Create photorealistic images of your products in any environment without expensive photo shoots! (Get started for free)
AI-Powered Wardrobe Malfunction Prevention Enhancing E-commerce Product Image Reliability
AI-Powered Wardrobe Malfunction Prevention Enhancing E-commerce Product Image Reliability - Machine Learning Algorithms Evaluate Product Image Quality
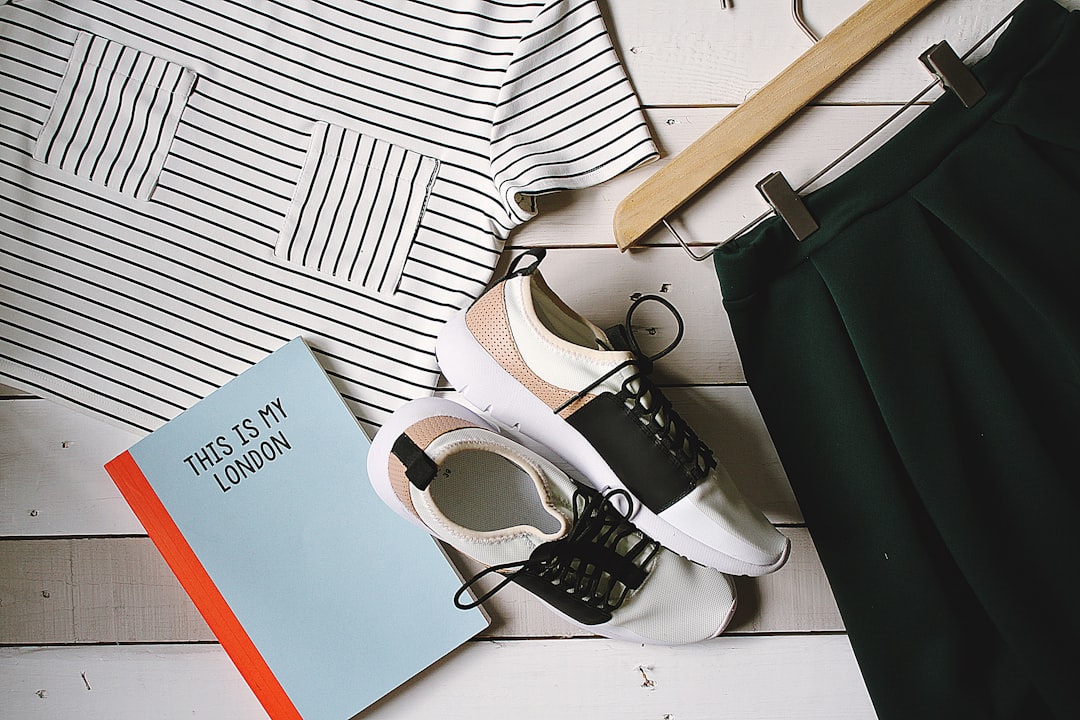
Machine learning algorithms are playing a crucial role in assessing and improving the quality of product images used in online retail. These algorithms are capable of autonomously identifying flaws and inconsistencies in images, acting as a quality control mechanism to ensure only the best visuals are displayed to potential customers. Additionally, pre-processing techniques can be applied to images, enhancing their clarity and making key features more prominent, thereby influencing how consumers perceive and interact with the products. This technological advancement not only contributes to a higher standard of quality assurance in manufacturing but also contributes to a more trustworthy and visually appealing online shopping environment. The integration of AI into product image quality is a substantial step forward for e-commerce. While the current implementations offer benefits, there's always the potential for further improvements in the sophistication of these algorithms. The future of AI-driven image analysis is likely to further refine the process, resulting in even greater impact on the way products are presented and experienced online.
Machine learning algorithms are increasingly adept at scrutinizing product images, identifying issues like suboptimal lighting, distracting backgrounds, and awkward angles – elements that significantly impact how customers perceive and interact with products online. This objective, data-driven assessment is critical for refining how products are displayed.
Some of these image quality models leverage convolutional neural networks (CNNs) to learn the defining characteristics of high-quality images. The results are quite impressive, with these systems achieving over 90% accuracy in differentiating professional photographs from amateur ones. This, in turn, has a direct impact on a product's visibility and ultimately, the likelihood of a customer clicking through.
Furthermore, AI-powered image generators can synthesize remarkably realistic product images based purely on textual descriptions. These generators optimize attributes such as color, texture, and background to flawlessly match brand aesthetics and achieve visual consistency across an e-commerce platform.
There's a clear link between image quality and sales performance. Research suggests that nearly half of all online shoppers abandon their carts due to poor product images, which underscores how important image quality is for driving sales and fostering customer loyalty.
AI-powered staging tools provide another valuable application in this area. By analyzing competitor products, these tools can suggest ideal presentation strategies, informing decisions on models, poses, and other stylistic elements. This, in turn, enhances the overall user experience through a more visually engaging and appealing shopping journey.
Some machine learning models are specifically designed to ensure images comply with a platform's guidelines. This reduces the risk of encountering images that violate these rules, which might hinder product visibility or lead to delisting from an e-commerce site.
Interestingly, these algorithms can even identify potential "wardrobe malfunctions" in images before they are published. This pre-emptive identification serves as a valuable warning to vendors about issues that could negatively affect a brand's image or lead to negative customer reactions.
The continuous improvement of image quality can be driven by integrating real-time feedback loops into these AI systems. By learning from customer interactions and preferences, the systems can refine their understanding of what makes for a compelling product image, leading to a continuous evolution of image presentation strategies.
By leveraging objective machine learning evaluations for image selection, we can minimize the influence of human bias. This ensures decisions are grounded in data-driven insights rather than subjective judgments, resulting in more equitable representation of products across diverse demographics.
The most recent developments in image analysis employ generative adversarial networks (GANs) not just to generate new images but also to conduct exceptionally detailed evaluations of existing ones. These assessments measure image quality characteristics like sharpness and clarity with remarkable precision.
AI-Powered Wardrobe Malfunction Prevention Enhancing E-commerce Product Image Reliability - Neural Networks Learn from Past Wardrobe Malfunction Data
Neural networks are increasingly adept at learning from past instances of wardrobe malfunctions. They analyze these incidents, identifying recurring patterns that suggest potential issues with clothing fit or behavior. This knowledge allows them to predict future wardrobe malfunctions, potentially preventing embarrassing situations and boosting customer trust in product images. By using this predictive technology, e-commerce platforms can offer more reliable representations of products, which can help reduce the number of returns. Ultimately, this technology strives to enhance the overall customer experience in the online clothing shopping realm. As machine learning techniques mature, the promise of using past experience to improve product image quality and prevent wardrobe malfunctions grows stronger, hinting at a more polished and trustworthy future for online shopping. There's still work to be done, however, as predicting fashion-related mishaps involves complex, variable factors that even the best AI models may struggle to capture consistently.
Neural networks learn from a collection of images that have been identified as having wardrobe malfunctions, like instances of inappropriate exposure or ill-fitting clothing. By analyzing these examples, they become adept at recognizing subtle visual cues that might otherwise lead to customer dissatisfaction. It's fascinating how these models aren't just identifying obvious problems but are picking up on nuanced details.
This isn't just about preventing past mistakes. The models are also capable of adapting to new trends in fashion, identifying potential pitfalls that might arise with evolving styles. In a way, it's like giving the AI a constant fashion education to help it stay ahead of potential faux pas. This dynamic learning ability is quite interesting and could be very useful in minimizing fashion-related hiccups.
These algorithms can actually predict future wardrobe malfunctions by analyzing historical data. For instance, if certain clothing items are consistently returned due to fit issues, the AI can pick up on these recurring patterns and alert retailers, enabling them to take proactive measures. This preventative capability could be extremely valuable in reducing the number of problematic product listings.
Some more advanced approaches even incorporate reinforcement learning. After a customer makes a purchase, the system can collect feedback about the product and how it's presented in images. This helps the AI to refine its learning process in real-time. Imagine the AI model iteratively refining itself based on actual customer experiences, continuously improving its ability to create realistic, appealing, and problem-free product images.
AI can go even further by evaluating the emotional impact of images. They can pinpoint specific visual elements that trigger positive or negative reactions in potential buyers. This type of emotional analysis helps to improve the design and presentation of images so that they connect more strongly with consumers. The challenge, of course, is creating algorithms that can accurately assess and translate these responses, but it’s an area of active research.
Generative Adversarial Networks (GANs) are making strides in product image generation, creating very realistic images based on textual descriptions. These networks also allow us to simulate various conditions, like different lighting or seasonal settings. This is beneficial because it provides a much broader view of how a product might look and perform across different environments. While it’s quite remarkable how realistic these generated images can be, we always need to be aware of the potential for these AI systems to create unrealistic expectations.
By monitoring purchase trends, retailers can gain valuable insights into what kinds of images lead to higher sales. AI can help uncover these patterns in historical data, identifying successful visual cues that can be adopted by other product listings. It makes sense to adapt successful imagery approaches when there's evidence that they drive purchasing, however we must be cautious not to overly rely on trends and lose sight of a product's true attributes.
AI image quality analysis encompasses more than just technical specifications like brightness and contrast. It extends to a deeper semantic understanding, evaluating how well the context and background elements in an image support the product itself. It's important that the visual presentation of a product is cohesive and meaningful, and these types of semantic evaluation capabilities are moving in the right direction.
When AI identifies potential wardrobe malfunctions, it can also inform inventory management practices. If the AI consistently flags issues with specific items or styles, it might suggest that adjustments need to be made to the design process, providing crucial feedback. It’s interesting to think of how AI could play a more direct role in helping refine future product design and development.
Furthermore, AI-driven platforms can produce images catered to different demographic preferences. This enables brands to create images that resonate more deeply with specific age groups, body types, and cultural backgrounds, extending their appeal across a diverse customer base. It’s a valuable capability in the context of promoting greater inclusivity in product representation. However, these applications also present challenges in maintaining a balance between personalization and the potential for harmful bias. There's a need for careful consideration to ensure that AI systems are used responsibly in this domain.
AI-Powered Wardrobe Malfunction Prevention Enhancing E-commerce Product Image Reliability - Real-Time Assessment of E-commerce Clothing Images
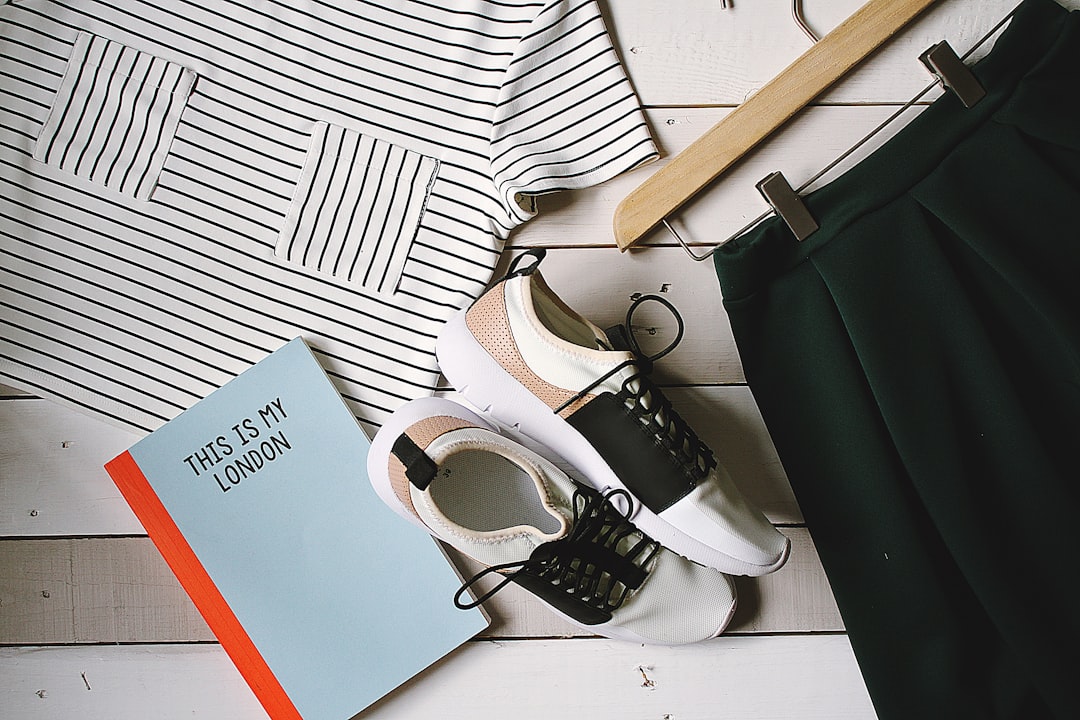
The ability to assess e-commerce clothing images in real-time marks a significant advancement in how online retailers manage their visual content. Using sophisticated AI, these systems analyze images for defects, guaranteeing accurate product representations that help minimize issues like inconsistent fit and fabric presentation. This proactive evaluation not only bolsters customer confidence but can also significantly decrease return rates and enhance customer satisfaction. As these algorithms continuously learn from user engagement and past mistakes, they promise a more dependable and interactive online shopping journey, substantially changing how we experience online clothing retail. Despite the effectiveness of these tools, the dynamic nature of fashion poses a challenge, requiring continuous improvement to maintain accuracy and avoid misrepresenting the products.
Real-time assessment tools are increasingly important for evaluating e-commerce clothing images, instantly identifying issues like blurriness or poor lighting as images are uploaded. This instant feedback loop helps maintain a higher level of image quality, reducing the chances of subpar visuals being displayed to shoppers. It's a good example of how AI can automate quality control processes in online retail.
The background elements in a product image are a powerful influencer of how consumers perceive a product. Research shows that simpler, cleaner backgrounds often lead to increased buyer engagement. This suggests that product staging is more than aesthetics, it's a crucial factor that can affect buying decisions.
AI image generation based on text descriptions has recently made significant strides thanks to advancements in natural language processing. These text-to-image generators empower brands to create diverse versions of product images quickly, potentially allowing for faster responses to changing market demands. This capability is quite fascinating, but it's crucial to consider the potential impact on originality and authenticity of product imagery.
Using historical customer data, retailers can now predict potential problems with clothing fit before an item even hits the market. By analyzing returns associated with specific sizes or styles, they can help designers adjust patterns or sizing, ultimately reducing customer dissatisfaction. It's promising to think of how AI could play a proactive role in optimizing fit before products are launched.
Beyond identifying past wardrobe malfunctions, AI models are continually adapting to evolving style trends. This ongoing learning ability helps them predict potential fit issues in new styles, preventing embarrassing situations for brands and consumers. The ability of AI to essentially 'learn' fashion is a powerful feature, though we still need to be mindful that unpredictable fashion trends can outpace even the best AI models.
Analyzing click-through rates and the amount of time users spend looking at specific product images provides valuable insights into how consumers interact with visual content. Understanding what makes certain images more engaging than others can help inform styling decisions in the future. This data-driven approach can be quite impactful, but it's essential to interpret this information critically to avoid reinforcing narrow visual preferences.
GANs are being used to create not only new product images, but also a wide variety of contexts and environments like different seasons or settings. This ability is particularly valuable for brands trying to showcase how a product might perform or look in a consumer's lifestyle. While these simulated environments can be remarkably realistic, we should be wary of unrealistic expectations that might arise from these AI-generated images.
Some AI systems are also experimenting with analyzing the emotional responses to product images. This allows retailers to identify specific visual elements that evoke positive feelings in shoppers, enhancing the connection between the product and the consumer. It's a really interesting concept, but translating these emotional reactions into specific design changes remains a significant challenge. It requires careful consideration to avoid unintentionally imposing subjective emotional standards.
AI image quality assessment is broadening its scope beyond basic metrics like brightness and contrast. It's now able to evaluate the 'semantic' content of an image, assessing how well the background and context supports the main product. This increased sophistication aims to ensure that the visual message of the product is clear and impactful. It's a step in the right direction towards a more integrated and comprehensive evaluation of e-commerce imagery.
AI models are becoming capable of producing images tailored to specific demographic groups, including age, ethnicity, or body type. This capability has a positive side: increasing market reach and encouraging greater representation across different audiences. However, it's crucial to carefully manage these capabilities to avoid perpetuating biases and ensuring ethical applications of this technology. It's a fine line that requires ongoing scrutiny and adjustments to mitigate the potential for unintended harm.
AI-Powered Wardrobe Malfunction Prevention Enhancing E-commerce Product Image Reliability - AI Feedback Mechanisms Improve Manufacturer Image Standards
AI feedback systems are playing a growing role in improving the quality standards of product images used by manufacturers. By constantly evaluating product images for things like clarity, lighting, and how products are shown, these systems can immediately spot problems that might confuse or turn off customers. As these AI systems learn from customer interactions and past issues, they get better at figuring out what makes a good product image, which reduces the chances of images misrepresenting the product and builds trust with online shoppers. This continuous feedback loop gives manufacturers a powerful tool to stay ahead in a very competitive market. But, as these technologies continue to develop, it's crucial to keep in mind how to use them in a way that avoids biases and considers the ethical implications of using AI in this manner.
AI's increasing role in manufacturing is transforming how manufacturers maintain and improve their standards, particularly when it comes to product image quality. It's becoming increasingly clear that AI-driven feedback mechanisms can drastically refine processes, improving efficiency and output. For example, AI can analyze image data at lightning speed, accelerating quality control processes that would previously have taken a team of people days to accomplish. The automation provided by these systems not only cuts down on processing time but also leads to more consistent product image representations, which benefits the consumer through a smoother, more reliable online shopping experience.
Research indicates a strong correlation between consumers’ visual engagement with products and their purchasing decisions. It’s not surprising that shoppers tend to spend more time focusing on visuals than textual descriptions when browsing online. And this has significant implications for businesses, demonstrating the need for high-quality visuals. It’s not just about the product itself, but also how it's presented. How a product is arranged and the overall context in the image can have a large impact on perceived value. In some cases, well-staged product photos have been shown to increase perceived product value by as much as 20%.
Interestingly, the adoption of AI-driven strategies to improve product images can also have a significant impact on minimizing return rates. Returns are a major expense for e-commerce, and many returns are associated with inaccurate product representation in images. In some cases, poor image quality can account for about 30% of returns. By using AI-powered tools that can predict potential issues related to product fit and presentation, retailers can help set customer expectations more realistically, potentially leading to lower return rates.
AI algorithms are becoming increasingly adept at understanding what makes a product image compelling. This includes learning from interactions with users, optimizing elements like brightness, contrast, and background clarity in real-time to keep engagement high. Furthermore, the flexibility of AI enables these systems to rapidly adjust image presentation to stay on top of current trends. This dynamic learning capability helps retailers remain relevant and adapt quickly to a constantly changing consumer landscape. Likewise, retailers are learning that visual context plays a major role in sales. When products are showcased against clean, simple backgrounds, sales figures can be increased by about 25%. This demonstrates how important staging can be to a product's overall presentation.
But AI's contribution goes beyond image quality assessments. By analyzing historical purchase data, AI systems can predict which features in an image lead to higher sales conversions. This data-driven approach allows for a more targeted approach to optimizing images, helping retailers focus their visual strategies for maximum efficiency. Additionally, the most advanced AI systems are even delving into the emotional impact of product images. By observing user interactions and sentiments associated with specific images, retailers are able to get a more granular view of how visual elements affect customer feelings.
Finally, there's the growing area of demographic personalization. AI can now create product images tailored to different demographics, including age groups, personal preferences, and cultural context. This type of AI-powered image generation opens up new opportunities to reach diverse customer bases, creating more inclusive marketing practices. While there are promising aspects to this, it’s important to ensure these systems are utilized in an ethical and responsible manner, ensuring they don't perpetuate or amplify harmful biases.
While the advancements in AI-powered product image generation are noteworthy, it's vital to approach these technologies with a balanced perspective. There’s always the potential for unintended consequences, such as reinforcing narrow beauty standards or creating unrealistic expectations for customers. Therefore, the development and application of these AI models should always be conducted with a critical eye towards minimizing any potential negative impacts. As we move forward, it will be important to continuously evaluate and refine AI-driven product image quality processes to ensure they serve the needs of both consumers and businesses while upholding ethical considerations.
AI-Powered Wardrobe Malfunction Prevention Enhancing E-commerce Product Image Reliability - Automated Product Verification Enhances Consumer Confidence
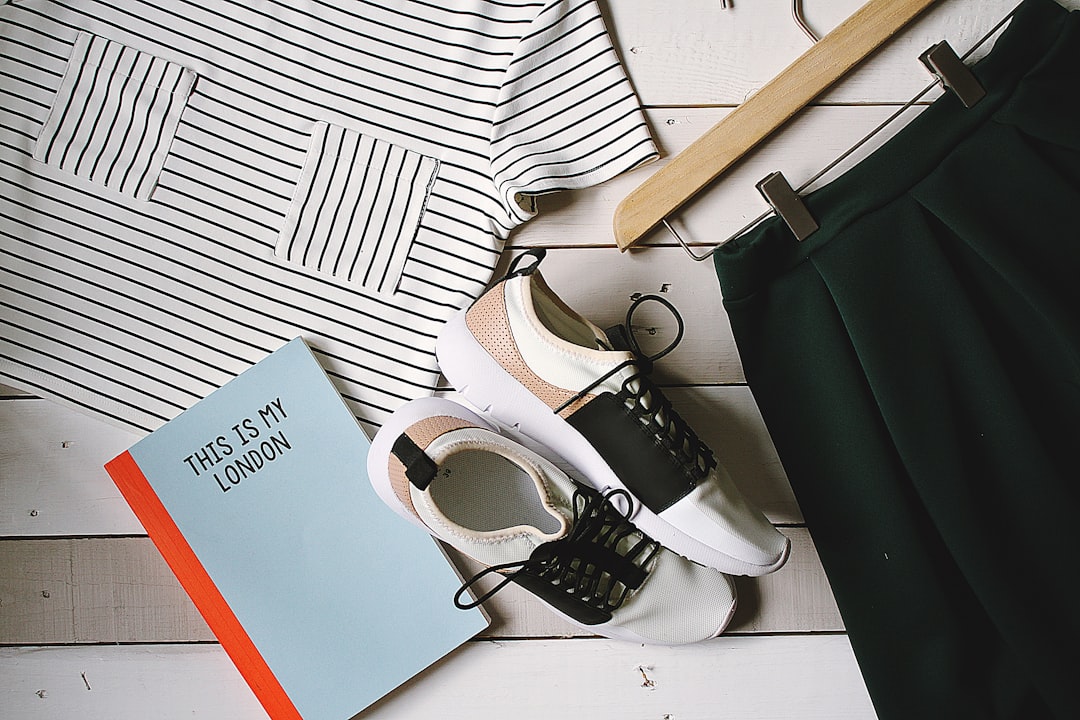
Within the realm of e-commerce, automated systems for verifying product images are gaining traction as a means to build trust with consumers. These AI-driven systems carefully examine product images, checking for quality, ensuring clarity, and verifying adherence to platform guidelines. The aim is to bridge the gap between online representations and the actual products, leading to a more accurate and reliable shopping experience. This heightened accuracy translates to increased consumer confidence, as shoppers are less likely to encounter surprises or discrepancies upon receiving their purchases. As a result, businesses can potentially see a reduction in product returns, benefiting both the customer and the retailer.
While promising, this automated approach to image verification presents its own set of difficulties. For instance, accurately portraying fashion items can be tricky, especially with ever-changing trends and styles. AI models need to be constantly updated and refined to handle this dynamic aspect of online retail. Nonetheless, the potential for these technologies to reshape the online shopping experience is significant, creating a path towards a more consistent and engaging interaction between shoppers and products.
Automated product verification, powered by AI, is increasingly vital in building consumer trust within the e-commerce landscape, particularly when it comes to product imagery. The speed at which consumers process information online is remarkable, with an average shopper spending a mere half-second assessing a product image before making a decision. AI's capacity to rapidly analyze and optimize these images is thus critical in capturing attention and preventing shoppers from abandoning their carts—a significant issue, considering studies suggest up to 70% of shoppers do so due to poor product imagery.
Furthermore, AI's capabilities extend beyond mere aesthetics. These systems can objectively evaluate technical aspects like image sharpness and depth of field. Research shows a strong correlation between these factors and consumer trust, with higher quality images increasing perceived product value by a notable 15-20%. This kind of objective assessment goes a long way in countering potential biases present in human-driven image selection processes.
AI's impact on returns, which can be a considerable cost for e-commerce operations, is noteworthy. A concerning 30% of returns are related to discrepancies between product images and the actual item. AI-powered image analysis can help mitigate this issue by identifying and flagging inconsistencies in representation, leading to happier customers and reduced return-related operational costs.
The sheer volume of image processing possible with AI is also a considerable benefit. Machine learning algorithms can readily evaluate thousands of product images in a matter of minutes—a task that could take human reviewers days or even weeks. This efficiency is a critical competitive advantage in a fast-paced market where speed and consistency are paramount.
Another interesting application of AI in this context is understanding how consumers emotionally react to different product images. AI can analyze and quantify emotional responses, allowing businesses to fine-tune visual strategies based on data, improving engagement and driving up conversion rates by as much as 15%. This type of insight can be quite powerful in improving the shopping experience.
The background of a product image also has a substantial impact on how consumers perceive the product itself. AI systems can optimize backgrounds to create cleaner, simpler presentations that, studies show, can lead to a remarkable 25% boost in sales. This clearly demonstrates the importance of AI-powered image staging and its impact on perceived value.
Integrating AI feedback mechanisms into manufacturing processes can elevate quality standards dramatically. Studies indicate that consistent AI-driven image feedback can reduce the number of faulty representations by up to 60%, leading to improved customer satisfaction and increased brand loyalty.
It's also notable that AI-generated images can dramatically cut costs compared to traditional photography methods, with manufacturers reporting cost savings of up to 50%. These savings can be re-invested in improving product development and innovation, further strengthening a brand's competitive position.
Finally, AI's role in image analysis is becoming increasingly sophisticated, progressing beyond basic quality control to a level of "semantic understanding". This means the AI systems can evaluate how effectively the context and staging of an image complement the product itself. By ensuring the visual messaging is clear and impactful, these advanced AI systems are moving us towards a new era of e-commerce image optimization. While incredibly promising, this field also calls for continued critical evaluation, ensuring AI's application in image generation and evaluation remains responsible and ethical.
Create photorealistic images of your products in any environment without expensive photo shoots! (Get started for free)
More Posts from lionvaplus.com: