Amazon Bedrock's Metadata Filtering A Game-Changer for AI-Driven Product Image Generation
Amazon Bedrock's Metadata Filtering A Game-Changer for AI-Driven Product Image Generation - Metadata Filtering Enhances AI-Driven Product Image Generation Accuracy
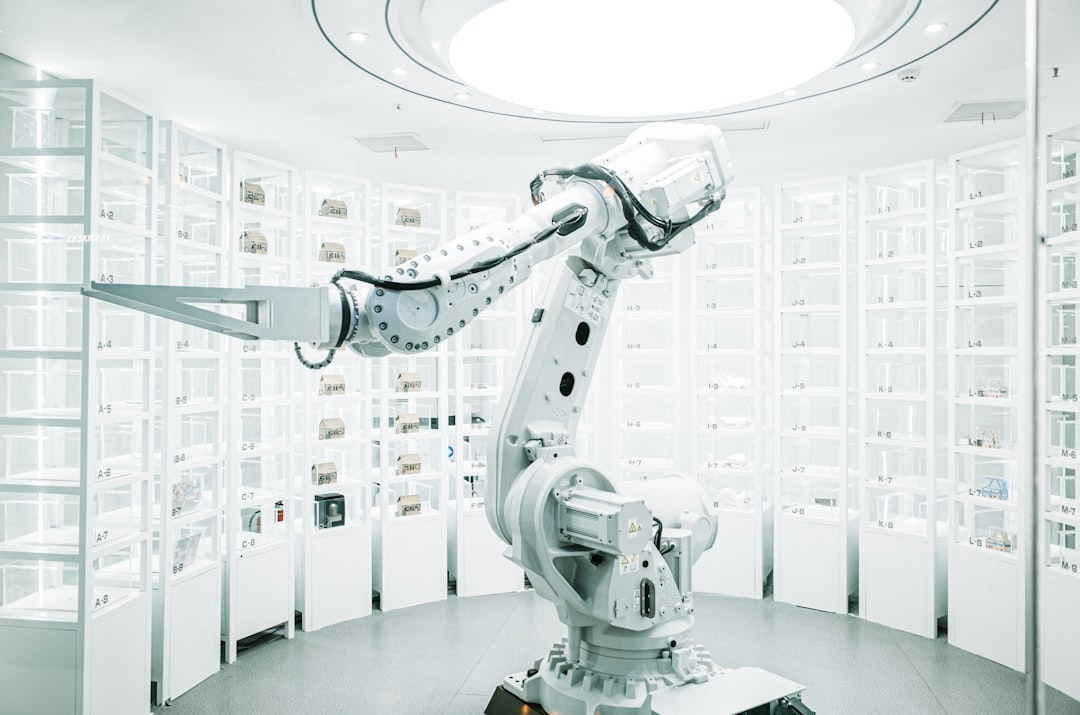
The ability to filter information based on metadata is revolutionizing how AI generates product images, especially within e-commerce. This filtering process allows for more precise control over the image creation process by enabling users to specify exactly what they need. This targeted approach ensures that the resulting images are not just relevant, but also meticulously aligned with specific requirements. Whether it's creating realistic product staging scenes or generating engaging images for marketing campaigns, metadata filtering empowers AI to produce visuals that are more accurate and effective.
The effectiveness of metadata filtering hinges on the availability of rich datasets with detailed annotations. Datasets like the Amazon Berkeley Objects Dataset, with its wealth of information about each product including size, materials, and brand, provide the AI with crucial details for generating highly accurate and contextually relevant images. By leveraging the power of metadata, AI can generate images that effectively showcase products and resonate with the target audience. As the reliance on AI for product visuals grows, the importance of meticulously managed and comprehensive metadata cannot be overstated. It's becoming a vital component for businesses hoping to leverage AI-driven visual content in a meaningful and successful way.
When it comes to generating product images using AI, metadata filtering acts like a powerful lens, focusing the AI's efforts on creating more accurate and relevant visuals. By filtering based on characteristics like product color, material, or even the intended use scenario, the AI can hone in on the specifics of the desired image. This increased precision leads to a more desirable outcome for online shoppers, potentially resulting in higher click-through rates on e-commerce sites.
The quality of generated images benefits as well. AI models trained on filtered metadata appear to produce fewer flaws or inconsistencies, contributing to an overall improvement in perceived image quality. It seems that the more organized and relevant the metadata, the more precise and efficient the AI model becomes in its image generation, requiring less computational power to achieve a high-quality output.
Furthermore, metadata filtering can enable AI-driven image generation to be more adaptive to regional variations. By filtering based on geographic preferences, we could see product images tailored to specific markets without requiring manual adjustments for each campaign. This automated adaptation can lead to more effective and targeted marketing efforts.
Interestingly, despite the many upsides, a significant portion of e-commerce companies haven't yet implemented these kinds of advanced metadata filtering techniques. There's a potential opportunity here for businesses to differentiate themselves by leveraging AI-driven product image generation with robust metadata filtering, potentially leading to lower return rates and customer satisfaction. It seems that those businesses actively using metadata effectively might have a competitive advantage, but there are still many who could benefit from exploring this area more fully. It might be that awareness and knowledge of metadata capabilities isn't widespread enough for full adoption just yet.
Amazon Bedrock's Metadata Filtering A Game-Changer for AI-Driven Product Image Generation - Improved Retrieval Augmented Generation for E-commerce Visual Content
The emergence of Improved Retrieval Augmented Generation (RAG) presents a new frontier in creating product visuals for e-commerce. RAG integrates the ability to retrieve and incorporate real-world data with existing AI-powered image generation techniques. Essentially, it bridges the gap between the fixed information traditional AI models are trained on and the ever-changing needs of online shopping, especially for niche products or specific details.
Instead of relying solely on a pre-set dataset, RAG allows the AI to access a more flexible, dynamic knowledge base as it generates images. This dynamic access helps the AI generate images that are more precisely tailored to particular product details or customer preferences. Beyond that, the process of fetching and using this information can be optimized to lower the overall cost of image creation. This is a welcome development as the creation of high-quality, diverse product images can be expensive.
The flexibility that RAG provides is particularly useful in e-commerce, where businesses might need to quickly adapt to new trends or customer demands. This flexibility could potentially help improve marketing campaigns as well as user experience. Despite this promise, there are challenges related to constantly updating the information RAG accesses and managing the large amounts of data that can be used. These issues need to be further addressed before RAG becomes fully integrated into the visual content creation processes of e-commerce platforms.
Enhanced AI-driven product image generation in e-commerce is increasingly relying on a technique called Retrieval Augmented Generation (RAG). RAG essentially incorporates a flexible knowledge base – think of it as a dynamic memory – that allows AI systems to easily incorporate new information and handle a wide range of product details, even the less common ones. This is a big step forward for AI image generation, since traditional AI models are trained on fixed datasets and may struggle with unique or evolving product characteristics.
RAG works by using retrieval mechanisms to provide the AI with relevant real-world data. This bridges a critical gap: the static knowledge of large language models (LLMs) doesn't always align with the ever-changing demands of the e-commerce world. RAG solves this by ensuring that the AI has access to up-to-date information that can be tailored to the product or marketing campaign at hand.
The benefit of this approach is evident in how AI tackles Artificial Intelligence Generated Content (AIGC). By drawing on external knowledge sources, the AI can produce more tailored and detailed outputs. This is extremely helpful in product visualization where a high degree of specificity is needed, for example, accurately displaying the texture of a fabric or depicting a specific product usage scenario.
While foundational AI models and the availability of rich datasets have propelled AIGC, challenges still persist, such as efficiently updating knowledge and managing generation costs. RAG helps to alleviate these. It facilitates more efficient management of information during the image creation process, reducing the computational resources needed.
RAG's capabilities extend beyond basic image generation. Studies have shown its effectiveness in sophisticated multi-step question-answering tasks, implying that it can refine queries and extract information more efficiently. This suggests its potential to generate images that not only look good but also effectively convey information to customers.
The shift towards RAG is significant. E-commerce, in particular, can leverage it to gain real-time access to updated information, allowing them to generate images that are relevant to current trends and customer interests. This isn't just a theoretical benefit; companies are increasingly embracing RAG and other generative AI tools. Examples like Stable Diffusion demonstrate how RAG can help create visually compelling product images.
While the potential is undeniable, there's a lot to explore still. The continued development and application of RAG will likely refine the image generation process even further. It will be interesting to see how RAG evolves, and if it can overcome the challenges of ensuring the consistency and accuracy of AI-generated product images.
Amazon Bedrock's Metadata Filtering A Game-Changer for AI-Driven Product Image Generation - Single API Access to Multiple AI Models for Product Staging
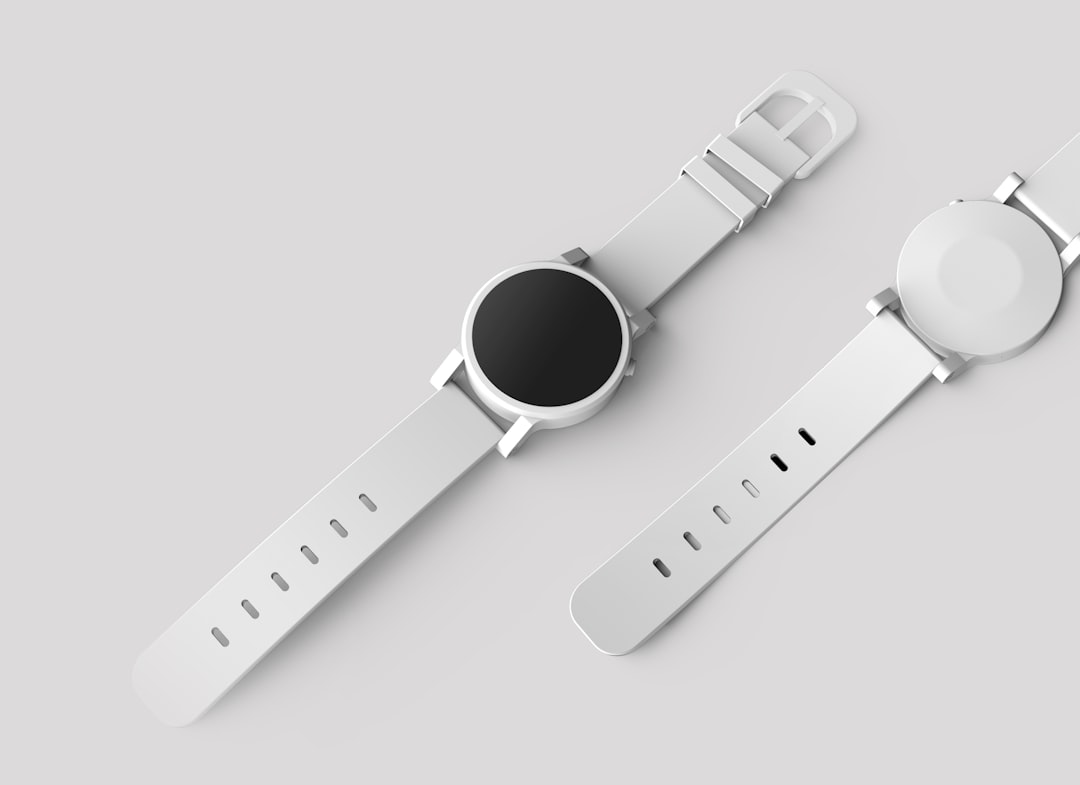
Giving access to a variety of AI models through a single API is a big step forward for creating product images used in online stores. This approach, which utilizes platforms like Amazon Bedrock, lets businesses easily tap into a range of powerful AI models from top developers in the field. They can then create product visuals specifically designed for different markets and audiences. This unified access simplifies workflows and improves the diversity and quality of the product images, making them more visually appealing. As AI technology for creating images matures, this method could produce more detailed and appropriate images that consumers respond to, increasing engagement and sales. Sadly, many businesses still rely on outdated methods, and it's this group that has the biggest opportunity to benefit from these advances, offering a path to innovation.
Imagine having a single, central point of access to a diverse range of AI models, all ready to generate product images for your e-commerce site. This is essentially what a unified API offers – a gateway to different AI engines, like those from Stability AI, Anthropic, or even Meta. It's like having a toolbox full of specialized image creation tools, all controllable through one interface.
The benefit of this approach is pretty obvious: developers can integrate multiple AI models into their systems without needing to learn the intricacies of each individual model's API. This flexibility means businesses can tap into the strengths of different AI engines to produce a variety of product visuals. Want a hyper-realistic product shot? One AI model might excel at that. Need to create a fun, quirky image for social media? Another AI might be perfect for that task.
However, this convenience comes with a trade-off. It can be tricky to maintain consistent output across different AI models. Each model may have slightly different strengths and quirks, leading to variability in the resulting image quality and style. It's something developers need to factor in when designing their image generation workflows. There is also the possibility of a vendor lock-in with this approach as some API providers may enforce specific constraints on their usage.
Another interesting aspect is the potential for faster A/B testing. With multiple AI models at your fingertips, it becomes easier to generate various versions of a product image and quickly evaluate their effectiveness in driving customer engagement or conversions. This can be a huge time-saver, as the time required to run A/B tests using traditional methods can be quite significant.
Beyond generating basic product shots, a unified AI model approach could enable us to create more sophisticated image types. Imagine creating dynamic staging scenarios, showcasing the product in various usage contexts. Or, how about leveraging semantic image retrieval to find images that resonate with specific target customer segments? These features could improve customer experience and enhance engagement with the product.
It's still early days in exploring this approach, but the potential impact is massive. It is important to explore if these capabilities truly deliver what is promised and if these advantages outweigh the potential drawbacks. But, it's likely that as AI technology continues to evolve, we will see more adoption of unified AI APIs in e-commerce and other domains as businesses strive for speed and efficiency.
Amazon Bedrock's Metadata Filtering A Game-Changer for AI-Driven Product Image Generation - Document Chatbots Revolutionize Product Information Retrieval
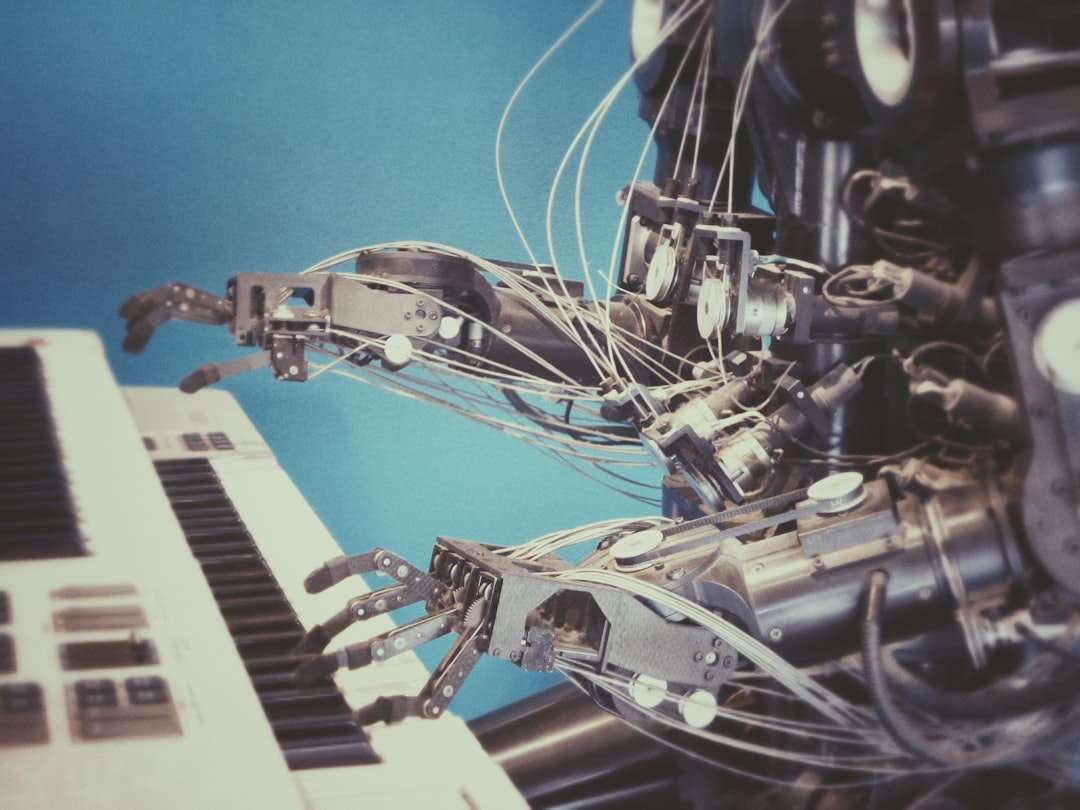
Document chatbots are rapidly changing how we find product information, especially in online shopping. These chatbots, often built using technologies like Amazon Bedrock's Retrieval Augmented Generation (RAG) framework, can quickly search through large collections of product documents to provide shoppers with accurate and specific details. This makes finding the information you need faster and more reliable, especially when dealing with many different kinds of data. As customer expectations for convenient access to information continue to rise, AI-powered chatbots help businesses to readily provide detailed product information, potentially boosting customer engagement and satisfaction. However, their success largely depends on the quality of the data used to train them and the ability to keep that data updated with the latest product information. The accuracy and usefulness of the chatbots ultimately hinge on these factors.
Document chatbots, powered by technologies like Amazon Bedrock's Knowledge Bases, are transforming how customers access product information, particularly within e-commerce. These AI-driven assistants are designed to interact with internal document repositories, offering a more streamlined and efficient approach to product discovery.
One key aspect is the use of Retrieval Augmented Generation (RAG). This approach blends information retrieval with natural language generation, allowing the chatbot to not only understand a customer's request but also to intelligently source relevant information from diverse data sources, including internal documentation and databases. This is a significant improvement over traditional search methods, as it moves beyond simple keyword matching to offer more comprehensive and contextually aware responses. Companies can easily ingest their own data into these systems without needing to rebuild their entire infrastructure.
The integration of proprietary data into generative AI applications through Amazon Bedrock is also noteworthy. This enables businesses to leverage their own unique product knowledge and tailor the chatbot experience to their specific needs. However, this approach also raises concerns about data privacy and security, especially when dealing with sensitive customer or product data. Hopefully, as this technology develops further, those challenges will be addressed.
While the development of chatbots within the Amazon Bedrock environment is facilitated through a combination of services like Kendra and Lex, it requires a thoughtful architectural design involving multiple components, potentially including an Amazon ECS cluster and Application Load Balancer. This complexity underscores the importance of carefully considering infrastructure requirements and the potential integration challenges.
Beyond the technical aspects, the adoption of document chatbots promises various benefits for e-commerce businesses. For instance, they offer 24/7 availability, addressing a significant challenge in traditional customer service models. They can also minimize human error during information retrieval, leading to more accurate and reliable product information for customers. And they provide a valuable source of customer data, offering businesses a deeper understanding of their customers' needs and preferences. This data can be harnessed to optimize product image generation and tailor marketing campaigns more effectively, potentially increasing customer engagement and driving sales.
While the benefits seem promising, there are still some practical concerns. Implementing and maintaining the infrastructure for a robust document chatbot can be complex, requiring technical expertise and ongoing maintenance. And as these chatbots become more sophisticated, there's a growing need to consider ethical considerations, such as biases in AI-generated responses or potential misuse of the technology. These challenges need to be considered alongside the opportunities that AI-powered chatbots offer in streamlining product information retrieval.
Overall, document chatbots have the potential to significantly reshape the e-commerce landscape. By providing customers with quick and accurate access to product information, these tools can enhance the shopping experience, increase sales, and provide valuable insights into customer behavior. We may see this approach being integrated into the front-end of ecommerce sites more readily as time passes. It seems reasonable to expect the development of even more sophisticated chatbot experiences moving forward, creating a new paradigm for product discovery and customer interaction.
Amazon Bedrock's Metadata Filtering A Game-Changer for AI-Driven Product Image Generation - Secure Development of Generative AI Applications for E-commerce
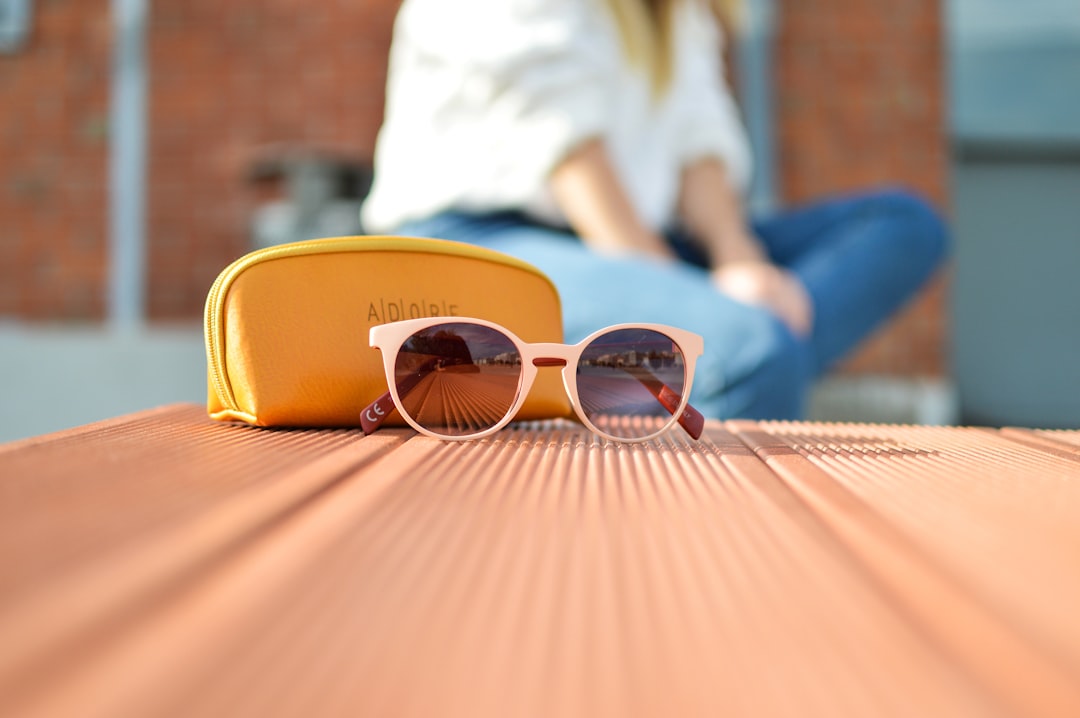
E-commerce is increasingly reliant on generative AI for creating engaging product visuals, but this also necessitates a strong focus on secure development practices. Building these AI applications in a secure manner is crucial for protecting sensitive customer data and maintaining trust. Platforms like Amazon Bedrock provide tools to help ensure the safety of these applications, including safeguards against harmful content and mechanisms to control data access. Features like metadata filtering and RAG allow for more precise image generation, catering to specific product details and customer needs. However, concerns remain around potential issues like the need to manage outputs from various AI models to maintain consistency, as well as the possibility that developers might become overly reliant on a particular provider. Balancing the advantages of generative AI with the need for secure development is vital as the landscape of online shopping evolves. Companies will need to stay ahead of the curve in order to ensure the trust and privacy of their customers, which in the long-run, is important for sustained success.
Amazon Bedrock offers a managed service that simplifies access to a wide range of powerful AI models, including those focused on image generation. Developers can leverage these models through a single API, which can streamline the process of building generative AI applications. This centralized access point makes it easier to experiment with different models and their unique capabilities. For example, developers can explore using AI21 Labs' or Anthropic's language models for generating product descriptions that complement the images. They can also utilize Stability AI's text-to-image models, like Stable Diffusion, to create diverse product images.
However, simply having access to these models isn't enough. Building secure and robust applications requires a deep understanding of the potential risks associated with AI. Bedrock addresses this by integrating strong security measures designed to prevent the generation of inappropriate or harmful content. This is particularly important in e-commerce where product images are often used to promote products to a wide audience. Developers need to consider the ethical implications of using AI and implement safeguards to prevent the unintentional generation of biased or misleading images.
The ease of use and flexibility offered by Bedrock has led to its adoption by a large number of users. It fosters innovation by making it easier for developers to build and deploy generative AI applications. Features like Bedrock Studio further aid this process, providing developers with an interactive environment to experiment with model parameters and fine-tune their applications. Retrieval Augmented Generation (RAG) and techniques like prompt engineering are being incorporated into many of the applications that are being built on this platform. By utilizing these foundational techniques, developers can create more sophisticated AI applications that can generate images based on complex queries or use cases. The ability to seamlessly connect with company data sources, tools, and other APIs adds another dimension of power to these AI applications.
Recent developments within Bedrock have focused on enhancing both speed and ease of development. This trend emphasizes the importance of efficient development cycles without compromising the security and privacy of users and sensitive data. As e-commerce relies increasingly on AI for product imagery and customer engagement, the role of secure and efficient tools like Bedrock will become even more prominent. However, we should also acknowledge that the field is still evolving. It is important to monitor how these powerful tools are implemented to ensure they are used ethically and responsibly.
Amazon Bedrock's Metadata Filtering A Game-Changer for AI-Driven Product Image Generation - Text-to-Image Models Advance Product Visualization on lionvaplus.com
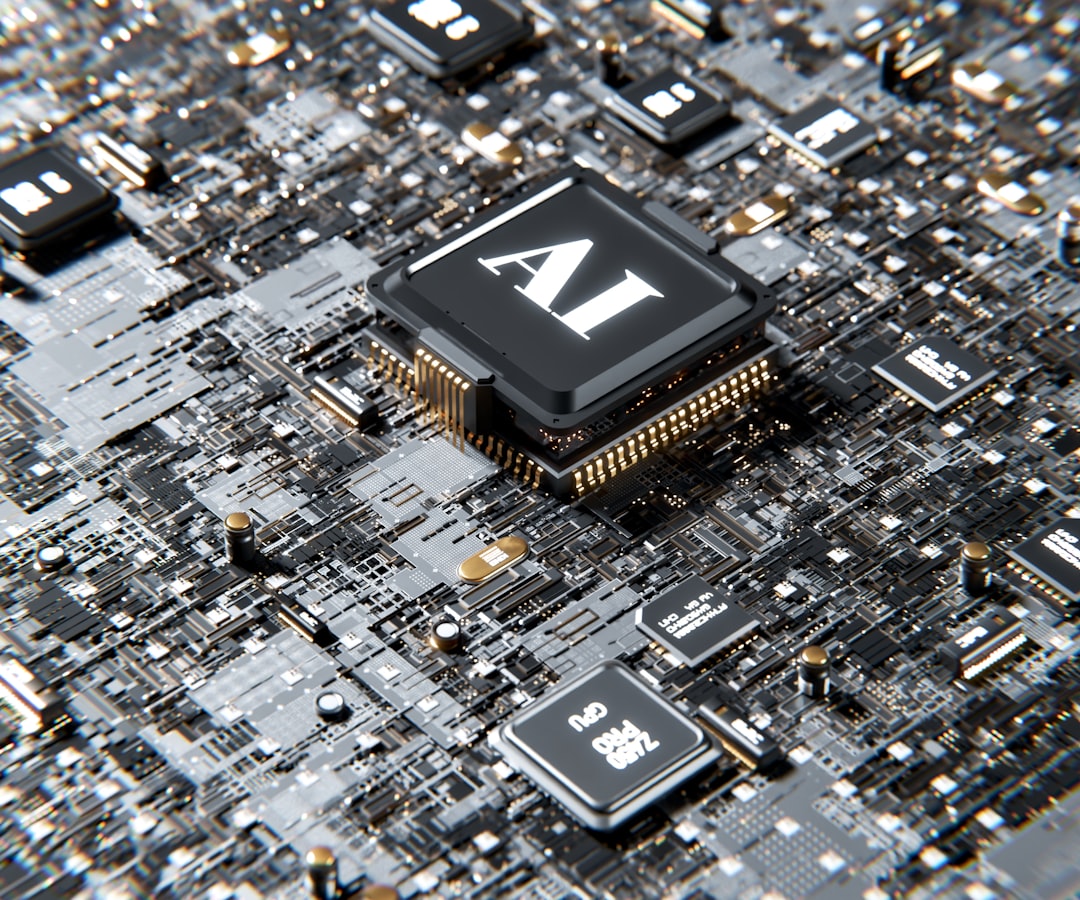
E-commerce sites like lionvaplus.com are seeing a transformation in product visualization thanks to text-to-image models. These AI systems can create realistic product images from simple text descriptions, opening up a world of possibilities for staging and showcasing items. The underlying technology, often based on large datasets and sophisticated algorithms like diffusion models, allows for a high degree of customization, letting businesses create images perfectly aligned with specific product details and marketing needs.
However, the technology isn't without its hurdles. Maintaining visual consistency across different generated images can be tricky. Similarly, ensuring the reliability and accuracy of the AI-generated images, especially in complex scenarios, is still a work in progress.
Moving forward, the effectiveness of these models is closely tied to the quality of the associated data. A well-defined metadata framework, providing rich information about products, is crucial to maximizing the potential of text-to-image models, leading to a better customer experience. The future of online product visualization might just depend on how well we can integrate these advanced image generation tools with structured, reliable information. This could ultimately revolutionize how products are marketed and experienced by online shoppers.
The field of AI image generation, particularly text-to-image models, is significantly impacting how product visualizations are created for online retail. These models, often built upon large language and vision foundation models, are trained on massive datasets and can produce images based on textual descriptions. This capability offers numerous potential advantages in e-commerce, particularly for generating visually appealing and relevant product images.
One of the key improvements is the **speed** of image creation. While traditional product photography can be a time-consuming process, text-to-image models can generate images in a matter of seconds, potentially saving businesses a significant amount of time and effort. Further, these models offer the ability to generate **customized images at scale**, tailoring them to specific target audiences or individual customers. This targeted approach to product visualization can enhance engagement and potentially lead to increased sales.
Beyond just creating images quickly, these models allow for **contextual accuracy**. The integration of rich metadata, for example, information on materials, size, color, and style, allows the models to generate images that are not only visually appealing but also highly specific to the product being showcased. This is important as consumers increasingly rely on visual cues to make purchasing decisions, so images that accurately represent the product's attributes become increasingly important. The success of this approach hinges on the **quality and diversity of the training datasets**. The more varied the training data, the better the model is likely to perform in terms of generating visually diverse and appealing images.
Interestingly, the ability to generate these high-quality images can also lead to a **reduction in return rates**. By accurately representing products in a variety of contexts, we might see fewer instances where a product doesn't meet consumer expectations leading to fewer returns, a costly aspect of online commerce. Additionally, the ability to incorporate **dynamic retrieval features** into image generation workflows offers businesses the ability to adapt their product visuals based on real-time market trends and customer preferences. This makes the images more relevant and increases their potential to grab the attention of potential buyers.
However, this is not a fully developed technology. We are still in the early stages of understanding the impact of these models. While some e-commerce platforms may have the ability to enable **user-driven image creation**, allowing customers to provide specific visual cues, this is still a feature in development. Similarly, integrating **multimodal inputs** into image generation, for example, using customer reviews or sentiment analysis, is a potential that is starting to be explored in the field.
Furthermore, these models offer the potential to **reduce common errors** found in traditional product photography like inconsistent lighting or poor product staging. Finally, AI-generated imagery can even create **entirely novel visual styles** that may diverge greatly from traditional photography. This is a valuable feature that could help businesses stand out in an increasingly crowded online market.
While the potential benefits of text-to-image models are clear, there are still some open questions. It will be important to continue to monitor these developments to see how they are used in practice and the long-term effects they may have on online commerce. It's clear that the future of product visualization in e-commerce is likely to be deeply influenced by AI image generation, with models like these becoming more sophisticated over time.
More Posts from lionvaplus.com: