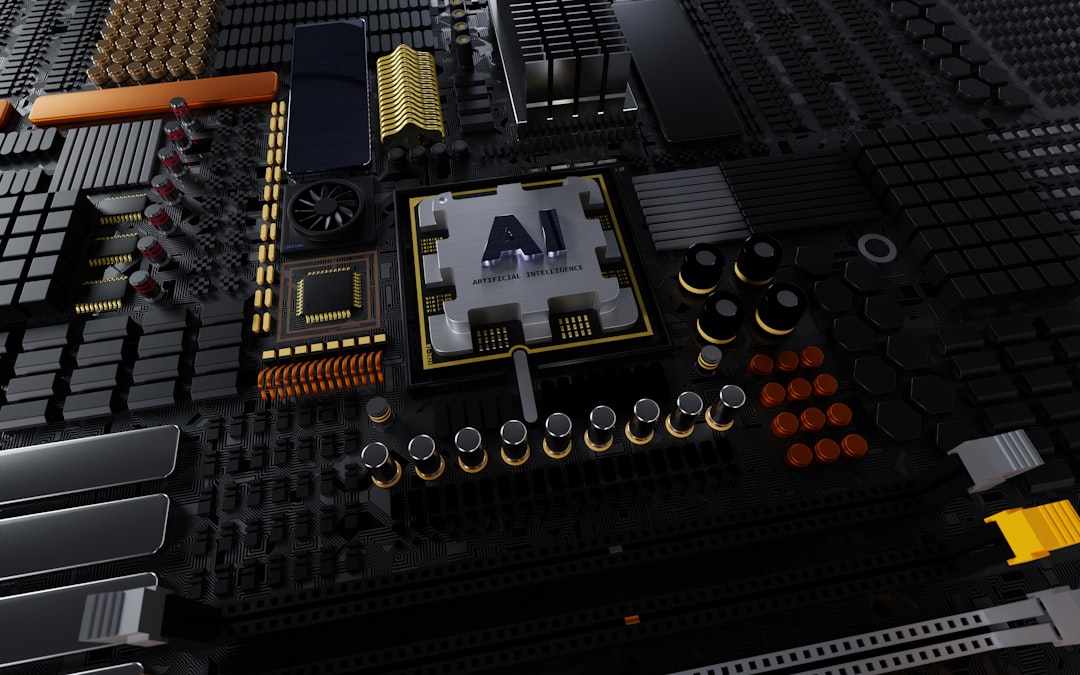
AI is changing the way we create and use visual content, especially in e-commerce. Tools like SWAV are making it easier to automatically generate high-quality product images that are more interesting and relevant to customers. This means we're less reliant on traditional design methods, and even people who aren't designers can make professional-looking visuals. While it's great that we can make images that change based on different products and needs, there's still work to be done in capturing the fine details and complexity of products. As AI gets better, it's likely to make shopping online more engaging and customized for each person.
AI applications, like SWAV, are changing the way we think about generating product images for e-commerce. It's not just about making things look nice anymore - it's about making the process more dynamic and intelligent.
One of the coolest things about SWAV is that it can create product images in real-time, adjusting to things like trends, new products, or even what a customer has looked at before. Imagine an online store where the pictures you see are constantly adapting to your interests.
But that's not all. SWAV can also figure out how products are connected to each other. For example, it can learn that a pair of shoes looks good with a specific outfit. This is a big deal because it helps businesses sell more stuff by suggesting related products.
Another important part of SWAV is how it saves time and money. Unlike older methods, SWAV doesn't require someone to manually label every image. It can learn on its own, which means companies can spend less time on that tedious task. This opens up possibilities for even smaller businesses to use advanced AI technologies.
But it gets even more interesting. AI can bring together text and images, which means that descriptions you read online can actually influence the way a product looks in a picture. This helps to make sure that the visuals match what people expect.
We're also seeing AI generate all sorts of different versions of the same product. This can help customers understand it better because they can see it from different angles and under various conditions. It's like having a virtual photoshoot that happens automatically.
But there are ethical questions to think about too. As AI gets better at tailoring things to individuals, we need to make sure that we use this information responsibly. We don't want to create a situation where people feel like they are being tracked too closely.
Overall, AI is giving us a glimpse into a future where online shopping will be more personal and engaging. The tools are getting more powerful, but we still need to find the right balance between innovation and responsible practices.
SWAV's Impact on AI-Driven Product Image Generation A Technical Deep Dive - Real-time data analysis improving product image adaptation
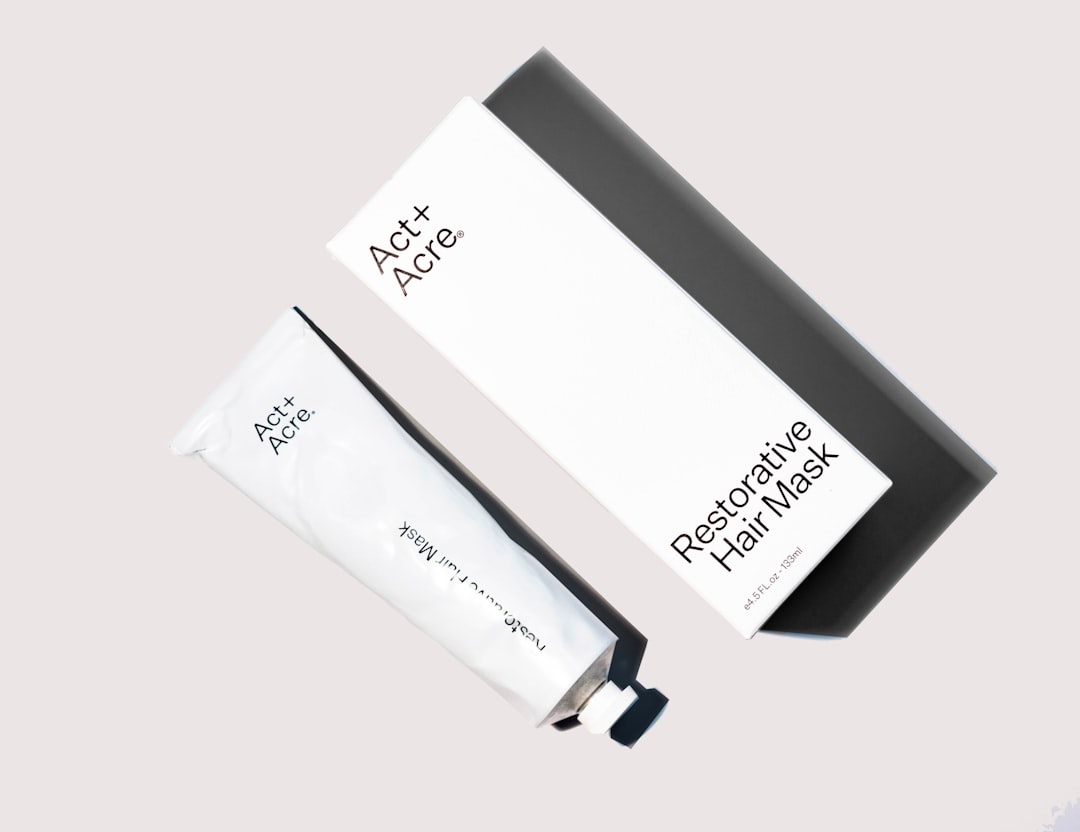
Real-time data analysis is transforming the way product images are created and adapted, particularly in e-commerce. AI models like SWAV can learn from massive datasets, instantly understanding trends in consumer preferences. This allows them to generate custom product visuals tailored to each shopper, making images more engaging. This responsiveness means e-commerce companies can quickly adapt to changing styles or seasonal trends without lengthy retraining, giving them a competitive edge. However, these systems still struggle with capturing minute details and representing intricate products accurately, demonstrating the need for ongoing development. As these technologies mature, they have the potential to revolutionize the online shopping experience, combining personalized visuals with seamless efficiency.
The real-time analysis of data is changing how product images are generated in e-commerce. SWAV, for example, isn't just about pretty pictures; it's about using AI to make images respond instantly to changing trends and customer preferences. It's like having a designer who constantly learns from the millions of decisions shoppers make every day.
This isn't just about changing a color or two. SWAV can figure out the best way to display a product based on how people have interacted with similar images in the past. It's also able to understand the relationship between products, suggesting related items based on what customers are browsing. This is like having a sales assistant that uses AI to recommend the perfect outfit based on what shoes you're looking at.
One of the things that makes SWAV so cool is its ability to generate images in complex scenes. Think about seeing a product displayed in a living room or on a model wearing a specific outfit, instead of just a plain white background. This type of visual realism helps shoppers imagine how the product would fit into their own lives, increasing the chances that they'll buy it.
The technology behind SWAV also allows for the generation of 3D models from 2D images, which is a big deal for e-commerce. Imagine being able to see how a shirt would look on you or how a sofa would fit in your living room - without having to physically try it on. This creates a much more immersive shopping experience.
Of course, there are also ethical considerations with AI-driven image generation. As the technology becomes more powerful, it's crucial that it's used responsibly. For example, we need to make sure that algorithms aren't used to create images that are misleading or exploit users. But if we can use this technology thoughtfully, it has the potential to revolutionize how we experience e-commerce, making online shopping more personal, engaging, and ultimately, more satisfying.
Create photorealistic images of your products in any environment without expensive photo shoots! (Get started for free)
More Posts from lionvaplus.com: