AI-Enhanced Product Photography Recreating Vintage Matchbox Design Aesthetics for E-commerce
AI-Enhanced Product Photography Recreating Vintage Matchbox Design Aesthetics for E-commerce - Training AI Models With Original 1960s Matchbox Car Photography Archives
Using original 1960s Matchbox car photos to train AI models allows them to grasp the specific visual language of that era. This approach is crucial for producing AI-generated product images that capture the authentic look and feel of vintage designs. By learning from these historical photo archives, AI systems become better equipped to generate images that appeal to the desire for retro aesthetics, a growing trend in online marketplaces. This merging of vintage photography with modern AI image generation offers a unique path for presenting products. It also raises the need to carefully consider how to incorporate the stylistic nuances of the past while using today's techniques to stage products compellingly. Ecommerce platforms now have a chance to merge the best of both worlds, presenting a nostalgic experience while utilizing fresh approaches to visual merchandising. This combination, if done thoughtfully, can be appealing to consumers who enjoy a blend of retro charm and contemporary shopping experiences.
Leveraging original 1960s Matchbox car photography archives provides a rich dataset for training AI models to understand and recreate vintage aesthetics. These archives showcase the characteristic color palettes of that era, including pastel tones and retro styles that heavily influenced vintage toy marketing. This knowledge can be incredibly useful for modern e-commerce strategies targeting a nostalgic consumer base.
Furthermore, studying the historical context of these photographs, including prevalent lighting and staging techniques of the 1960s, allows AI models to replicate these stylistic elements with greater authenticity. The simple, minimalist product staging often used with Matchbox cars can guide AI image generators toward creating clean, visually focused images. This simplicity can potentially enhance clarity and visual impact for e-commerce platforms.
AI model training necessitates considering the historical context of consumer behavior as well. By analyzing these archives, AI can predict the aesthetics that resonate with certain market segments, leading to more effective and targeted product images. Exploring the principles of computational design used in reconstructing vintage aesthetics also offers valuable insights for AI, allowing it to replicate variations in staging prevalent in the 1960s, improving virtual merchandising strategies.
Interestingly, the unique depth-of-field techniques used in 1960s Matchbox photography—highlighting the car while subtly blurring the background—can be replicated by AI to increase user engagement with e-commerce listings. Analyzing the composition techniques used in these photographs can also guide e-commerce businesses in choosing framing and angles that best appeal to buyers who appreciate the historical visual language.
By incorporating features of these vintage photos, AI can create more accurate virtual simulations of product usage scenarios. This enhances a buyer's understanding of how the product functions in real-world contexts. Additionally, the characteristic grain and lower resolution of original photographs often possess a unique texture lacking in modern images. Incorporating these features in AI-generated photos can add a sense of authenticity and nostalgia that might resonate with collectors. Finally, the specific cultural references present in Matchbox car imagery, such as themes and trends of the era, can be harnessed to create more compelling narratives surrounding products. This contextual enrichment can greatly enhance the buying experience for consumers seeking a strong historical connection.
AI-Enhanced Product Photography Recreating Vintage Matchbox Design Aesthetics for E-commerce - Automated Background Generation Using Period Accurate Props and Settings
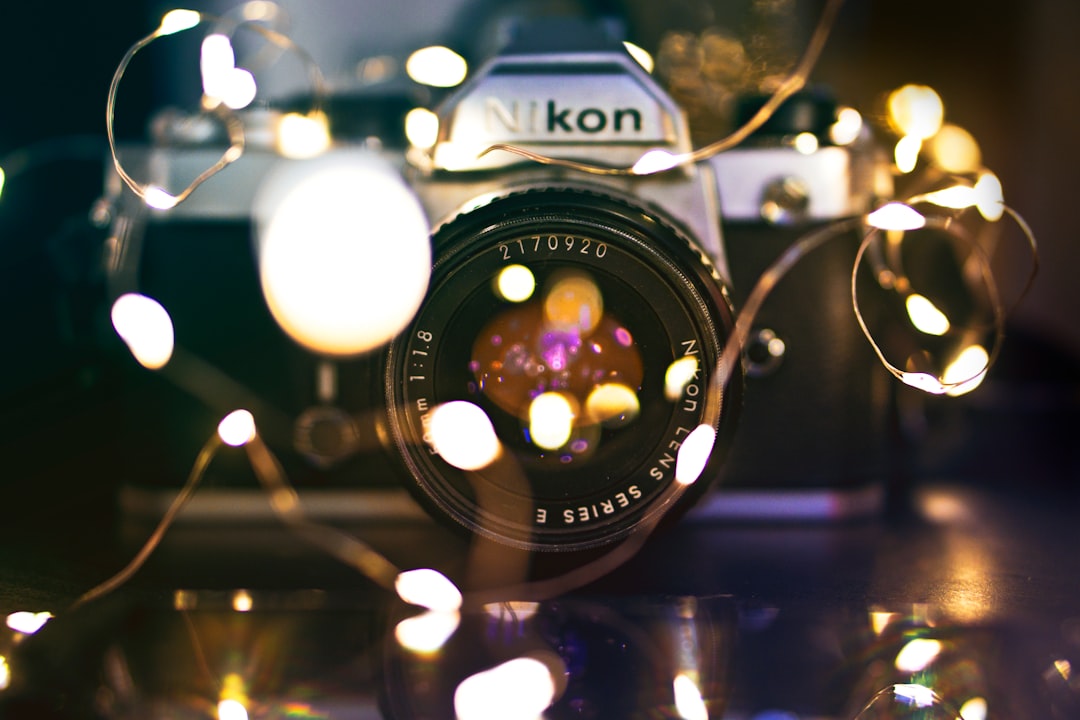
Automating the creation of backgrounds for product photography is now possible using AI, allowing e-commerce to incorporate period-accurate props and settings. This technology can be incredibly helpful when trying to recreate a specific aesthetic, especially for vintage-inspired product lines, such as the revival of 1960s toy designs. The algorithms within these tools are sophisticated enough to produce realistic backgrounds that enhance the overall presentation of the product. One key aspect is the ability to analyze product images and intelligently suggest appropriate background options, leading to more harmonious and engaging product visuals. Essentially, e-commerce platforms can now present product listings in a way that feels both modern and historically authentic, potentially appealing to a wider range of buyers. Importantly, the tools themselves are often very user-friendly, making it feasible for even less experienced individuals to create professional-quality images for their online shops. While this automation brings clear benefits, it also raises questions about authenticity, particularly when recreating historical aesthetics in a contemporary context. The challenge lies in ensuring that the AI-generated visuals remain true to the original aesthetic while also enhancing the product’s appeal within the current marketplace.
Automating the creation of product backgrounds using period-accurate props and settings within AI-generated imagery is a fascinating area of study. By meticulously analyzing original vintage photographs, specifically those from the 1960s Matchbox car era, AI systems can learn to recreate the unique stylistic elements of those times. This ability to digitally reconstruct the past is quite remarkable. We're talking about the subtle nuances of light, shadow, and composition that were common in those photos. AI can essentially deconstruct those elements, allowing us to see how seemingly small variations in these visual aspects impacted consumer perceptions back then.
This reconstruction is not just about aesthetics, though. Studying past consumer behavior patterns is invaluable. By analyzing what worked visually in the 1960s, we can potentially develop insights that lead to more successful visual merchandising strategies in contemporary e-commerce. For example, the specific techniques for creating depth of field in older photos—where the subject is in focus while the background is subtly blurred—can be precisely replicated by AI. This enhances user engagement and draws attention to the product itself. It's intriguing how technical details can spark memories and create a sense of nostalgia, particularly for niche audiences like collectors.
It's also interesting how AI can dynamically weave in contextual details within the backgrounds it generates. Imagine recreating a 1960s scene with seasonal elements or incorporating cultural references specific to that era. It offers a way to tell visual stories about products, potentially strengthening the emotional connection a customer feels. And there's a certain charm to the lower resolution and film grain seen in those old photographs—elements that modern, crystal-clear images often lack. The AI has the potential to emulate these textural qualities, further enhancing authenticity.
Another intriguing aspect is the possibility of juxtaposing historical and contemporary visual styles in the same product image. This could be beneficial for showing product evolution or simply for allowing consumers to compare different aesthetic preferences.
Of course, one of the main benefits of automated background generation is the potential for consistency and efficiency. It can ensure that every image within a product line has a uniform look and feel, creating a strong brand identity. It's also a considerable time-saver compared to traditional product photography. It is exciting to explore how AI can subtly influence a customer's decision-making process by evoking nostalgia and recreating effective advertising strategies from the past. In short, the ability to create these hyper-realistic, vintage-inspired backgrounds could become a valuable tool for enhancing product presentation in online marketplaces. While still relatively new, the potential for its impact on consumer engagement and product perception is quite promising.
AI-Enhanced Product Photography Recreating Vintage Matchbox Design Aesthetics for E-commerce - Lighting Simulation Tools That Recreate Mid Century Film Stock Effects
Tools that simulate lighting are now capable of recreating the distinctive visual characteristics of mid-20th century film stock, offering photographers and filmmakers a powerful way to achieve vintage aesthetics. This is especially useful for recreating the unique look and feel of vintage product photography from the 1960s and 70s, as seen in areas like toy advertising. These tools provide a virtual environment where users can design complex lighting setups before an actual shoot, improving efficiency and offering greater control over the final look. It's interesting how AI-driven lighting techniques are now being incorporated into these tools, allowing for more precise recreations of the subtle lighting effects that contributed to the overall feel of vintage images. E-commerce businesses seeking a retro aesthetic can leverage these tools to present products in a manner that appeals to a nostalgia-driven consumer base. While the potential benefits for creating a truly vintage aesthetic are promising, there's also the challenge of ensuring the AI-generated lighting styles stay authentic to the original source materials. This aspect becomes especially important for businesses striving to reimagine historical aesthetics in a modern commercial context. The blending of vintage aesthetic sensibilities with contemporary digital imaging technologies is a growing trend that is bound to shape how product imagery is created and presented.
Tools designed for lighting simulation are becoming increasingly adept at recreating the distinctive visual characteristics of mid-20th-century film stock. This capability is quite valuable for anyone trying to achieve a vintage aesthetic, particularly in the realm of product photography for e-commerce. For example, we can now digitally replicate the unique color palettes and tones of vintage film, which are often associated with a sense of nostalgia. These tools offer granular control over lighting, allowing us to recreate the soft, warm light that was typical of that era.
One interesting application is the simulation of bokeh and depth of field, techniques commonly used in vintage product photography to highlight the main subject while softening the background. This approach can be quite effective in drawing a viewer's attention to the key elements of a product image, something crucial for online marketplaces. Furthermore, these simulation tools can even generate the distinctive grain and texture of vintage film, adding a subtle visual warmth and character that can enhance a product's perceived authenticity. The technology has advanced to a point where it can incorporate specific lighting techniques from the past, such as the use of diffused light, thus reducing the need for extensive post-production work.
It's fascinating how some of these tools go beyond just manipulating light and color. Some can even take the surrounding environment into account, ensuring a product image looks consistent with the broader historical context it's trying to emulate. Moreover, a few offer interactive features, allowing designers to experiment in real-time with different lighting setups and see the immediate effect on the final image. It's essentially a virtual photography studio within a software program. Interestingly, some advanced algorithms can even learn from historical consumer behavior, helping predict what types of lighting effects are most likely to resonate with different audience segments, potentially improving marketing campaigns.
Beyond simply generating visually appealing images, these simulation tools allow us to explore the interplay between lighting, color, and narrative. We can craft a better visual story that potentially resonates more strongly with buyers who appreciate the charm of yesteryear. Some can even simulate the characteristics of particular vintage cameras and lenses, allowing us to refine the aesthetic further. The evolving landscape of digital tools and AI in this field is quite exciting. It gives us new creative avenues to present products, particularly when trying to tap into the appeal of nostalgia and vintage aesthetics. While the tools themselves are evolving, they raise intriguing questions about authenticity and our relationship with historical visual language in a world dominated by digital imagery.
AI-Enhanced Product Photography Recreating Vintage Matchbox Design Aesthetics for E-commerce - Machine Learning Algorithms That Analyze Vintage Product Package Design Elements
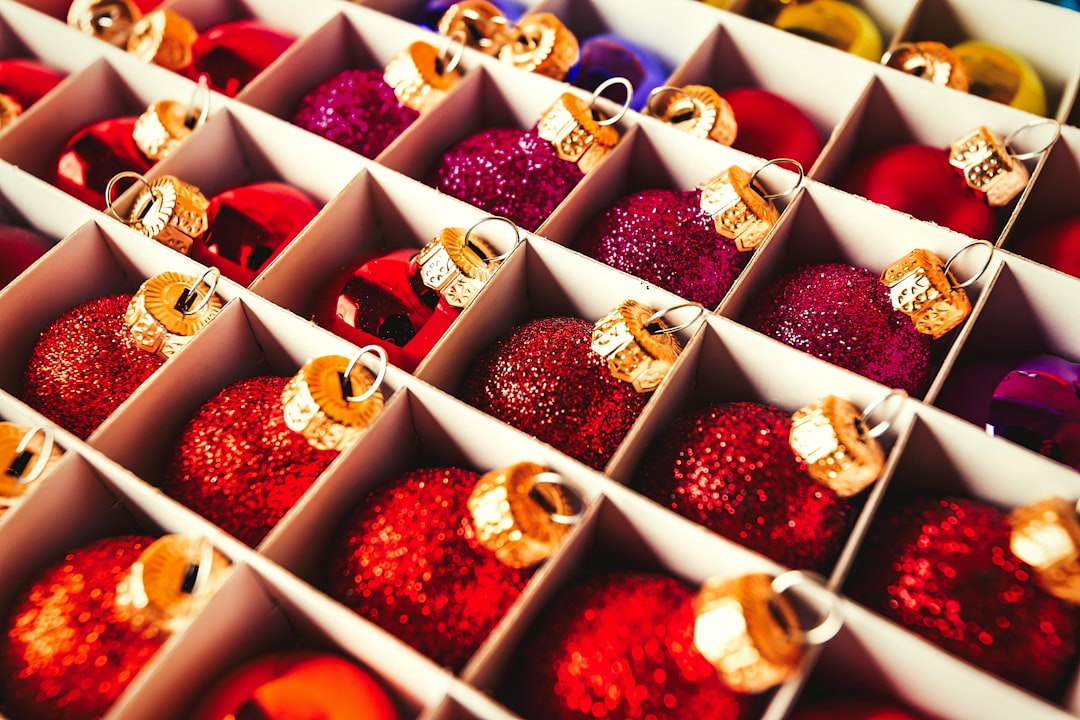
Machine learning algorithms are increasingly being used to analyze the design elements found in vintage product packaging, particularly within the realm of e-commerce. These algorithms, relying on methods like pattern recognition and deep learning, can analyze huge numbers of vintage advertisements to identify popular design features. By understanding these past trends, AI can suggest design choices, such as layout, color palettes, and images, that are likely to appeal to today's consumers who appreciate a vintage look. This approach blends AI with design, making it possible for businesses to quickly test out and refine product designs in ways that maintain a genuine connection to historical styles while also looking appealing to current buyers. The use of AI in this area, while offering advantages, does create questions about the authenticity and emotional impact of recreating vintage aesthetics using digital tools. This is especially important for businesses hoping to leverage a nostalgic feel within their e-commerce strategy.
Machine learning algorithms, particularly those focused on pattern recognition and deep learning, are capable of sifting through vast collections of vintage product packaging. This allows them to pick out recurring design elements and styles that contributed to the overall aesthetic of a specific era. For instance, they can recognize the typical color palettes used, such as the muted pastels common in the 1960s, and identify these features as potential keys to generating images with a nostalgic feel for e-commerce.
These algorithms aren't just analyzing colors. By studying the historical context of the designs—considering social and cultural factors that influenced aesthetics—AI models can develop a better understanding of how to create imagery that resonates with a target audience. This means not just copying visual styles, but also capturing the spirit of the era in the images. For example, they might be able to generate product shots that evoke the carefree optimism of the 1960s through both design elements and the broader visual context of the image.
One of the interesting aspects of these machine learning methods is their ability to mimic the composition techniques used in vintage photography. This includes things like breaking traditional symmetry, utilizing focal points, and manipulating visual space in ways that catch the eye. By emulating these, AI could help create product listings that stand out more on online platforms.
Furthermore, these systems are very good at pulling out specific features from a large set of vintage images. This means that they can identify subtle things, like certain typography or graphic styles, that defined vintage aesthetics. The amount of data that can be processed is considerable, and the details they pick out are more than just surface-level.
The potential to simulate film grain and textures from old photography is another aspect worth noting. This can add a certain depth and authenticity to digital images, giving them a sense of nostalgia that might influence buyers' perceptions.
Linking consumer behavior data with insights from historical designs is also a powerful application. Machine learning models can start to predict which vintage design elements are more likely to grab the attention of particular customer groups, enabling more focused and effective product visuals.
Along those same lines, the ability to digitally recreate vintage environments and settings is a fascinating application. The AI could be trained to generate backgrounds that fit the style of a particular era, providing an added layer of authenticity and narrative around the product.
One practical outcome of these methods is the potential for automated testing of different design options. A system could be built to automatically create versions with slightly different elements, track how users respond to them, and refine the design accordingly to maximize its appeal to a nostalgic audience.
Looking forward, these techniques could help companies create product images that appeal to a wider range of audiences. By analyzing design trends across different generations, the potential exists to find new ways to connect with both those who long for a vintage experience and younger audiences seeking a unique or classic aesthetic. There's a challenge in ensuring these AI-generated visuals don't become just imitations of the past but are carefully used to create truly compelling visuals for today's shoppers.
AI-Enhanced Product Photography Recreating Vintage Matchbox Design Aesthetics for E-commerce - AI-Powered Color Grading Systems That Match Historical Print Techniques
AI is transforming product photography in e-commerce, particularly in achieving the distinctive color palettes of vintage print techniques. Tools like Colourlab AI and Match AI are examples of systems that can automatically match and balance colors, effectively simplifying the color grading process for photographers of all levels. This opens up new possibilities for e-commerce businesses to give their product images a retro feel, aiming to capture the charm and aesthetic of past eras. The ability to recreate these specific color profiles is an advantage, but it also highlights a key question: how can we use these AI tools to stay faithful to the vintage aesthetic while still making the products visually compelling in today's online marketplaces? There's a fine line between leveraging these powerful tools and potentially diluting the very unique qualities that define vintage visual styles. It's an important aspect for e-commerce to carefully consider if they want to genuinely capture the attention of consumers who value this style.
AI systems are becoming increasingly sophisticated in their ability to mimic the look and feel of historical print techniques for e-commerce product images. It's fascinating how these systems can replicate the chemical processes of older methods like lithography or screen printing, creating a sense of authenticity in images that might resonate with those who appreciate vintage aesthetics. Interestingly, the algorithms in these color grading programs are not just focused on the color itself but also on aspects like grain and texture. They can analyze the subtle variations in older photographs and then recreate them in the digital images, giving them a more textured look, a quality sometimes missing in overly crisp modern photos.
Further, there is a growing focus on how these tools can help understand and tap into cultural trends of past eras. AI systems can learn the color palettes and design aesthetics that were popular during a certain period and use them to generate images that evoke nostalgia. For example, an image aiming for a 1960s aesthetic might incorporate the muted, softer color tones that were common at the time, potentially drawing on a sense of nostalgia from consumers who lived through that era or are interested in its style.
This ability extends to recreating lighting conditions common in vintage photos. Tools can digitally reproduce the soft, diffused light often seen in 1960s photography, allowing for a more inviting, softer look in a product image. It is still early days for this technology, but one can imagine how it might be useful for businesses to produce product photos that have a certain vintage aesthetic, hoping it appeals to buyers who are attracted to a sense of history and nostalgia.
Beyond simply mimicking aesthetics, these AI tools are also starting to integrate elements of consumer behavior. They can analyze how consumers have interacted with different visuals in the past and use that to optimize images for modern e-commerce. This is where it gets interesting—trying to blend historical aesthetic principles with the ability to fine-tune the images in a way that is likely to resonate with a wider market. While this is helpful for e-commerce, it's also something that needs to be handled carefully, considering questions about originality and authenticity when these tools are used to manipulate visual information in a sales context.
One of the most promising things is the speed at which these systems can iterate. AI-driven systems can rapidly produce different versions of a product image, tweaking various aspects of the vintage style—color, texture, and even the context of the background. This speeds up the design process compared to the longer, more traditional methods of photography and helps companies experiment more with different aesthetics.
However, a major question remains regarding how to balance the attempt to recreate historical aesthetics with the realities of contemporary visual communication and e-commerce. There's a fine line between authenticity and commercialization, and these technologies raise intriguing questions about the relationship between consumers and the images that are used to entice them to buy a product. While we are at an early stage with these systems, it's clear that they are becoming increasingly powerful in their ability to blend past aesthetics with modern technology, and how these technologies are applied will be something to watch in the coming years.
AI-Enhanced Product Photography Recreating Vintage Matchbox Design Aesthetics for E-commerce - Computer Vision Tools That Apply Authentic Aging and Weathering Effects
Computer vision tools are increasingly adept at applying convincing aged and weathered effects to images, which is a boon for product photography, especially in ecommerce. These tools utilize techniques like texture analysis, employing small visual elements to mimic the natural wear and tear that occurs over time. This approach helps create images with a greater sense of realism, appealing to those who enjoy vintage and nostalgic aesthetics. Additionally, the use of Generative Adversarial Networks (GANs) has been instrumental in improving texture quality and reducing the artificial look of these effects. The result is AI-generated imagery that captures the essence of vintage designs more effectively.
However, as ecommerce leverages these tools, the challenge of maintaining a balance between authenticity and appealing to modern design sensibilities becomes apparent. While the ability to artificially age an image can boost a product's visual appeal, it also raises questions about the overall honesty of the presentation. This is particularly important for brands that want to create a connection with customers who appreciate a genuine connection to the past. In the end, the incorporation of computer vision into product photography is not just about making new kinds of images. It also questions how we use vintage aesthetics and visual storytelling in today's online environments.
Computer vision tools are increasingly being used to convincingly simulate the effects of aging and weathering on products shown in e-commerce images. They achieve this by analyzing the textures and wear patterns found in genuinely aged items, enabling them to recreate the look of scratches, fading, and other signs of time in a remarkably lifelike way. This capability is valuable for recreating the aesthetic of vintage designs, especially when aiming for a sense of nostalgia.
Sophisticated machine learning models can analyze large collections of old product images to pick out incredibly subtle details that hinted at a product's age. These details, such as discoloration and material degradation, can then be replicated to achieve more authentic-looking vintage styles. It's fascinating how these models can essentially learn the visual language of age.
Parametric modeling techniques allow for more precise control over the aging process. By adjusting things like texture intensity and how aggressively the aging effect is applied, based on examples from specific time periods, designers can create variants of the same product image, each reflecting a slightly different stage of "aging" or level of wear. This makes them incredibly useful for ecommerce, especially when trying to create a range of visuals within a product line.
There's growing evidence that consumers respond positively to products that appear aged. This may be due to a perception that these items have a greater emotional depth or authenticity. Mimicking wear can evoke memories or tap into subconscious associations that nudge buyers towards certain product types, especially those with nostalgic value. It's an interesting area of research in how simulated wear can impact purchasing choices.
These computer vision tools leverage texture mapping to give 2D images a sense of physical depth and realism. By effectively creating the illusion of layers and surface variations, they can help ecommerce products look more tactile and engaging. This tactic can play on the idea that we want to be able to 'feel' what we're buying even if it's online.
By integrating historical background elements into the photos, the computer vision tools can place products within a more engaging narrative context. Adding details from the original era can make the product feel more believable and relevant to those interested in vintage aesthetics. This tactic can work well for product lines trying to evoke a certain time period.
There's also the ability to fine-tune aging effects to match different preferences or target particular segments within a market. By manipulating imperfections, ecommerce brands can tailor the vintage feel to specific tastes, a clever way to address the variety of visual styles that fall under the broader category of "vintage."
Using historical data on design variations over time, AI can check that the simulated aging effects are genuine to the time period it's trying to emulate. This helps ensure the generated images are faithful to the aesthetic the ecommerce site is aiming for. It's a way to check for errors, like accidental anachronisms, in an AI-driven workflow.
Some of these tools are starting to include feedback loops that use real consumer interactions to influence the way aging effects are applied. This ability for the AI system to learn in real-time is impressive and could lead to ever more realistic and refined aging effects over time. It's the equivalent of a kind of design evolution guided by data.
Finally, these tools don't just simulate aging but can also recreate lighting conditions found in older photographs. The careful interplay between light and shadow is a critical component in how we perceive age and wear, making this a critical aspect of creating realistic-looking vintage effects. This further increases the authenticity of the product representation.
While the use of these computer vision techniques is still a developing field, it offers an intriguing avenue for presenting products online. It can potentially improve the connection between ecommerce images and the desire for nostalgia and vintage aesthetics. How this technology evolves over time and how it's used will be interesting to watch.
More Posts from lionvaplus.com: