7 Key Factors When Integrating Copyright-Free Images into AI Product Photography Workflows
7 Key Factors When Integrating Copyright-Free Images into AI Product Photography Workflows - Setting Up Digital Asset Management Systems For Large Scale Creative Commons Images
When dealing with a vast library of Creative Commons images for e-commerce, particularly within AI-powered product photography workflows, a robust digital asset management (DAM) system becomes indispensable. These systems serve as a central hub for storing, organizing, and retrieving all your imagery, streamlining collaboration amongst teams. Having a clear system in place ensures everyone can easily locate and use the necessary images for various projects, which is especially beneficial when dealing with a large volume of Creative Commons images.
The effectiveness of these DAM solutions depends on their capabilities to efficiently categorize and tag images with rich metadata. This allows for quick search and retrieval, seamlessly integrating copyright-free images into your workflow. Whether choosing proprietary or open-source DAM software, the focus should always be on creating a system that promotes efficient organization and effortless retrieval.
Beyond simple storage, a functional DAM also needs to address the intricacies of managing Creative Commons usage. Implementing secure access and sharing protocols is paramount, ensuring compliance with the licensing conditions of the images. These elements are critical for preventing issues related to copyright and maintaining the integrity of your image library. By building a system that integrates workflows and safeguards compliance, e-commerce businesses can successfully leverage the benefits of Creative Commons images while mitigating potential risks. Essentially, a well-designed DAM system is a foundational component for any e-commerce operation that utilizes AI and Creative Commons assets, leading to a smoother and more efficient image management process.
When dealing with a large volume of Creative Commons images for ecommerce product photography, especially when integrated with AI-generated imagery, a well-structured Digital Asset Management System (DAMS) is crucial. These systems provide a centralized repository for all your image assets, streamlining how they're accessed, organized, and used. For instance, tools like Canto or Cloudinary can help manage a large collection by automatically adding metadata, tags, and labels. This organization is vital when it comes to locating specific images quickly. Think of it like having a highly-organized library for your images instead of a chaotic pile of folders, drastically cutting down on the time spent searching for the right picture.
Open-source options like Pimcore and AtroDAM also exist, and provide flexibility for tailoring the system to your specific requirements. Furthermore, these tools can help integrate with other platforms you're using for product management or online sales. Setting up a DAMS involves defining protocols for secure sharing, creating user-friendly portals for browsing images, and configuring options for external uploads. Key features include image capture and storage, as well as repurposing elements from branded templates.
It's worth noting that these systems can also contribute to better collaboration and feedback on images. Think of a built-in commenting and approval system built into the system for your team. This simplifies the process of getting feedback and approvals before launching new product images.
The challenge with using Creative Commons images is ensuring compliance. Many users struggle with the different license types available and their limitations. It's easy to make a mistake and use an image in a way that wasn't intended. Properly managing these licenses and making sure they're understood is an important step in avoiding legal headaches down the line. You don't want to wind up in a legal dispute over image usage.
7 Key Factors When Integrating Copyright-Free Images into AI Product Photography Workflows - Midjourney Style Transfer Techniques Using Public Domain Reference Materials
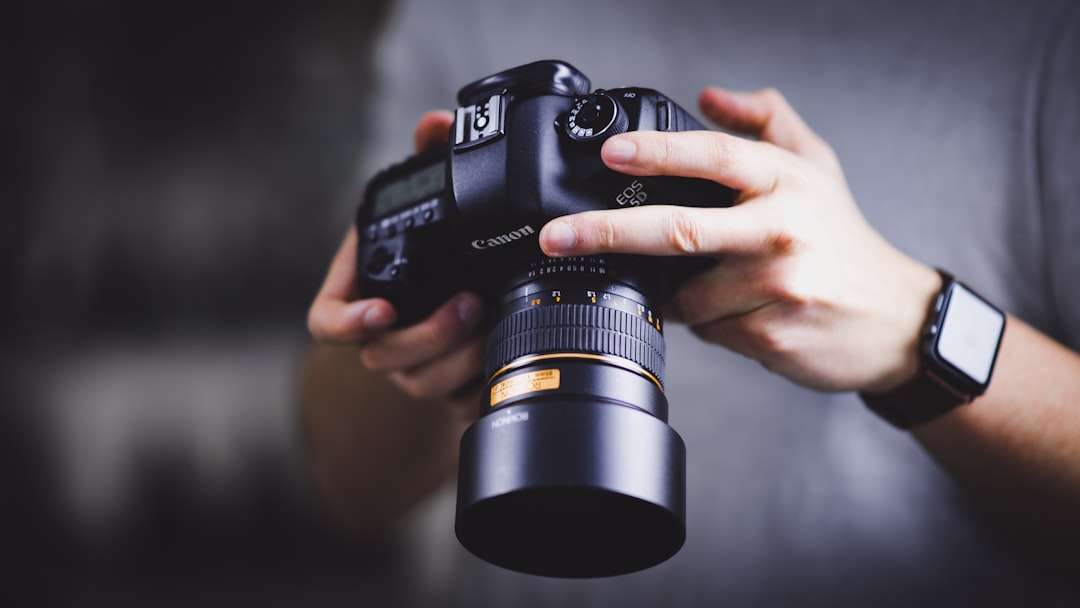
Midjourney's style transfer capabilities, specifically leveraging public domain reference materials, offer a compelling approach for refining the aesthetic of e-commerce product images generated through AI. By using the 'sref' feature, you can introduce specific stylistic elements into your images by referencing an image URL. This allows for the recreation of complex visual aesthetics that might be difficult to convey solely with text prompts. For example, one could apply the stylistic characteristics of early color photography pioneers to product imagery, creating a unique visual identity. It's important to remember that maintaining clarity is crucial when using style references. Keeping the chosen reference images simple and relevant to the desired style helps ensure that the final generated images achieve the intended visual effect. Furthermore, managing aspect ratios correctly when applying style references ensures consistency between the reference and the output image. The ability to use 'sref' to call upon a particular style, either alone or in conjunction with a URL for a specific image, is useful in creating visual continuity across a range of product imagery, which in turn can positively contribute to the presentation of a brand. This method shows promise for ecommerce product image creation by injecting character and consistency into product visualizations in a way that avoids the issues of copyright restrictions.
Midjourney's version 6 introduced a way to apply specific visual styles to generated images using a 'sref' parameter linked to an image URL. This 'style reference' capability is particularly handy for styles that are tough to describe with just text prompts. For example, we can take inspiration from Sergey Prokudin-Gorskii's pioneering color photography work and apply that style to our generated product images. The key here is to pay attention to image aspect ratios – matching the reference and output dimensions is important for predictable results.
Keeping it simple is often the best approach when choosing style references. Using too many, or overly complex references, can lead to unclear outcomes. However, it's also possible to mix and match style reference codes with image URLs, allowing for blending predefined styles with specific image examples. Platforms like Lummi specialize in curated style references for Midjourney, streamlining the process of achieving consistent imagery without constant manual adjustments.
Maintaining visual consistency across a range of product imagery is important. A guide focusing on consistent visual outputs emphasizes the importance of style references to ensure uniformity. Srefsco is one example of a resource offering a large selection of Midjourney style references, with over 50,000 available and searchable. Ultimately, using style references in a Midjourney prompt involves incorporating an 'sref' code along with the image URL. This allows for greater control over the final aesthetic output and promotes consistency in newly created images. It is worth noting that this approach can be a double-edged sword; while it can accelerate consistent stylistic outputs, it can also limit the model's ability to explore novel visual solutions. There may be instances where it might be better to generate a wider variety of results before focusing on stylistic consistency.
7 Key Factors When Integrating Copyright-Free Images into AI Product Photography Workflows - Training Product Photography AI With Wikimedia Commons Image Datasets
Using Wikimedia Commons images to train AI for product photography offers exciting possibilities but also presents challenges. The vast collection of freely available images can be used to improve e-commerce operations by creating visually appealing product photos and streamlining processes. However, there's ongoing discussion about the legal and ethical implications of using these images for AI model training. Questions around fair use and how AI's creative output impacts copyright remain unclear. Balancing the need to generate high-quality, brand-consistent images with the need to comply with copyright restrictions is a difficult task for businesses. While utilizing these public domain resources can spark creative solutions for product visuals, businesses must carefully manage potential legal risks when using them in AI training and generation. The key is to be mindful of the legal aspects while pursuing the potential for more efficient and creative visual outputs.
Training AI models for product photography using image datasets from Wikimedia Commons presents a fascinating avenue for research and development in e-commerce. One notable aspect is the abundance of metadata associated with these images. Metadata, which includes information like licensing terms and original creator details, is crucial for ensuring that any generated product imagery complies with copyright restrictions, a major concern for e-commerce businesses. This rich metadata can help us ensure that AI-generated images aren't accidentally used in a manner that violates licensing agreements.
Additionally, many images within Wikimedia are available in a range of formats and resolutions. This diversity in the training data can be beneficial for generating high-quality product images suitable for a wider array of e-commerce platforms, which often have different requirements for product photos. The sheer scale of the Wikimedia Commons project allows us to train AI models on millions of images, creating a more robust model that's exposed to a greater variety of product styles and presentations. This breadth of data, in theory, should lead to more realistic and higher-quality AI-generated product photos.
Another key advantage of Wikimedia is the presence of numerous images in the public domain or licensed under Creative Commons. This resource offers a sizable and readily accessible set of images without the legal complications that can arise with proprietary image resources. This can lead to a much smoother workflow, since you're not constantly worrying about potential copyright issues. And then there's the interesting element of art historical images found in Wikimedia Commons. Training AI models with this data can lead to some unique possibilities in e-commerce imagery. For example, we could explore generating images in the style of early photography, creating unique visuals that stand out.
Data augmentation techniques can further enhance AI model training by generating variations of images within the dataset. This can lead to models with improved generalizability and the ability to create a more diverse range of product images. The multilingual nature of Wikimedia tagging, with images often tagged in multiple languages, also opens up possibilities for image localization, potentially making it easier to adapt product visuals to different regions and cultures. It's interesting to think about how the historical context and descriptions often associated with Wikimedia images could contribute to AI's ability to not just generate product visuals, but also tell a narrative about them. This can be a valuable feature for enriching e-commerce storytelling, something we are seeing a growing demand for.
Furthermore, models trained on such a vast and diverse dataset might be better at understanding how products are visually represented and improve product image search and retrieval within e-commerce platforms. The diversity of artistic styles in Wikimedia can also encourage AI to explore a wider range of aesthetic variations when generating images. This could lead to innovative and engaging product displays on e-commerce sites, breaking away from the somewhat standardized visuals that are often seen in online shopping.
However, it's crucial to remember that the usefulness of this approach depends heavily on how well the Wikimedia Commons data is curated and organized. If the images are not accurately tagged or categorized, it could create challenges in training a truly robust AI model. Also, there are concerns about the potential for biases to be present within the dataset, which may lead to the generation of biased outputs from the AI models. These considerations are essential to keep in mind when exploring this avenue of AI training for product photography.
While these are promising avenues for enhancing product image creation through AI, careful attention to the data quality, bias detection, and the nuances of copyright and licensing is crucial to fully realize its potential.
7 Key Factors When Integrating Copyright-Free Images into AI Product Photography Workflows - Automated Metadata Tagging Of Stock Photo Libraries For Machine Learning
The automated tagging of images within stock photo libraries is fundamentally altering how e-commerce and AI-powered product photography manage their image assets. By employing machine learning algorithms, these systems can analyze images and automatically generate a set of descriptive keywords, significantly boosting the efficiency of organizing vast image collections. In some cases, automation has reportedly reduced manual tagging workloads by as much as 85%.
These tagging systems are commonly integrated into digital asset management (DAM) systems. This allows for improved image searchability, making it simpler to locate and use copyright-free images for product photography or other marketing initiatives. This is becoming increasingly important as businesses are more frequently needing to find and use image resources that adhere to specific license requirements. The overall goal is to make it easier to integrate and manage copyright-free images into e-commerce workflows.
The trend towards automated tagging is not just about simplifying processes; it's also about improving compliance with copyright stipulations and ensuring that usage aligns with licensing agreements. The ability to dynamically tag images, through future AI developments, has the potential to significantly reduce delays when integrating new imagery. As a result, AI tagging solutions can increase the overall effectiveness of managing images while improving the overall organizational capabilities of both photographers and the companies that use their work.
The use of automated metadata tagging in stock photo libraries is becoming increasingly important, especially for businesses using AI in their product photography workflows, such as those involved in e-commerce. AI-powered systems are designed to improve the efficiency and effectiveness of managing large image libraries by automatically generating relevant keywords and tags.
One of the most significant benefits of automated tagging is the considerable time savings it offers. It's been reported that AI tools can reduce the time spent manually labeling images by up to 85%, freeing up human resources to focus on other aspects of the workflow. These tools use sophisticated algorithms that analyze the content of images, identifying key objects, colors, and other relevant details to create a set of relevant tags. For example, some tools can automatically suggest up to 30 keywords based on the various elements within an image.
These systems are particularly helpful when integrated with Digital Asset Management (DAM) systems. By adding AI-generated tags, search capabilities are greatly enhanced, allowing users to quickly find the images they need based on a wider variety of search criteria. This is especially critical in e-commerce where a wide variety of images are needed for different purposes, from product displays to marketing materials.
The ability to dynamically tag images using generative AI is also a game-changer. It can greatly reduce the time it takes to get new images added to a library and ready for use, streamlining the integration process. Furthermore, AI tagging isn't just about improving efficiency; it can also boost a business's overall ability to manage its visual assets. It essentially helps to create smarter workflows, resulting in better image organization and retrieval.
This trend toward automation reflects the growing need for businesses to manage massive volumes of images effectively, particularly in light of copyright considerations. As businesses continue to integrate AI into their product photography processes, it's likely that automated tagging solutions will become even more critical for ensuring that image assets are properly managed and easily accessible. While there are clear benefits, there are potential challenges such as potential biases within datasets used to train the AI, that need to be acknowledged and carefully addressed. The potential for biases to influence image selection needs to be monitored, and algorithms refined to ensure a wider range of representations. Nonetheless, automated tagging is clearly becoming a crucial technology for anyone working with large image libraries for e-commerce and related AI applications.
7 Key Factors When Integrating Copyright-Free Images into AI Product Photography Workflows - Background Removal Workflows Using Non-Licensed Image Resources
When creating product images for e-commerce, using readily available, non-licensed images for background removal can significantly streamline workflows. AI-powered tools can quickly and effectively erase existing backgrounds, allowing for the creation of clean, professional-looking product shots. This automation speeds up the process of generating a large number of visuals for online catalogs, while simultaneously offering opportunities for customizing backgrounds to match a specific brand's aesthetic.
Although the ease and accuracy of AI background removal tools are beneficial, their integration into e-commerce workflows requires careful consideration. Businesses must navigate the complex landscape of copyright and image licensing to avoid any legal complications. It's also vital to maintain an awareness of the limitations of some AI tools, as they can sometimes compromise the quality of the image itself. Achieving the right balance between efficiency and image integrity is key to successfully using this technique for creating impactful product visualizations.
When working with a large volume of freely available images for e-commerce product photography, a key aspect of the workflow involves removing the background. This is especially important when integrating AI-generated elements or creating unique product visualizations. Many readily available tools can handle background removal, leveraging AI-powered techniques to automate the process.
While some services offer robust APIs like Removebg, which can automate background removal across a large number of images, others focus on specific aspects of the task. For example, MODNetBGRemover is specifically built for automated background removal, showcasing the niche focus of some of these technologies. The command-line tool, BackgroundRemover, provides an open-source alternative for those who prefer greater control or are looking for a free option.
Cloud-based services such as Cloudinary allow integration into existing workflows through add-ons. This means they can automatically process image uploads and replace original images with a transparent PNG after analyzing and removing the background. This type of functionality streamlines the entire workflow, but we also have to consider that it often requires image upload to a third-party service, which might raise concerns for some users about data privacy and security.
Other projects, such as the djangobgRemoverML project, tie background removal functionality into a larger software project (Django), providing a specific solution within a particular environment. It's interesting to see how background removal becomes part of a larger software framework. The use of AI for background removal is also available in more user-friendly formats. For instance, InPixio and Erasebg are free tools that simplify the image editing process for users without extensive photo editing experience. These types of applications potentially make image enhancement tools accessible to a wider audience of ecommerce sellers and managers.
While the use of these tools can simplify the process of generating product visuals for e-commerce, we have to consider the quality of the results and how effective these tools are in complex situations, such as images that feature complex textures or unusual lighting conditions. It's important to understand that in such scenarios, manual image editing or more specialized tools might still be necessary to create truly high-quality product images. It also is interesting to reflect on how background removal and related technologies might influence creative decision-making in e-commerce. Are these tools enabling new forms of product photography? Or are they simply creating new ways to achieve the same outcomes as traditional image editing? These are interesting questions to consider as we see an increasing reliance on these types of tools in the e-commerce space.
7 Key Factors When Integrating Copyright-Free Images into AI Product Photography Workflows - Legal Documentation Requirements When Using Open Source Images For Commercial AI
When employing open-source images within commercial AI projects, like generating product visuals for an e-commerce store, understanding the related legal documents is vital. A careful review of the licenses is crucial to ensure they permit commercial use, as some licenses, despite claiming to be open-source, may contain restrictions against it. Furthermore, the legal landscape around AI-generated works is still forming, especially concerning authorship and originality. Copyright considerations for these AI-created images can be tricky because of different laws around the world, and keeping track of usage rights is important to avoid problems. It's a good idea for businesses to keep an eye on the ever-changing legal environment surrounding AI and copyright so they can use these resources responsibly while maximizing their creative potential. Essentially, careful documentation is key for staying on the right side of the law when using these images in your business.
When using open-source images to train AI for commercial tasks like generating product visuals, it's crucial to consider the legal landscape, which is still under development. While the idea of free-to-use images seems appealing, the reality is often more complex. For example, even if you're using images under a "public domain" license like Creative Commons Zero (CC0), you still need to be mindful that if your AI model learns specific styles from these images, it could potentially reproduce them without clear attribution, leading to potential ownership issues.
Different open-source licenses have different requirements. Some, like CC0, offer a lot of freedom, while others, like CC BY, require giving proper credit to the original creator, which can be a challenge in automated image generation. And then there's the legal concept of "fair use," which isn't always clear when it comes to AI-generated content. It's generally understood that fair use doesn't extend to commercial activities, so businesses need to be cautious about how they use open-source images in their product photography workflows. We're seeing more legal cases emerge that focus on AI-generated content and potential copyright violations, which makes it essential for businesses to stay informed about evolving laws related to ownership and reproduction.
The data within open-source images, especially the metadata, plays a significant role in legal compliance. Metadata can provide insights into licensing restrictions and usage rights, offering a valuable resource for understanding how to properly integrate these images into AI training. In addition to copyright, companies should consider "trade dress," which protects the overall look and feel of their products. Using open-source images might create issues if the way a product is visualized looks too similar to another brand's established imagery, which can create legal difficulties.
Understanding the specific attribution requirements of the images you're using is also critical. Incorrect attribution, even for images with open licenses, can lead to legal disputes. Additionally, AI models trained on open-source datasets can sometimes perpetuate biases that are present in those datasets. This could lead to products being presented in ways that aren't in line with a company's brand values or consumer expectations. The source of the open-source image also matters. Images from lesser-known or unregulated sources might have less-defined legal protections compared to those from established platforms, which could make copyright issues more likely.
Furthermore, with the development of automated tagging technologies, businesses are increasingly relying on dynamic tags to manage image libraries. However, this reliance comes with the responsibility of ensuring that the tags adhere to the licensing agreements for the images. If AI tagging tools mislabel or misuse images due to inaccurate tagging, it can expose companies to substantial legal risks. It highlights the continuous need for careful oversight of image management systems as they integrate AI components. It seems clear that navigating the legal landscape of open-source images for AI is becoming increasingly important for businesses, and attention to detail is key to avoid potential issues.
7 Key Factors When Integrating Copyright-Free Images into AI Product Photography Workflows - Quality Control Methods For AI Generated Product Photos Using Public Images
When using publicly available images to generate product photos with AI, ensuring high quality and adherence to licensing is essential for e-commerce. As AI tools are integrated into product image creation, it's crucial to establish reliable methods for controlling the quality of the generated visuals. This involves evaluating the images carefully, checking if they align with brand standards, and making sure they're not distorted or otherwise flawed during the AI process.
Leveraging the rich metadata often available with public images helps ensure that the AI output respects licensing and copyright restrictions. By using this information, it's possible to avoid legal issues or the accidental misuse of images. Furthermore, incorporating user feedback is beneficial for continuously improving the performance and aesthetic output of the AI models. As consumers interact with the images, their insights can highlight specific issues and pave the way for fine-tuning the AI models to produce visually appealing and commercially viable results.
But, there's a catch. AI algorithms are trained on large datasets, and those datasets can carry biases that might unintentionally surface in the product photos. Being mindful of this is important, as biased visuals could negatively impact the brand's image or alienate specific segments of the consumer base. Additionally, legal landscapes are evolving with AI-generated works, and it's important to be aware of how image usage rights apply in these new contexts. It's a dynamic environment that requires ongoing monitoring and adapting to stay legally compliant. By combining careful evaluations, consistent monitoring, and user insights, companies can harness the efficiency of AI while safeguarding the integrity and visual quality of their product images.
When using publicly available images to train AI models for generating product photos, ensuring the quality of the output becomes a crucial concern. One approach involves using algorithms designed to mimic human perception of image quality. These tools can analyze things like sharpness, color accuracy, and overall visual appeal, helping to ensure that the AI-generated photos meet specific aesthetic standards. This process can be inspired by how our visual systems work, aiming to produce images that are not only technically sound, but also visually appealing to consumers and potentially more likely to encourage sales.
Another quality control method involves comparing AI-generated product images to real, original photos. Techniques like structural similarity index (SSIM) and peak signal-to-noise ratio (PSNR) can be used to find even subtle differences between the two, highlighting discrepancies that might not be obvious to the human eye. These differences might, however, influence how consumers perceive the product.
The variety of the public domain images used to train AI systems also plays a crucial role in the quality of the outputs. For example, if the training dataset consists of images with a wide range of lighting conditions, product angles, and backgrounds, the AI model is likely to be better equipped to handle those same variations when generating new images. This creates more versatility and a potentially more realistic output.
Furthermore, techniques like semantic segmentation can be applied for more advanced quality control. This approach breaks down an image into its different parts (like the product itself, background, and any surrounding elements), ensuring that the AI renders those different parts correctly and consistently.
However, there are inherent challenges related to the quality of public domain images. Images that might be scanned poorly or heavily compressed could potentially introduce artifacts or imperfections into the generated photos. This highlights the importance of having careful selection processes for the images that are used in the training data.
Automated error detection tools can also help identify issues that might affect the quality of generated images. Machine learning models can be built to identify inconsistent dimensions, strange proportions, or unexpected elements in the context of the image, potentially flagging these errors before they ever make it to the e-commerce website.
Ideally, a balanced approach that incorporates both technical evaluation and human feedback would produce the best results. We can use algorithms to generate quantitative metrics, but that shouldn't be the sole evaluation criterion. Having expert human reviewers judge the aesthetic qualities alongside the algorithmic assessments helps bridge the gap between what the model thinks is good and what the target consumers would find appealing.
Information from the metadata of the source images, such as resolution, color profiles, and file format, can also guide the AI model towards using higher-quality images for generating new ones. This data can play a role in filtering out images that might lead to substandard AI outputs, resulting in an overall improvement in image quality.
Finally, incorporating mechanisms that allow for ongoing adjustments based on user feedback can further improve the AI model's ability to generate high-quality product images over time. These feedback loops provide a way to refine the system based on actual user interaction with the AI-generated photos. This type of continual refinement would ideally allow the system to adapt to changing trends and consumer expectations.
The future of product photography is intertwined with AI, but managing quality in this evolving landscape presents a constant challenge. As AI becomes more integral to ecommerce, the methods for controlling quality and generating consistent, high-quality product images will become increasingly sophisticated, ensuring an attractive and engaging shopping experience for consumers.
More Posts from lionvaplus.com: