New AI Image Enhancement Techniques Beyond Presets in 2024
New AI Image Enhancement Techniques Beyond Presets in 2024 - AI-Powered Noise Reduction Revolutionizes Low-Light Photography
AI is revolutionizing how we capture images in low-light environments. Traditional methods often struggle to balance noise reduction with detail preservation, leading to images that are either overly smooth or overly noisy. However, new AI-driven software like ON1 NoNoise AI and Topaz DeNoise AI are changing the game. These programs leverage sophisticated algorithms to specifically target high ISO noise, effectively cleaning up images without sacrificing the fine details that give photos life.
Beyond noise reduction, researchers are actively exploring AI systems that can dramatically brighten extremely dark images without introducing the very noise they aim to eliminate. This push into ultra-low light situations represents a significant leap forward. This progress is encouraging photographers to explore AI-powered enhancements that offer much more refined controls than traditional, pre-set filters. This trend signals a shift towards image manipulation that is both smarter and more tailored to individual needs, ultimately delivering a more pleasing and detailed final result, making low-light photography accessible and rewarding for a broader audience.
The field of low-light photography is experiencing a transformation thanks to AI-powered noise reduction techniques. These systems leverage sophisticated algorithms to scrutinize noise patterns at the pixel level, differentiating noise from actual image information. This granular analysis allows for exceptionally precise rendering of fine details within the image.
The foundation of these AI systems often lies in deep learning models trained on massive collections of low-light images. This training allows them to acquire noise reduction strategies that often closely mirror human visual perception and editing practices. These models can dynamically adjust exposure levels across low-light images, resulting in a broader dynamic range than traditional methods which frequently produce blown-out highlights or overly dark shadows.
With advancements in computational power, several AI noise reduction algorithms can now perform image processing in real-time. This capability permits photographers to view enhanced images instantly during the shooting or editing process, overcoming some of the bottlenecks inherent in traditional image editing workflows.
Some of the more advanced methods involve capturing multiple exposures of the same scene and intelligently blending them to enhance detail and reduce noise. By leveraging slight variations in each individual shot, these approaches generate cleaner final images.
Furthermore, AI-based solutions go beyond basic noise reduction. They can effectively minimize artifacts such as color distortion and banding which often appear in poorly lit conditions and are often exacerbated by traditional noise reduction methods. It is quite remarkable how these systems often preserve the integrity of fine details and textures in an image, a trait frequently lost during traditional noise removal.
A new level of control has emerged as many noise reduction tools include adjustable AI parameters. This gives photographers the freedom to balance noise reduction with detail preservation, tailoring the output to their specific stylistic preferences. We're also starting to see a synergistic relationship emerge between AI noise reduction and upscaling tools. These technologies can enhance lower-resolution images while concurrently reducing noise, enabling creation of sharper results from less than ideal image sources.
And it goes further. Some recent AI models are beginning to exhibit the capability to remove watermarks with astonishing precision. More surprisingly, a few can even restore portions of images that have been obscured by these markings, potentially salvaging compromised photographs. The progress is impressive, and we are only starting to grasp the full potential of how AI can fundamentally shift the landscape of image enhancement and recovery.
New AI Image Enhancement Techniques Beyond Presets in 2024 - Real-Time Object Removal Using Advanced Machine Learning Algorithms
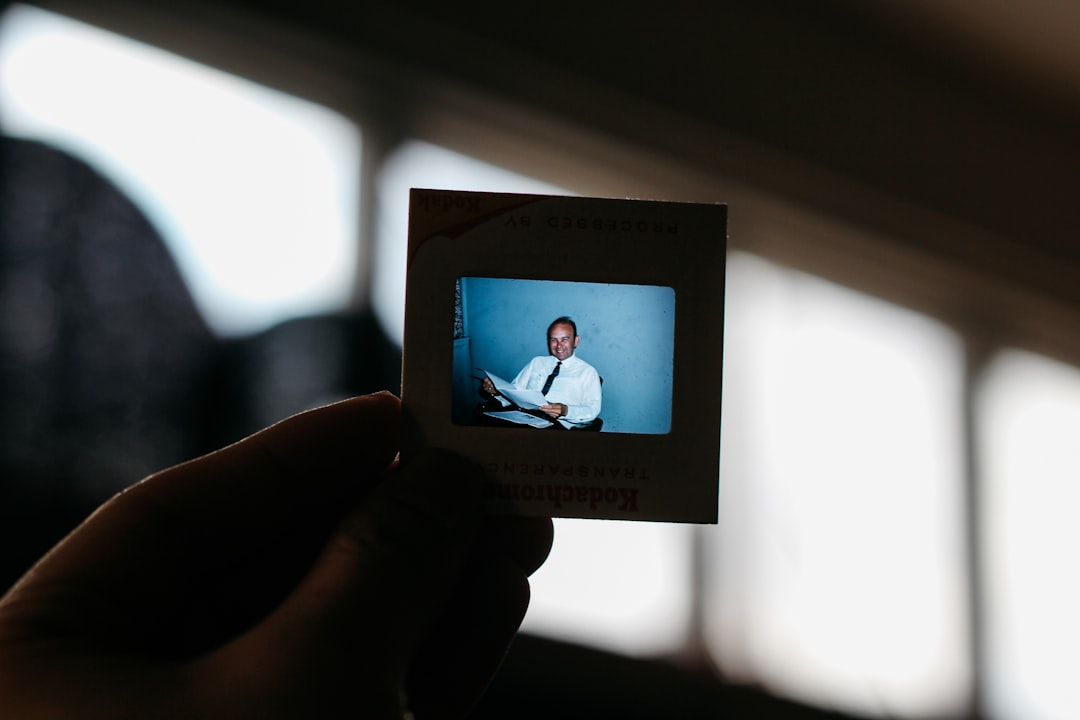
The realm of image editing is being reshaped by AI's ability to intelligently manipulate photos in real-time. One particularly intriguing development is the ability to remove objects from images instantaneously using advanced machine learning methods. These techniques, often relying on generative models like cGANs, are able to seamlessly fill in the gaps left by the removed objects. This filling-in process leverages information about the surrounding elements and colors, resulting in a natural and harmonious reconstruction of the scene.
The practical applications of this capability are vast, allowing photographers to easily declutter their images, remove unwanted intrusions, or focus the viewer's attention on a specific subject. However, achieving this level of sophistication comes with its own set of challenges. The algorithms need to be carefully trained to recognize different object types and seamlessly blend the surrounding elements for realistic results.
There's also a trade-off between the speed and quality of the reconstruction process. While the goal is real-time object removal for a smooth user experience, it's crucial to maintain the original image's integrity and high resolution. Achieving both high quality and rapid processing is an ongoing challenge, but as the underlying algorithms improve and computational resources become more readily available, we can expect more powerful object removal capabilities to become standard in future photography workflows. Ultimately, the emergence of real-time object removal underscores AI's growing influence in providing greater creative control to photographers.
Real-time object removal is becoming increasingly sophisticated thanks to advanced machine learning algorithms. These algorithms, often based on deep learning approaches, are able to process images incredibly quickly, providing photographers with immediate visual feedback as they remove unwanted elements from their photos. This instant gratification streamlines the editing workflow, making the entire process feel more interactive and intuitive.
One of the key features of these newer methods is their ability to consider the surrounding context when removing an object. Traditional methods often leave behind noticeable artifacts or unnatural transitions, but these new AI-powered techniques intelligently analyze the surrounding pixels to create a more realistic fill-in. It's quite impressive how they can reconstruct missing portions of the image in a way that seems seamlessly integrated with the rest of the scene, sometimes requiring little to no manual intervention.
Generative Adversarial Networks (GANs) have emerged as a powerful tool within this field. These networks consist of two competing neural networks – one generating plausible image content while the other analyzes it for authenticity. This adversarial approach results in the generation of incredibly realistic details during the object removal process.
Interestingly, these algorithms frequently use a multi-layered approach. Instead of using a single, broad-stroke neural network, they may employ different networks to address various facets of the image—like texture, color, or shading. This specialized approach enhances the overall coherency of the edited result.
Another fascinating aspect is how these AI systems learn from huge datasets of images. They don't simply remove objects; they've been trained on countless examples of real-world photographs, enabling them to deduce plausible backgrounds based on similar scenes. This ability to extrapolate knowledge from a vast pool of images allows them to replicate human-like editing decisions with surprising accuracy.
Beyond basic object removal, these algorithms are becoming remarkably adaptive. They can intelligently recognize different types of objects and textures—like fur, hair, or fabrics—and process them appropriately during the removal process. It's an area where the adaptability of the algorithm is critical to maintain the integrity of the image.
Even more intriguing is the extent to which these techniques are finding applications beyond their original purpose. For example, algorithms initially developed for object removal are starting to be used in other areas, such as image upscaling or even recovering damaged image textures. This broader scope speaks to the inherent versatility of the underlying principles.
Furthermore, modern tools often offer users more control over the AI process. While these methods are capable of fully automated enhancements, many now give users the ability to fine-tune parameters or adjust the level of intervention. This brings together the benefits of intelligent AI automation with the familiar experience of traditional image editing, allowing users to achieve both speed and precision.
However, as these tools become more powerful and widespread, there are legitimate ethical concerns to consider. The ease with which they can realistically alter images raises questions about authenticity in photography. With the potential to convincingly modify photos, it becomes more challenging to distinguish between a genuinely captured scene and a manipulated one. This presents unique challenges in an era where photography is often seen as a way to document and preserve reality.
Finally, the accessibility of these technologies is changing as well. Powerful machine learning algorithms are finding their way into mobile photography apps, making sophisticated editing tools available to a broader audience. This democratization of image manipulation puts the ability to achieve professionally edited results within the grasp of anyone with a smartphone, regardless of their technical background or prior editing experience. This is perhaps one of the most profound impacts of these developments—the power to create and modify images in creative and compelling ways becomes truly universal.
New AI Image Enhancement Techniques Beyond Presets in 2024 - Intelligent Color Grading Beyond Traditional LUTs
The realm of color grading is experiencing a shift with the emergence of AI-powered tools that extend beyond traditional LUTs. These new tools, including programs like Match AI and Colourlab Ai 2.0, are using machine learning to refine and automate color correction in ways that were previously unavailable. They introduce capabilities like Smart LUTs, allowing users to easily replicate specific color palettes, and also facilitate intelligent shot organization based on visual similarity, significantly streamlining the workflow for color grading projects.
This move toward AI-driven color grading not only makes the process faster and more precise but also gives creators the ability to tie the visual language of their images more directly to the narrative tone they're trying to convey. The technology even makes it easier for creatives to replicate the look and feel of their favorite movies in their own work, opening up a whole new range of artistic possibilities.
Furthermore, the integration of these AI tools with major editing software platforms means that filmmakers and photographers of all experience levels can take advantage of these advances. This trend towards more intelligent, adaptive color grading techniques might make traditional, preset-based solutions feel increasingly restrictive. The current development marks a clear move towards leveraging AI for enhancing and refining visual storytelling, offering a level of control and customization that was not readily available before.
Moving beyond the limitations of traditional LUTs, we're seeing the emergence of intelligent color grading powered by AI. These systems go beyond simple global color adjustments by analyzing the image's content and context, allowing for far more nuanced and precise alterations. Instead of applying the same color changes across the entire image, as LUTs do, AI-powered grading can target specific areas, such as foreground or background objects, enabling tailored adjustments based on the scene.
The ability to modify color balances at the pixel level opens up exciting possibilities for fine-tuned image manipulation. Additionally, these systems are becoming increasingly adaptive, offering real-time feedback during adjustments, enabling on-the-fly color experimentation during a shoot. This interaction with the color grading process offers a level of control previously unavailable.
Furthermore, researchers are training AI systems on vast collections of images from diverse artistic styles, enabling them to learn the subtleties of color grading from renowned photographers. This allows users to replicate complex color palettes and grading approaches within their own work, effectively mimicking the visual aesthetics of professionals. This is a substantial step forward compared to static LUTs that offer only a limited range of predefined styles.
These AI-driven tools are also adept at handling dynamic range, effectively boosting shadows and retaining highlight details without the clipping issues commonly seen with traditional color grading. The ability to intelligently manage dynamic range contributes to a more controlled and vibrant final image.
Beyond the technical capabilities, new algorithms are starting to understand the content of the image. This means that they can apply differential color adjustments based on subject recognition, such as enhancing skin tones while maintaining the overall scene's mood. This is particularly useful for portrait photography, where a delicate balance is needed.
The algorithms are also becoming increasingly sophisticated in applying color theory principles, ensuring the final color palettes are aesthetically pleasing and in harmony with the subject matter. This automatic application of color harmony concepts offers a pathway to more visually appealing images.
The synergy between AI color grading and upscaling techniques is noteworthy. The ability to apply color corrections in conjunction with resolution enhancements ensures that the upscaled image maintains its color fidelity. This combined approach creates images that are sharper and more detailed with accurate color representation.
Some groundbreaking systems are even developing the ability to seamlessly transition between multiple color grading styles within a single image or video. This offers exciting creative storytelling potential, allowing filmmakers and photographers to control visual continuity while using distinct color palettes within different sections of their work.
One area where intelligent grading is proving particularly beneficial is anomaly detection in color profiles. Some advanced tools use algorithms to identify inconsistencies or unusual color shifts in an image, allowing for faster identification and correction of issues that may otherwise be missed during manual adjustments. This automated quality check promotes a consistent color palette across an entire project.
Despite the impressive advancements, it's important to remain mindful of potential limitations and unforeseen consequences. While these tools are becoming increasingly powerful, the development and application of these intelligent color grading tools still present unique challenges and questions about the future of image manipulation and authenticity. Nonetheless, the ongoing research in this field continues to unlock powerful capabilities, suggesting an exciting future where the process of color grading becomes both more efficient and incredibly creative.
New AI Image Enhancement Techniques Beyond Presets in 2024 - Automated Composition Correction With Dynamic Cropping
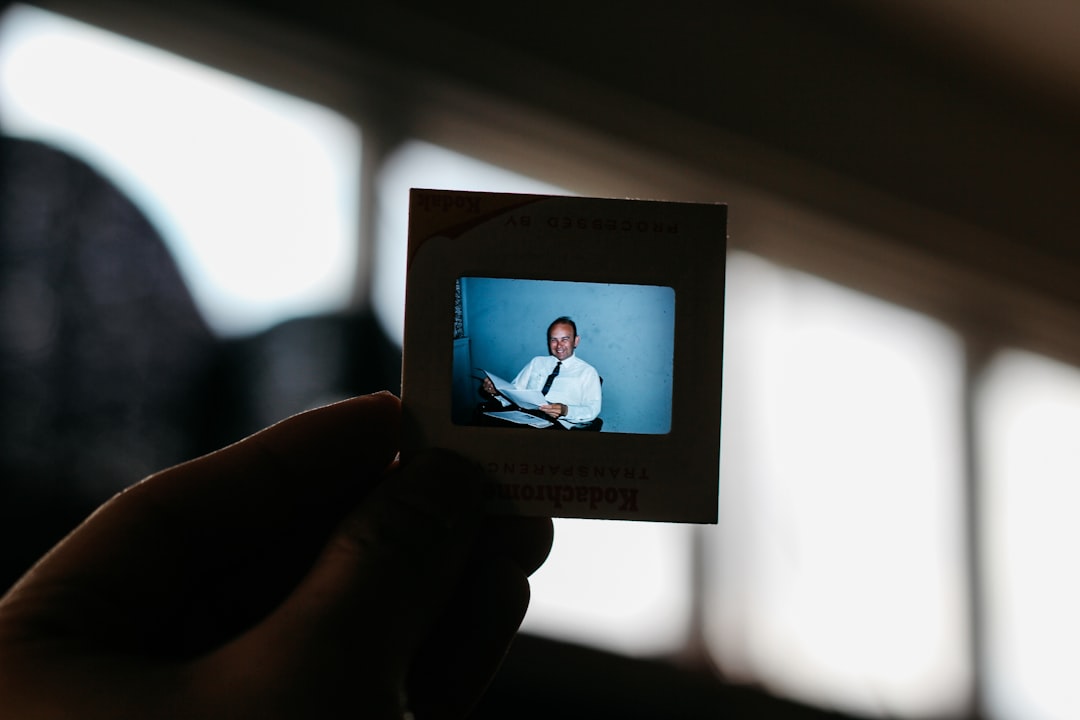
AI is pushing the boundaries of image editing with automated composition correction using dynamic cropping. Instead of relying on traditional, often subjective cropping methods, new AI systems analyze massive datasets of images to learn what makes a composition visually appealing. These AI models go beyond simply identifying objects and can now intelligently suggest cropping options based on the overall context and elements within each image. This dynamic approach means the cropping suggestion adapts to the intricacies of the specific photograph, rather than applying a generic rule.
The AI algorithms prioritize key visual elements, aiming to improve the aesthetic balance of the image through these automated cropping recommendations. This evolution in image processing demonstrates a shift toward more sophisticated and personalized image enhancements, moving beyond the constraints of preset options. As AI algorithms become more refined, we can anticipate a future where understanding image composition becomes deeply integrated into image editing workflows, offering photographers new avenues for creative expression and a deeper level of control over their final image. While the promise is significant, it's also important to acknowledge the evolving nature of AI and the potential need for careful consideration of how this technology impacts the perception and authenticity of photographic works.
Automated composition correction through dynamic cropping is an interesting development in image enhancement. It's essentially a system that intelligently analyzes an image's composition and suggests, or even automatically applies, the best cropping to highlight the most visually appealing aspects. The goal is to improve the overall story the photograph is trying to tell without the need for manual adjustments.
A key element is the ability to dynamically crop while maintaining the image's original aspect ratio. This is crucial because it avoids distortions and keeps the integrity of the image intact. The algorithms achieve this by figuring out the best framing for the photograph with minimal user input.
Furthermore, these tools often use machine learning to understand the context of an image. They can detect elements like people or objects, automatically adjusting the crop to ensure the focus is on the most important parts of the scene. This makes the images more engaging and the narrative easier to follow.
We're even seeing some tools try to understand the typical viewer and adjust the composition based on those expectations. This audience-driven approach is meant to improve the relevance of the image and lead to a more satisfying experience for the viewer.
Interestingly, the combination of dynamic cropping with upscaling techniques is becoming a standard feature. By smartly resizing the image after cropping, the focus is enhanced, and the resolution often seems improved, particularly around the main subject.
This type of intelligent cropping often helps reduce the annoying artifacts you sometimes get with traditional cropping methods, like pixelation or blur. The AI-powered tools can fill in missing information by looking at nearby pixels, making the adjustments smooth and seamlessly integrated.
Many tools now give photographers real-time feedback as they change the crop, making the editing process more interactive. This allows for faster, more informed decisions without extensive post-editing.
However, some AI models are exploring more nuanced concepts. A few are being trained to understand cultural nuances and storytelling traditions within visual elements, implying that they could adapt cropping based on the intended audience and their cultural background. This is a fascinating exploration of how AI can respect and reflect cultural sensitivity within image composition.
Dynamic cropping techniques appear to be flexible enough to work with various formats like still images, videos, or even social media post sizes. This adaptable nature ensures creators can maintain a consistent level of visual quality and composition across different media.
Although the advantages of automation are compelling, discussions within the photography community are ongoing about finding the balance between artistic control and relying on AI for composition. Striking the right balance is key, ensuring that photographers can retain their creative vision while using the power of advanced tools. It's a trade-off that continues to be explored as AI integration in photography matures.
New AI Image Enhancement Techniques Beyond Presets in 2024 - Multi-Frame Super-Resolution for Ultra-Sharp Images
Multi-Frame Super-Resolution (MFSR) is a leading-edge AI image enhancement method that aims to deliver ultra-sharp results. Essentially, it leverages multiple images taken from slightly different viewpoints or times to synthesize a higher-resolution output. This approach utilizes advanced algorithms that intelligently combine the individual frames, effectively recovering fine details that might be lost in lower-resolution versions of the same scene. While MFSR has shown considerable promise in boosting image quality, it faces limitations, particularly when attempting extreme magnification. Trying to reconstruct images at drastically increased resolutions can reveal weaknesses in the process. This points to ongoing research and development aimed at enhancing the performance of MFSR. The use of transformer-based architectures in newer models holds promise in refining the reconstruction process, bringing us closer to images where upscaled resolution is nearly indistinguishable from the original. This field promises a future where achieving incredibly sharp and detailed photos, even from less than ideal sources, may be achievable.
### Surprising Facts About Multi-Frame Super-Resolution for Ultra-Sharp Images
The pursuit of ultra-sharp images has led to fascinating advancements in super-resolution techniques. Among these, multi-frame super-resolution (MFSR) stands out for its ability to leverage multiple images captured from slightly different angles or time points to produce a single, higher resolution image. It's a powerful technique that can create results that often surpass what's achievable with a single image alone.
One interesting facet is the way MFSR enhances temporal resolution. By intelligently combining frames from videos or burst sequences, it can significantly improve clarity and reduce the blur often associated with motion. It does this by exploiting the subtle differences between frames to extract details that might be hidden or too subtle in a single frame.
Moreover, MFSR excels at achieving a higher level of sharpness through a process of intelligent pixel interpolation. Instead of simply upscaling, it strategically calculates new pixel values based on the data across several frames. This gives us a reconstructed image that can often far exceed the native resolution of the camera sensor.
An intriguing aspect is how MFSR tackles a common imaging artifact called aliasing. Aliasing occurs when the fine details in a scene are too intricate for the sensor to capture accurately, resulting in jagged edges or moiré patterns. By taking advantage of the multiple frame information, MFSR is capable of mitigating these visual flaws and produces a smoother, more accurate rendering of fine detail.
Even with minor camera movements or unintentional shifts between captures, MFSR remains surprisingly effective. The algorithms can handle the slight misalignments or shifts that are common in practical situations, such as handheld shooting. This robustness makes it a more practical and versatile tool for improving image quality, especially in uncontrolled settings.
Some of the more advanced MFSR techniques now utilize neural networks, and these models learn from extensive datasets of images. They're trained on pairs of high-resolution and low-resolution images, essentially allowing them to develop a visual intuition that helps them reconstruct subtle details and texture.
Beyond its technical aspects, MFSR gives photographers an exciting opportunity for creative experimentation. It can even encourage creative capture techniques. By intentionally capturing multiple images with small variations like exposure or focus point, photographers can further enhance the output during post-processing, potentially creating images far more detailed than they could with a single shot.
Further, the integration of MFSR with established noise reduction techniques creates a potent combination for producing pristine images. Since the noise patterns can be averaged across multiple frames, MFSR can effectively minimize noise, producing sharper results with a reduced loss of detail.
The growing computational power available today is also impacting MFSR. Certain implementations have moved into real-time processing, meaning that users can now experience enhanced image quality in a more responsive way. This is especially beneficial when capturing dynamic scenes where swift visualization of results is critical.
Interestingly, the impact of MFSR extends beyond the world of photography. We see it being explored in fields such as medicine (improving the resolution of medical scans), satellite imagery (creating more precise maps), and forensic science (assisting in investigations). In all these domains, sharper images have the potential to improve outcomes and provide deeper insight.
However, the power of MFSR also raises important questions regarding image authenticity. It's now much easier to create highly enhanced versions of pictures, which brings challenges in terms of determining what’s real or modified. This raises some ethical considerations about image manipulation and its influence on how we perceive and interact with photography.
MFSR is a continuously evolving area of research and development, with the potential to transform how we capture and interact with images. While there are some ethical considerations that need continued discussion, the improvements in image clarity and detail that it provides are pushing the boundaries of digital imaging, making it a compelling area of research and a potent tool for photographers and image analysts.
New AI Image Enhancement Techniques Beyond Presets in 2024 - Generative Fill for Seamless Photo Expansion and Restoration
AI-powered Generative Fill offers a new way to manipulate images, allowing for seamless expansion and restoration. This technology uses AI algorithms to intelligently fill in missing areas of a photo, whether it's expanding the boundaries of an image or repairing damaged sections. It's a tool that's accessible to both professionals and amateur photographers, fostering creativity through the ability to change backgrounds, insert new objects, or restore older, worn photos. The technique also ensures that the image maintains quality across various aspect ratios, making it useful for a wide array of tasks, including marketing and artistic projects. However, alongside its advantages, there's a growing discussion around the potential impact on the authenticity of photography. With Generative Fill making it so easy to alter images, there's a risk that the line between genuine captured moments and manipulated photos could become blurred. The future of image editing relies on finding a balance between the incredible creative potential of these tools and the ethical responsibility that comes with their widespread use.
Generative Fill, powered by AI, is a fascinating technique that lets us seamlessly expand and restore photos by filling in missing or damaged areas with AI-generated content. It's a tool that's becoming increasingly accessible, making it useful for both photography enthusiasts and professionals alike. You can use it to replace boring backgrounds with something more exciting—imagine swapping a mundane park for a tropical beach. It even opens doors for experimentation with things like hairstyles and accessories in portraits, adding a fresh layer to personal creativity within photo editing.
Platforms like ExtendImageAI and others are making these tools readily available, allowing exploration of different image options and enhancing all kinds of digital projects, from ads to social media posts. It shines particularly brightly when it comes to photo restoration. We can use it to bring old or damaged photos back to life by intelligently filling in the gaps, ultimately boosting their overall quality and restoring memories.
One of the noteworthy aspects of this technology is its ability to maintain high-quality visuals across different image proportions when expanding photos. This unlocks new avenues for creative manipulation and expression, offering a wider canvas for artists and photographers. Tools like Adobe Photoshop's Generative Fill are designed for user-friendliness, making photo restoration and enhancement achievable with just a few clicks.
The capability to add new objects or elements that weren't in the original image opens a path to dynamic and exciting image editing. Moreover, it's often integrated with other tools, like those in Picsart, allowing for further refinement, even creating entirely new backgrounds.
It also incorporates 'outpainting' features, expanding images by extending the cropping boundaries seamlessly. This capability offers a new perspective on image editing, going beyond simple cropping. In essence, generative fill has quickly become a popular tool for various applications, from digital art to marketing and social media, profoundly impacting how we generate and enhance visual content in 2024.
The sophistication of the algorithms is noteworthy; they analyze surrounding pixels and the image's context to create realistic additions. They are capable of not just filling holes but often can intuitively suggest better compositions, leading to more visually appealing results. This is in part due to these AI models being trained on massive datasets of various image types. However, this comes with an inherent speed versus quality trade-off. Faster processing can sometimes result in less nuanced results.
The ability to understand an image's context goes beyond simple inpainting. It allows for more semantic reconstruction, recognizing objects and scenes within the image and adjusting output accordingly. It leads to results that fit the overall photographic narrative rather than being a mere copy of nearby textures. While extremely impressive, this advanced manipulation raises legitimate questions about authenticity in photography. It's getting increasingly harder to distinguish between a genuine and a manipulated photo, potentially impacting public trust.
On the positive side, these generative fill methods work across a variety of image formats and resolutions. They're also extremely flexible when used alongside other AI tools like noise reduction or upscaling. They can take a low-quality image and, with a combination of these AI techniques, deliver surprising results. Importantly, the user often has control over the parameters, blurring the line between AI assistance and human creativity in photography. Lastly, some advanced systems are designed to learn from user interactions, further refining the output over time. While still a rapidly evolving field, Generative Fill presents exciting possibilities in image editing, although as always, ethical considerations in visual manipulation need careful consideration.
More Posts from lionvaplus.com: